Parametric statistics
Introduction
Parametric statistics is a branch of statistics that assumes data has come from a type of probability distribution and makes inferences about the parameters of the distribution. The term "parametric" comes from the fact that these types of statistics depend on the parameters of the data's distribution. This is in contrast to non-parametric statistics, which do not rely on the data fitting any particular distribution.
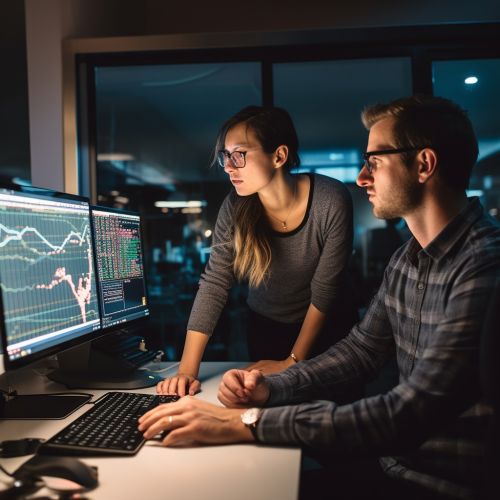
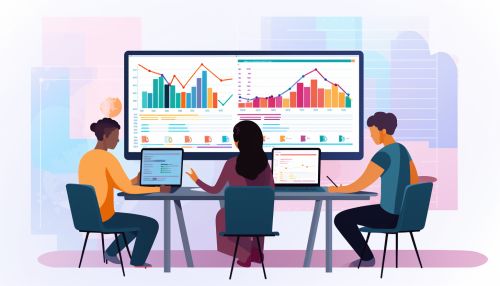
Assumptions of Parametric Statistics
Parametric statistics are based on several key assumptions. These assumptions, if met, allow for the application of a range of powerful statistical tests and models.
Independence
The first assumption is that of independence. This means that each observation in the dataset is independent of all other observations. In other words, the occurrence of one event does not influence the occurrence of another. This assumption is crucial for many statistical tests, such as the t-test and ANOVA.
Normality
The second assumption is that of normality. This means that the data follows a normal distribution, also known as a Gaussian distribution. This bell-shaped distribution is characterized by its mean and standard deviation, and it is a key assumption for many parametric tests.
Homogeneity of Variances
The third assumption is that of homogeneity of variances, also known as homoscedasticity. This means that the variances, or spread of data, are equal across all levels of the independent variables. This assumption is important for tests such as ANOVA, where the comparison of variances is a key part of the analysis.
Parametric Tests
There are many statistical tests that fall under the umbrella of parametric statistics. These tests are powerful tools for making inferences about population parameters based on sample data.
T-Test
The t-test is a parametric test used to determine whether there is a significant difference between the means of two groups. The test assumes that the data follows a normal distribution and that variances are equal between groups.
ANOVA
Analysis of variance, or ANOVA, is a parametric test used to compare the means of three or more groups. Like the t-test, ANOVA assumes that the data follows a normal distribution and that variances are equal between groups.
Regression Analysis
Regression analysis is a set of statistical processes for estimating the relationships among variables. It includes many techniques for modeling and analyzing several variables, when the focus is on the relationship between a dependent variable and one or more independent variables.
Advantages and Disadvantages of Parametric Statistics
Parametric statistics, while powerful, come with their own set of advantages and disadvantages.
Advantages
Parametric tests are more powerful than non-parametric tests, meaning they have a greater ability to detect a statistically significant effect, if one exists. This is because they make full use of the information in the data, including the magnitude and direction of differences, as well as the scores themselves.
Disadvantages
The main disadvantage of parametric statistics is that they require the data to meet certain assumptions. If these assumptions are not met, the results of the statistical tests may not be valid. In these cases, non-parametric statistics may be a more appropriate choice.
Conclusion
Parametric statistics are a powerful tool in the field of statistics, allowing for robust inferences about population parameters. However, they require certain assumptions to be met, and when these assumptions are not met, non-parametric statistics may be a more appropriate choice.