Mathematical Statistics
Introduction
Mathematical statistics is a branch of applied mathematics that uses probability theory and mathematical analysis to interpret statistical experiments. It involves the collection, analysis, interpretation, presentation, and organization of data. Mathematical statistics is a key foundation of data science, machine learning, and artificial intelligence, and it has applications in numerous fields, including economics, engineering, and the natural and social sciences.

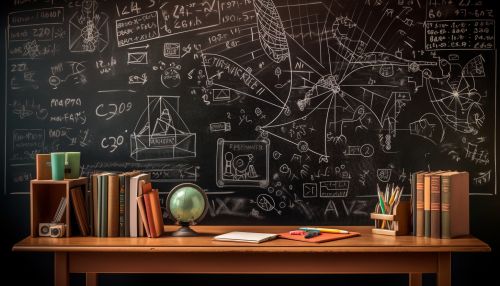
History
The history of mathematical statistics can be traced back to the 17th century with the development of probability theory by mathematicians such as Pascal and Fermat. In the 18th century, Gauss and Laplace made significant contributions to the field, including the development of the method of least squares and the central limit theorem.


Probability Theory
Probability theory is a fundamental component of mathematical statistics. It provides a mathematical framework for quantifying uncertainty and making predictions based on incomplete information. Key concepts in probability theory include random variables, probability distributions, and expectation values.

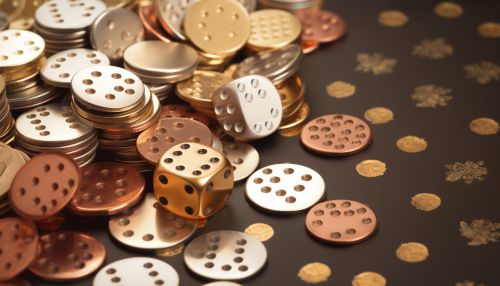
Random Variables
A random variable is a variable whose possible values are outcomes of a random phenomenon. There are two types of random variables: discrete and continuous. Discrete random variables have a countable number of possible values, while continuous random variables can take on any value in a given interval.
Probability Distributions
A probability distribution describes the likelihood of each possible outcome of a random variable. There are numerous types of probability distributions, including the uniform distribution, normal distribution, and binomial distribution.
Expectation Values
The expectation value of a random variable is a key concept in probability theory. It provides a measure of the "average" outcome of a random variable and is often used to make predictions about future outcomes.
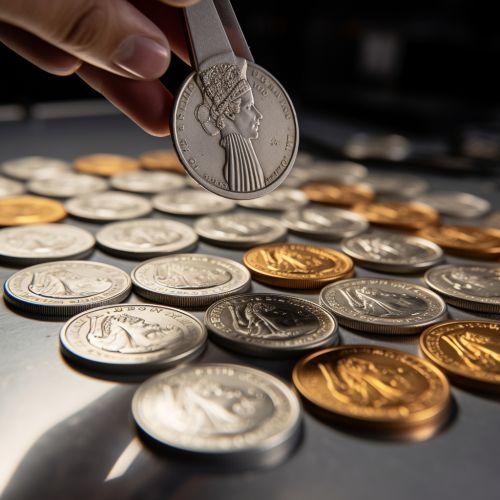
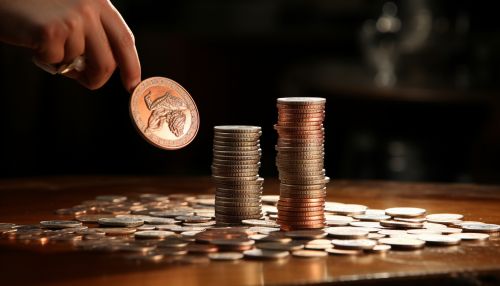
Statistical Inference
Statistical inference is the process of using data to make conclusions about a population or process. It involves the use of statistical models and methods to draw conclusions from data and to make predictions. Key concepts in statistical inference include hypothesis testing, confidence intervals, and regression analysis.
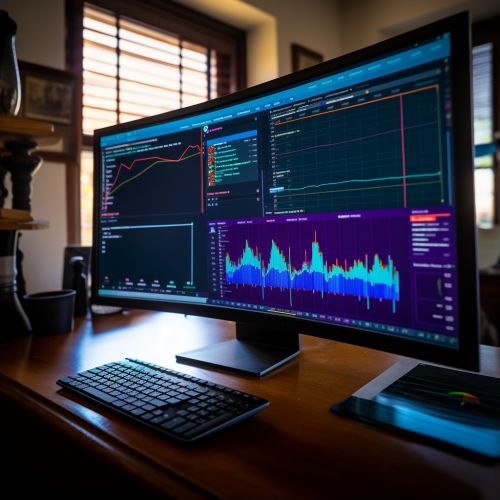
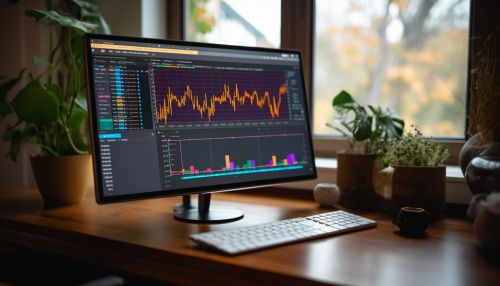
Hypothesis Testing
hypothesis testing is a method used in statistics to test the validity of a claim or hypothesis about a population based on a sample of data. It involves the use of statistical tests such as the t-test, chi-square test, and ANOVA.
Confidence Intervals
A confidence interval is a range of values that is likely to contain the true value of an unknown population parameter. The confidence level represents the frequency (i.e., the proportion) of possible confidence intervals that contain the true value of the unknown population parameter.
Regression Analysis
Regression analysis is a set of statistical processes for estimating the relationships among variables. It includes many techniques for modeling and analyzing several variables, when the focus is on the relationship between a dependent variable and one or more independent variables.

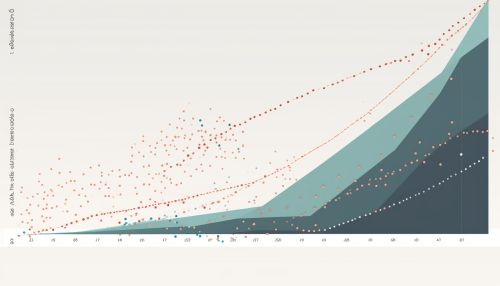
Applications
Mathematical statistics has a wide range of applications in various fields. In economics, it is used to model and predict economic behavior. In engineering, it is used in quality control and reliability analysis. In the natural and social sciences, it is used to test hypotheses and make predictions based on empirical data.

