Dynamic Causal Modeling
Introduction
Dynamic Causal Modeling (DCM) is a statistical technique used in neuroimaging to understand the causal interactions among brain regions. It is a hypothesis-driven approach that allows researchers to test specific models of effective connectivity in the brain.
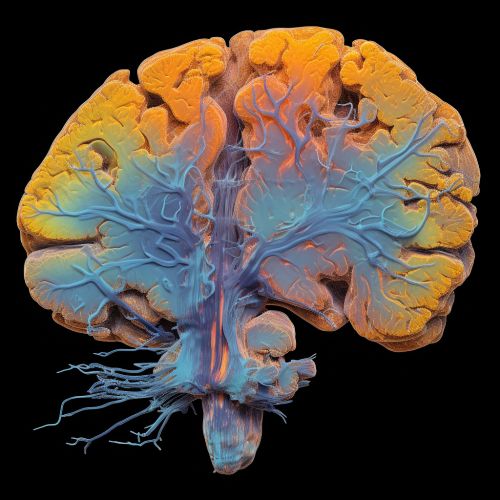
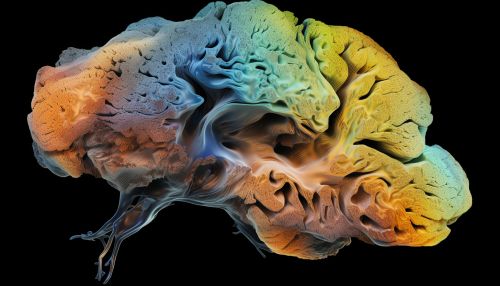
Overview
DCM was developed by the Wellcome Trust Centre for Neuroimaging at University College London. It is implemented in the Statistical Parametric Mapping (SPM) software, a widely used tool in neuroimaging research.
The technique is based on Bayesian inference principles, which allows it to quantify the uncertainty associated with the estimated parameters. This makes DCM a powerful tool for understanding the underlying neural processes that give rise to observed brain activity.
Methodology
DCM uses a biophysical model of neural dynamics to predict the observed neuroimaging data. This model includes parameters that describe the strength and direction of connections between brain regions, as well as the influence of experimental manipulations on these connections.
The model is fitted to the data using a variational Bayes approach, which approximates the complex posterior distribution of the model parameters. This results in a set of estimated parameters and their associated uncertainties.
Applications
DCM has been used in a wide range of neuroimaging studies to investigate the effective connectivity in the brain. These include studies of cognitive functions such as attention, memory, and decision making, as well as clinical studies of neurological and psychiatric disorders.
Limitations and Criticisms
Despite its strengths, DCM has also been subject to criticism. Some researchers argue that the biophysical model used in DCM is overly simplistic and does not accurately capture the complexity of neural dynamics. Others have raised concerns about the reliability of DCM estimates, particularly when the model is applied to noisy or low-quality data.
Future Directions
Recent developments in DCM aim to address these criticisms by incorporating more realistic models of neural dynamics and by improving the estimation methods. These advances promise to further enhance the utility of DCM in neuroimaging research.