Non-parametric statistics
Introduction
Non-parametric statistics, also known as distribution-free statistics, is a branch of statistics that does not require the data to follow a specified distribution. This field of statistics is often used when the data does not meet the assumptions of parametric statistics, or when no specific distribution can be assumed Statistics.

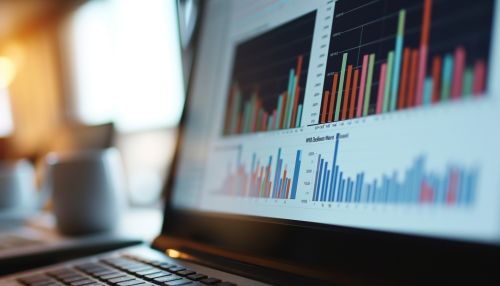
Overview
Non-parametric statistics make fewer assumptions about the data compared to parametric statistics. They do not require the data to be normally distributed and are often used when the data is ordinal or nominal. Non-parametric statistics are more robust to outliers and can be used with smaller sample sizes. However, they may not be as powerful as parametric tests when their assumptions are met.
Non-parametric Tests
There are several non-parametric tests used in statistical analysis. These include the Mann-Whitney U test, the Wilcoxon signed-rank test, the Kruskal-Wallis test, and the Spearman's rank correlation coefficient.
Mann-Whitney U Test
The Mann-Whitney U test, also known as the Wilcoxon rank-sum test, is used to compare two independent samples. It tests the null hypothesis that the distributions of the two samples are equal Mann-Whitney U Test.
Wilcoxon Signed-Rank Test
The Wilcoxon signed-rank test is used to compare two paired samples. It tests the null hypothesis that the median difference between pairs of observations is zero Wilcoxon Signed-Rank Test.
Kruskal-Wallis Test
The Kruskal-Wallis test is a non-parametric alternative to the one-way ANOVA. It is used to test the null hypothesis that the medians of the groups are equal Kruskal-Wallis Test.
Spearman's Rank Correlation Coefficient
Spearman's rank correlation coefficient is a non-parametric measure of the strength and direction of association between two ranked variables Spearman's Rank Correlation Coefficient.
Advantages and Disadvantages of Non-parametric Statistics
Non-parametric statistics have several advantages over parametric statistics. They are more robust to outliers and do not require the data to be normally distributed. They can be used with smaller sample sizes and with ordinal or nominal data.
However, non-parametric statistics also have some disadvantages. They may not be as powerful as parametric tests when their assumptions are met. They also require more data to achieve the same level of power as a parametric test.
Conclusion
Non-parametric statistics provide a valuable tool for researchers when the assumptions of parametric statistics cannot be met. They offer a robust and flexible approach to data analysis that can be used with a wide range of data types.