Intra-class variation
Introduction
Intra-class variation refers to the diversity or differences that exist within a single class or category of objects or entities. This concept is widely applicable across various fields such as machine learning, statistics, biology, and sociology. It is a crucial aspect to consider in any analysis or study as it can significantly impact the results and interpretations.
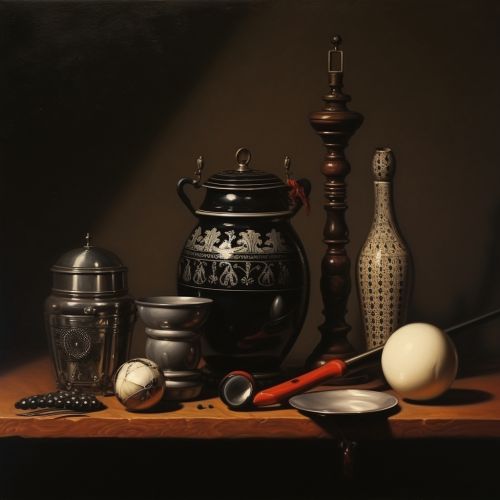
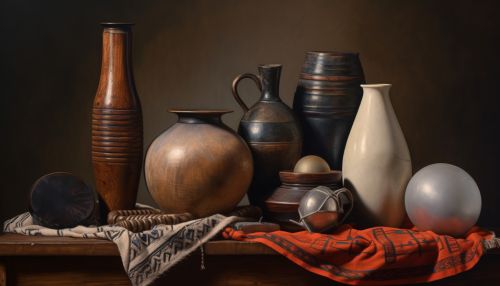
In Machine Learning
In the field of machine learning, intra-class variation plays a vital role in the performance and accuracy of models. It refers to the differences in the features or characteristics of data points that belong to the same class. For example, in an image recognition task, images of the same object (class) can have variations in terms of lighting, angle, size, and other factors. These variations within the same class can pose challenges in accurately classifying the data points.
Impact on Model Performance
Intra-class variation can significantly impact the performance of machine learning models. High intra-class variation can make it difficult for models to accurately classify data points, leading to lower accuracy and precision. On the other hand, low intra-class variation can make the model overfit to the training data, resulting in poor generalization to unseen data.
Handling Intra-class Variation
Several techniques can be used to handle intra-class variation in machine learning. These include data augmentation, feature extraction, and the use of complex models that can capture the variations within the class. For instance, Convolutional Neural Networks (CNNs) are often used in image recognition tasks due to their ability to handle intra-class variation.
In Statistics
In statistics, intra-class variation is often encountered in the analysis of variance (ANOVA), where it refers to the variability within groups or classes. It is an essential factor in determining the significance of differences between groups.
Analysis of Variance (ANOVA)
In ANOVA, the total variation in the data is partitioned into intra-class and inter-class variation. The intra-class variation, also known as the within-group variation, measures the variability of data points within each group. The inter-class variation, or between-group variation, measures the variability between the group means.
Impact on Statistical Tests
The level of intra-class variation can significantly impact the results of statistical tests. High intra-class variation can lead to a failure to detect significant differences between groups, while low intra-class variation can increase the power of the test to detect differences.
In Biology
In biology, intra-class variation refers to the differences that exist within a single class or group of organisms. This can include variations in physical characteristics, genetic makeup, behavior, and other traits.
Genetic Variation
Intra-class genetic variation is a key factor in evolution and biodiversity. It is the raw material upon which natural selection acts, leading to the evolution of new species. Genetic variation within a class can occur due to mutations, gene flow, genetic drift, and other processes.
Phenotypic Variation
Phenotypic variation refers to the differences in physical characteristics within a class of organisms. This can include variations in size, color, shape, and other observable traits. Phenotypic variation can be influenced by genetic factors, environmental factors, or a combination of both.
In Sociology
In sociology, intra-class variation refers to the differences that exist within a single social class. These differences can be in terms of wealth, education, occupation, lifestyle, and other factors.
Impact on Social Analysis
Intra-class variation can significantly impact social analysis and the understanding of social dynamics. It can challenge the stereotypical views of social classes and highlight the diversity and complexity within each class.