Bayesian Statistics
Introduction
Bayesian statistics is a theory in the field of statistics which provides a framework for updating probabilities based on evidence. It is named after the Reverend Thomas Bayes, who provided the first mathematical formulation of the theory. Bayesian statistics contrasts with frequentist statistics, the other main approach to statistical inference, in that it assigns probability distributions to unknown parameters.
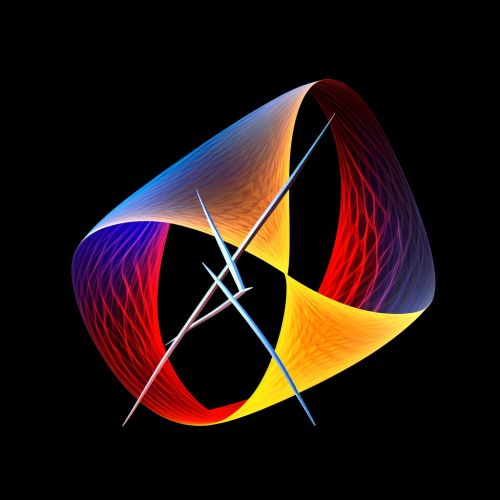
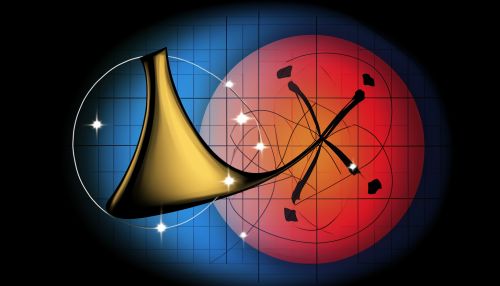
Historical Background
The concept of Bayesian statistics originated from the work of Thomas Bayes, an 18th-century English statistician and Presbyterian minister. His work "An Essay towards solving a Problem in the Doctrine of Chances" was published posthumously in 1763, introducing what is now known as Bayes' theorem. This theorem provides a mathematical method for updating probabilities based on new data.
Bayesian Theory
The core of Bayesian statistics is Bayes' theorem, which describes the relationship between conditional probabilities. The theorem is typically expressed as follows:
P(A|B) = [P(B|A) * P(A)] / P(B)
Where: - P(A|B) is the posterior probability, or the updated probability of event A given that event B has occurred. - P(B|A) is the likelihood, or the probability of event B given that event A has occurred. - P(A) is the prior probability, or the initial probability of event A before new data is considered. - P(B) is the evidence, or the total probability of event B.
Bayesian Inference
Bayesian inference is the process of updating probabilities based on new data using Bayes' theorem. The process begins with a prior probability, which is updated with new data to produce a posterior probability. This posterior probability then becomes the new prior probability for subsequent updates. This iterative process is a key feature of Bayesian statistics and distinguishes it from frequentist statistics, which does not update probabilities based on new data.
Bayesian Models
Bayesian models are statistical models that incorporate prior knowledge about parameters in the form of probability distributions. These models are used in a wide range of fields, including machine learning, artificial intelligence, and data analysis. The choice of prior distribution is a critical aspect of Bayesian modeling and can significantly influence the results of the analysis.
Bayesian vs Frequentist Statistics
Bayesian and frequentist statistics represent two different philosophies of statistical inference. Frequentist statistics, the traditional approach, interprets probabilities as long-run frequencies of events. In contrast, Bayesian statistics interprets probabilities as degrees of belief, which can be updated as new data becomes available. This fundamental difference leads to different approaches to statistical modeling and hypothesis testing.
Applications of Bayesian Statistics
Bayesian statistics has a wide range of applications in various fields. In machine learning and artificial intelligence, Bayesian methods are used for probabilistic modeling and decision making. In data analysis, Bayesian methods can handle complex models and incorporate prior knowledge. In addition, Bayesian methods are used in fields such as epidemiology, genetics, and economics, among others.
Criticisms and Limitations of Bayesian Statistics
Despite its advantages, Bayesian statistics also has its criticisms and limitations. One criticism is the subjective nature of the prior probability, which can lead to different results depending on the analyst's beliefs. Another limitation is the computational complexity of Bayesian methods, which can be challenging for large datasets or complex models.
Conclusion
Bayesian statistics offers a powerful and flexible framework for statistical inference. It allows for the incorporation of prior knowledge and the updating of probabilities based on new data. Despite its criticisms and limitations, Bayesian statistics continues to be widely used in various fields, contributing to advancements in machine learning, artificial intelligence, data analysis, and more.
See Also
- Frequentist statistics - Probability theory - Statistical inference - Machine learning - Artificial intelligence