Ordinal data
Introduction
Ordinal data is a type of categorical data where the categories have a meaningful order or ranking, but the intervals between the categories are not necessarily equal. This type of data is commonly used in various fields such as statistics, psychometrics, and social sciences to measure variables that have a natural order but do not have a precise numerical value. Examples of ordinal data include Likert scale responses, class rankings, and levels of education.
Characteristics of Ordinal Data
Ordinal data possesses several key characteristics that distinguish it from other types of data:
- **Order:** The primary characteristic of ordinal data is that it has a clear, meaningful order. For example, in a survey measuring customer satisfaction, responses might be categorized as "very dissatisfied," "dissatisfied," "neutral," "satisfied," and "very satisfied."
- **Non-Equal Intervals:** Unlike interval data or ratio data, the intervals between the categories in ordinal data are not necessarily equal. For instance, the difference in satisfaction between "neutral" and "satisfied" may not be the same as the difference between "satisfied" and "very satisfied."
- **Categorical Nature:** Ordinal data is categorical, meaning it consists of distinct categories or groups. However, unlike nominal data, these categories have a specific order.
Collection and Representation
Ordinal data can be collected through various methods, including surveys, questionnaires, and observational studies. It is often represented using numbers or labels that indicate the order of the categories. For example, a Likert scale might use numbers from 1 to 5 to represent levels of agreement, with 1 indicating "strongly disagree" and 5 indicating "strongly agree."
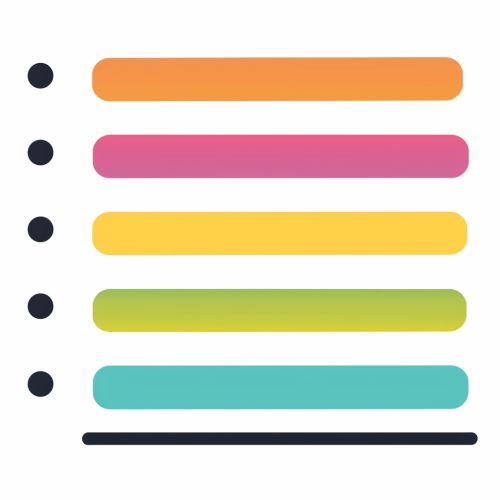
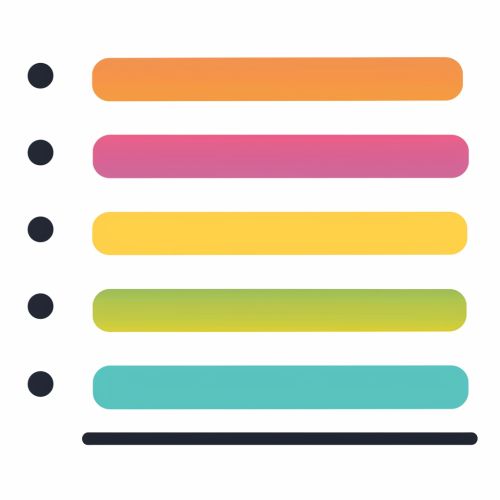
Analysis of Ordinal Data
Analyzing ordinal data requires specialized statistical techniques that account for the ordered nature of the data. Some common methods include:
- **Descriptive Statistics:** Measures such as the median and mode are often used to summarize ordinal data. The median is particularly useful because it represents the middle value in an ordered dataset.
- **Non-Parametric Tests:** Since ordinal data does not assume equal intervals, non-parametric tests like the Mann-Whitney U test, Kruskal-Wallis test, and Wilcoxon signed-rank test are commonly used. These tests do not rely on the assumption of normality and are suitable for ordinal data.
- **Ordinal Regression:** Ordinal regression models, such as the proportional odds model, are used to analyze the relationship between an ordinal dependent variable and one or more independent variables. These models account for the ordered nature of the data and provide insights into the factors influencing the ordinal outcome.
Applications of Ordinal Data
Ordinal data is widely used in various fields due to its ability to capture ordered information. Some notable applications include:
- **Psychometrics:** In the field of psychometrics, ordinal data is used to measure psychological traits, attitudes, and behaviors. Instruments like the Likert scale and Guttman scale are commonly employed to collect ordinal data in psychological assessments.
- **Education:** Ordinal data is used to rank students, courses, and educational institutions. For example, class rankings and grade levels are ordinal measures that provide meaningful information about students' academic performance.
- **Market Research:** In market research, ordinal data is used to gauge customer preferences, satisfaction, and perceptions. Surveys often include ordinal questions to understand consumer attitudes and behaviors.
Limitations of Ordinal Data
While ordinal data is useful for capturing ordered information, it has certain limitations:
- **Lack of Equal Intervals:** The non-equal intervals between categories can make it challenging to perform certain statistical analyses. For example, calculating the mean of ordinal data is not meaningful because the intervals between categories are not consistent.
- **Limited Precision:** Ordinal data provides less precise information compared to interval or ratio data. It indicates the order of categories but does not quantify the exact differences between them.
- **Subjectivity:** The interpretation of ordinal data can be subjective, especially when the categories are based on respondents' perceptions or opinions. This subjectivity can introduce bias and variability in the data.