Statistical Hypothesis Testing
Introduction
Statistical hypothesis testing, often referred to as confirmatory data analysis, is a method used in statistics to test the validity of a claim or hypothesis about a population parameter. The method uses data from a sample to make inferences about the population from which the sample was drawn.
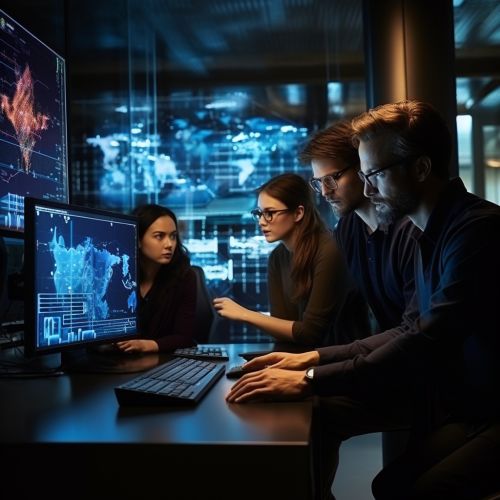

Hypotheses in Statistical Testing
In statistical hypothesis testing, two hypotheses are made: the null hypothesis (denoted by H0) and the alternative hypothesis (denoted by H1 or Ha). The null hypothesis is a statement of no effect or no difference and is assumed to be true until evidence suggests otherwise. The alternative hypothesis is a statement that directly contradicts the null hypothesis.
Steps in Hypothesis Testing
The process of hypothesis testing involves several steps:
- Formulate the null and alternative hypotheses.
- Choose the level of significance (α). This is the probability of rejecting the null hypothesis when it is true.
- Select the appropriate test statistic based on the nature of the data and the sample size.
- Formulate the decision rule based on the type of test statistic.
- Calculate the test statistic.
- Make a decision to reject or not to reject the null hypothesis.
Types of Errors in Hypothesis Testing
In hypothesis testing, there are two types of errors: Type I and Type II. A Type I error occurs when the null hypothesis is true, but is rejected. A Type II error occurs when the null hypothesis is false, but is not rejected.
Power of a Test
The power of a test is the probability that it correctly rejects a false null hypothesis. The power is affected by several factors including the significance level, the sample size, and the effect size.
Parametric and Non-Parametric Tests
Statistical tests can be classified into parametric and non-parametric tests. Parametric tests assume that the data follow a certain distribution, while non-parametric tests do not make such assumptions.
Conclusion
Statistical hypothesis testing is a crucial tool in statistics that allows researchers to make inferences about population parameters based on sample data. Despite its widespread use, it is not without its limitations and criticisms, particularly concerning its reliance on p-values.