Prescriptive Analytics
Introduction
Prescriptive analytics is a field of business analytics that focuses on finding the best course of action for a given situation. It is related to both descriptive and predictive analytics but goes a step further by recommending actions based on the results of the previous two.
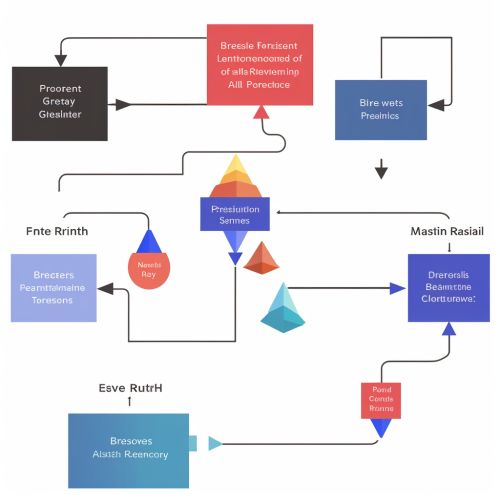
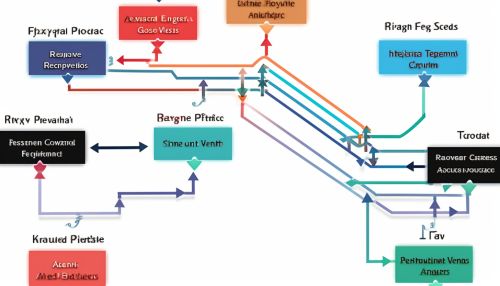
Overview
Prescriptive analytics is the third and final phase of business analytics, which also includes descriptive and predictive analytics. While descriptive analytics aims to provide insight into what has happened and predictive analytics helps model and forecast what might happen, prescriptive analytics seeks to determine the best solution or outcome among various choices, given the known parameters.
Prescriptive analytics can be thought of as the final step in an organization's decision-making process, allowing it to take informed action based on data and analytical insights. It uses a combination of techniques and tools such as business rules, algorithms, machine learning and computational modelling procedures. These techniques are applied against input from many different data sets including historical and transactional data, real-time data feeds, and big data.
Applications
Prescriptive analytics is used in many industries to optimize production schedules, inventory management, and supply chain design. It is also used in the oil and gas industry to optimize production levels and in the airline industry to set ticket prices. Other industries that use prescriptive analytics include healthcare, insurance, telecommunications, and retail.
In healthcare, prescriptive analytics can be used to improve patient outcomes by analyzing various treatment options and predicting how each option would affect the patient's health status. In insurance, it can be used to optimize pricing and risk assessment. In telecommunications, it can be used to optimize network infrastructure. And in retail, it can be used to optimize pricing, promotions, and inventory levels.
Techniques
Prescriptive analytics uses several techniques such as machine learning, artificial intelligence, and mathematical sciences. Some common techniques include decision tree analysis, Monte Carlo simulation, optimization, stochastic optimization, and graph analysis.
Decision tree analysis is a graphical representation of possible solutions to a decision based on certain conditions. It's often used in operations research, specifically in decision analysis, to help identify a strategy that most likely leads to a goal.
Monte Carlo simulation is a computational algorithm that relies on repeated random sampling to obtain numerical results. It's often used in physical and mathematical problems and is useful when it's impractical or impossible to use other approaches.
Optimization is the process of making a system or design as effective or functional as possible. Mathematical optimization techniques are used to determine the best solution for a problem in terms of a specified set of criteria.
Stochastic optimization is a method that incorporates randomness into the optimization process. It's often used when the best solution may change over time due to uncertainty.
Graph analysis is a method used in mathematical sociology to measure pairwise relationships between nodes. It's often used in network theory, a part of graph theory, and has applications in fields such as computer science and neuroscience.
Challenges
While prescriptive analytics can be incredibly powerful, it's not without its challenges. Some of these include data quality, the need for skilled analysts, and the requirement for powerful computing resources.
Data quality is a significant challenge because the results of prescriptive analytics are only as good as the data that's used. If the data is incomplete or inaccurate, the results will be too.
The need for skilled analysts is another challenge. Prescriptive analytics is a complex field that requires a deep understanding of data analysis, business strategy, and operations research. Not every organization has these skills in-house.
Finally, prescriptive analytics requires powerful computing resources. The algorithms used in prescriptive analytics can be computationally intensive, especially when they're applied to big data. This means that organizations need to invest in high-performance computing systems to support prescriptive analytics.
Conclusion
Prescriptive analytics represents the future of business analytics. By using advanced techniques like machine learning and artificial intelligence, organizations can gain insights into their data that were previously unimaginable. These insights can then be used to make informed decisions, optimize operations, and improve overall business performance.