Real-time Analytics
Introduction
Real-time analytics is a concept that refers to the process of extracting, analyzing, visualizing and acting upon data as soon as it enters a database or a system. This process is achieved through the use of advanced technologies and methodologies that allow for immediate insights and actions. Real-time analytics is a critical component of big data and business intelligence (BI) strategies, enabling organizations to make informed decisions promptly and accurately.
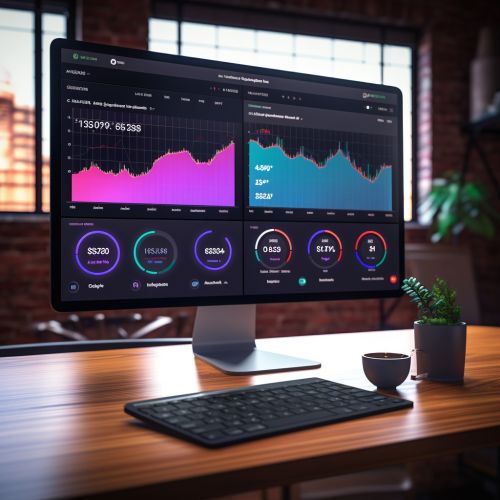
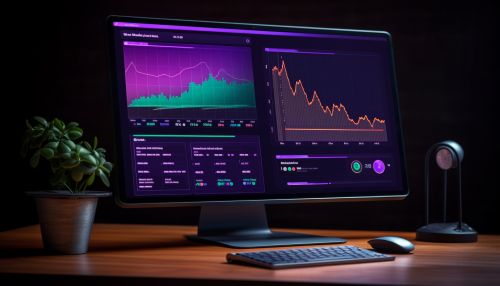
Overview
Real-time analytics involves the use of real-time data processing tools and techniques to analyze data as soon as it is produced or received. This allows for immediate insights and actions, which can be critical in various fields such as finance, healthcare, manufacturing, and more. The primary goal of real-time analytics is to provide the ability to act upon data immediately, without the need for batch processing or storing data for later analysis.
Importance of Real-Time Analytics
The importance of real-time analytics can be attributed to its ability to provide immediate insights and actions. This can significantly improve decision-making processes, operational efficiency, and overall business performance. For instance, in the financial sector, real-time analytics can be used to detect fraudulent transactions as they occur, allowing for immediate action to be taken. Similarly, in the healthcare sector, real-time analytics can be used to monitor patient health in real-time, enabling immediate medical intervention when necessary.
Real-Time Analytics Technologies
Various technologies enable real-time analytics, including stream processing, in-memory computing, and real-time databases. Stream processing involves the use of specialized software to process and analyze data streams in real-time. In-memory computing, on the other hand, involves storing data in the main memory of computing systems to allow for faster data processing and analysis. Real-time databases are databases designed to handle real-time data processing and analysis.
Real-Time Analytics Applications
Real-time analytics can be applied in various fields and industries. Some of the most common applications of real-time analytics include:
- Fraud detection: Real-time analytics can be used to analyze transaction data in real-time to detect fraudulent activities as they occur.
- Health monitoring: In the healthcare sector, real-time analytics can be used to monitor patient health in real-time, enabling immediate medical intervention when necessary.
- Supply chain management: Real-time analytics can be used to monitor and manage supply chain operations in real-time, allowing for immediate adjustments to be made when necessary.
- Social media monitoring: Real-time analytics can be used to monitor social media platforms in real-time, enabling immediate responses to customer queries, complaints, or feedback.
Challenges in Real-Time Analytics
Despite its numerous benefits, implementing real-time analytics can pose several challenges. These include data quality issues, data integration challenges, and the need for advanced analytical skills. Additionally, real-time analytics requires significant computational resources, which can be costly.
Conclusion
Real-time analytics is a critical component of modern data-driven strategies, providing immediate insights and actions that can significantly improve decision-making processes, operational efficiency, and overall business performance. Despite the challenges associated with its implementation, the benefits of real-time analytics often outweigh the costs, making it a worthwhile investment for many organizations.