Sampling Distribution
Introduction
A Sampling Distribution is a probability distribution of a statistic obtained through a large number of samples drawn from a specific population. The sampling distribution of a given population is the distribution of frequencies of a range of different outcomes that could possibly occur for a statistic of a population.
Understanding Sampling Distribution
The concept of a sampling distribution is fundamental to the field of statistics and is often used in the social sciences, natural sciences, and other research fields. It is a method used to describe the distribution of sample statistics. The sample statistics are derived from repeated sampling from the same population.
Importance of Sampling Distribution
The importance of sampling distribution lies in its ability to quantify the statistical accuracy and variability of sample estimates. By understanding the concept of sampling distribution, one can infer about the population parameters from a set of sample data.
Types of Sampling Distribution
There are several types of sampling distributions, including the distribution of sample means, the distribution of sample proportions, the distribution of sample variances, and others. Each type of sampling distribution has its own unique properties and uses.
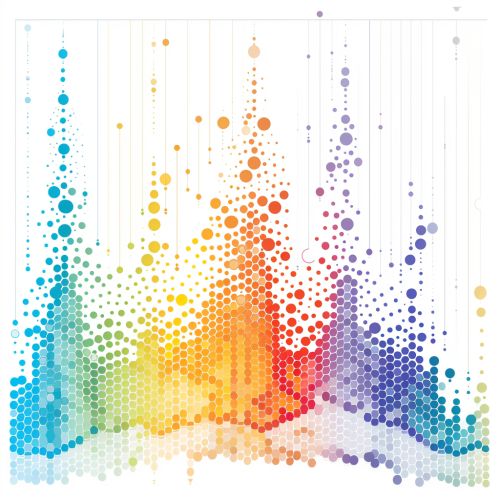

Distribution of Sample Means
The distribution of sample means, also known as the sampling distribution of the mean, is the probability distribution of the means of all possible samples of a given size that can be drawn from a population. The mean of the distribution of sample means is equal to the mean of the population, and its standard deviation is the standard error of the mean.
Distribution of Sample Proportions
The distribution of sample proportions, also known as the sampling distribution of the proportion, is the probability distribution of the proportions of all possible samples of a particular size that can be drawn from a population. The mean of the distribution of sample proportions is equal to the proportion of the population, and its standard deviation is the standard error of the proportion.
Distribution of Sample Variances
The distribution of sample variances, also known as the sampling distribution of the variance, is the probability distribution of the variances of all possible samples of a certain size that can be drawn from a population. The mean of the distribution of sample variances is equal to the variance of the population, and its standard deviation is the standard error of the variance.
Central Limit Theorem
The Central Limit Theorem is a key theorem in statistics that states that the distribution of sample means approximates a normal distribution as the sample size becomes larger, regardless of the shape of the population distribution. This theorem is fundamental to the reliability of many statistical procedures and concepts, including confidence intervals and hypothesis testing.
Law of Large Numbers
The Law of Large Numbers is another important concept related to sampling distributions. It states that as the size of a sample increases, the mean of all samples from the same population will approach the mean of the population. This law underpins many statistical concepts and validates the approach of reaching inferences about a population based on sample data.
Conclusion
In conclusion, a sampling distribution is a probability distribution of a statistic obtained from a large number of samples drawn from a specific population. It is a fundamental concept in statistics that enables us to make inferences about a population based on sample data.