Measurement Uncertainty
Introduction
Measurement uncertainty is a quantifiable parameter associated with the result of a measurement, which defines the dispersion of the values that could reasonably be attributed to the measured quantity. It is a critical component in the field of metrology, the science of measurement. The concept of measurement uncertainty is central to ensuring the reliability, accuracy, and consistency of measurements in various scientific, industrial, and societal applications.
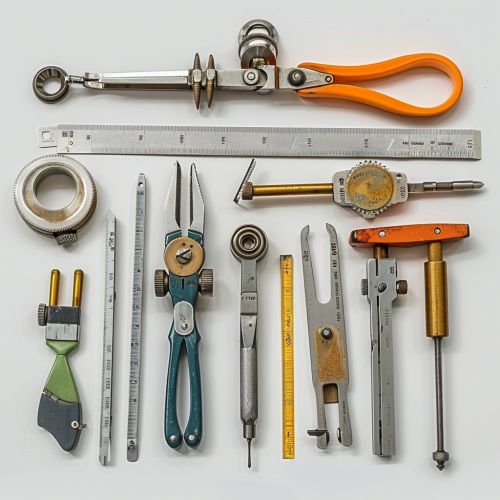
Understanding Measurement Uncertainty
Measurement uncertainty can be understood as a numerical value that characterizes the range within which the true value of a measurement lies. It is not a measure of the accuracy of the measurement, but rather a quantification of the doubt about the measurement result. This doubt arises due to the inherent limitations of the measurement process, including the effects of environmental conditions, the characteristics of the measurement instrument, and the interaction between the operator and the instrument.
Sources of Measurement Uncertainty
Measurement uncertainty can arise from various sources, which can be broadly classified into two categories: random and systematic uncertainties.
Random Uncertainties
Random uncertainties are those that vary in an unpredictable manner when a series of measurements of the same quantity are made under the same conditions. They are caused by inherently unpredictable fluctuations in the readings of a measurement apparatus or in the experimenter's interpretation of the instrumental reading. These uncertainties can be reduced by increasing the number of observations or measurements.
Systematic Uncertainties
Systematic uncertainties, on the other hand, are those that tend to shift all measurements in a systematic way. Causes of systematic uncertainties include imperfect calibration of measurement instruments, changes in the environment that interfere with the measurement process, and personal bias in reading the instruments. Unlike random uncertainties, systematic uncertainties cannot be reduced by increasing the number of measurements.
Evaluation of Measurement Uncertainty
The evaluation of measurement uncertainty is a critical step in the measurement process. It involves identifying and quantifying the sources of uncertainty, and combining them to give a total uncertainty for the measurement. There are two main approaches to the evaluation of measurement uncertainty: the 'GUM' approach and the Monte Carlo method.
'GUM' Approach
The 'GUM' approach, named after the Guide to the Expression of Uncertainty in Measurement (GUM), is a widely used method for evaluating measurement uncertainty. It involves identifying and quantifying the individual sources of uncertainty, and then combining them using the law of propagation of uncertainty. This approach is typically used when the relationship between the measurand and the input quantities is well defined and can be approximated by a linear function.
Monte Carlo Method
The Monte Carlo method is a statistical technique that involves generating a large number of random samples from the probability distributions of the input quantities, and then calculating the corresponding values of the measurand. The distribution of these values provides an estimate of the uncertainty of the measurement. This method is particularly useful when the relationship between the measurand and the input quantities is non-linear or complex.
Importance of Measurement Uncertainty
Understanding and quantifying measurement uncertainty is crucial in many fields, including engineering, physics, chemistry, and medicine. It allows scientists and engineers to assess the reliability of their measurements, to compare different measurement methods and systems, and to ensure the quality of products and services. In addition, the evaluation of measurement uncertainty is often required by international standards and regulations.