Environmental Modeling
Introduction
Environmental modeling is a broad field of study that involves the use of mathematical and computational tools to understand and predict the behavior of natural systems. This field is an integral part of environmental science, ecology, earth science, and environmental engineering. It is used to analyze and predict a wide range of environmental phenomena, from the spread of pollutants in air and water, to the growth and decay of biological populations, to the impact of human activities on climate change.
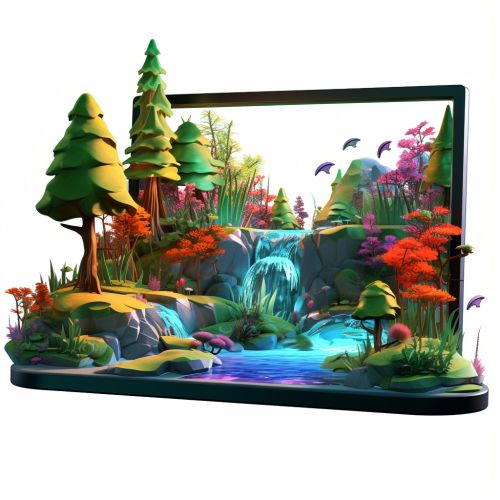
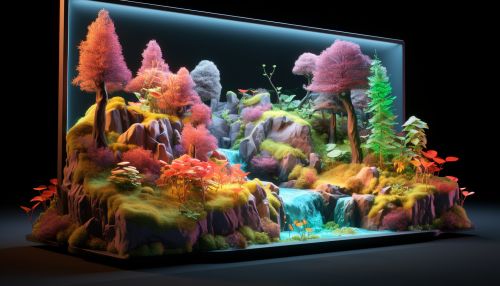
Types of Environmental Models
Environmental models can be broadly classified into four types: empirical models, deterministic models, stochastic models, and hybrid models.
Empirical Models
Empirical models are based on observed data. They use statistical methods to identify relationships between different variables. These models are often used when the underlying mechanisms of the system are not well understood, or when it is not feasible to model the system in detail.
Deterministic Models
Deterministic models are based on known physical, chemical, or biological laws. They predict a unique outcome for a given set of initial conditions. These models are often used when the system is well understood and can be described by a set of differential equations.
Stochastic Models
Stochastic models incorporate randomness into the model. They are used when the system is influenced by random factors, or when the system itself is inherently random. These models predict a range of possible outcomes, each with a certain probability.
Hybrid Models
Hybrid models combine elements of empirical, deterministic, and stochastic models. They are used when the system is partially understood, or when it is necessary to balance the accuracy of the model with the computational cost.
Applications of Environmental Modeling
Environmental modeling has a wide range of applications in various fields. Some of the key applications are discussed below.
Climate Change Modeling
Climate change modeling is a key application of environmental modeling. It involves the use of complex computer models to predict the future state of the climate based on various scenarios of greenhouse gas emissions. These models are used to understand the potential impacts of climate change and to guide policy decisions on mitigation and adaptation strategies.
Air Quality Modeling
Air quality modeling is used to predict the concentration of pollutants in the atmosphere. These models are used to assess the impact of various sources of pollution, to design air quality management strategies, and to forecast air quality conditions.
Water Quality Modeling
Water quality modeling is used to predict the concentration of pollutants in surface and groundwater. These models are used to assess the impact of various sources of pollution, to design water quality management strategies, and to forecast water quality conditions.
Ecological Modeling
Ecological modeling is used to understand and predict the dynamics of biological populations and communities. These models are used to assess the impact of various environmental factors on biodiversity, to design conservation strategies, and to predict the spread of invasive species.
Challenges in Environmental Modeling
Despite its many applications, environmental modeling faces several challenges. These include the complexity of natural systems, the uncertainty in model parameters, the lack of data, and the computational cost of running complex models.
Complexity of Natural Systems
Natural systems are inherently complex, with many interacting components and nonlinear dynamics. This complexity makes it difficult to develop accurate and predictive models.
Uncertainty in Model Parameters
Many environmental models rely on parameters that are difficult to measure directly, such as the rate of chemical reactions in the atmosphere or the growth rate of biological populations. This leads to uncertainty in the model predictions.
Lack of Data
Environmental modeling often requires large amounts of data, which can be difficult to obtain. This is particularly true for remote or inaccessible areas, or for variables that are difficult to measure directly.
Computational Cost
Running complex environmental models can be computationally expensive, particularly for large-scale or high-resolution models. This can limit the feasibility of running these models on a regular basis or for long-term predictions.
Future Directions in Environmental Modeling
Despite these challenges, there are several promising directions for future research in environmental modeling. These include the development of more accurate and efficient models, the integration of models across different scales and disciplines, and the use of new data sources and computational tools.
More Accurate and Efficient Models
There is ongoing research to develop more accurate and efficient environmental models. This includes the development of new mathematical and computational methods, as well as the refinement of existing models based on new scientific understanding.
Integration of Models Across Scales and Disciplines
There is a growing recognition of the need to integrate models across different scales and disciplines. This includes the integration of climate models with ecological models, or the integration of air quality models with health impact models.
Use of New Data Sources and Computational Tools
The advent of new data sources, such as remote sensing and citizen science, and new computational tools, such as machine learning and cloud computing, offers new opportunities for environmental modeling.