Data Science in Urban Planning
Introduction
Data science has emerged as a critical tool in urban planning, enabling planners to analyze vast amounts of data to make informed decisions. This article delves into the application of data science in urban planning, exploring its methodologies, tools, and impact on the development of urban areas.
Historical Context
Urban planning has evolved significantly over the centuries, from ancient city designs to modern smart cities. The integration of data science into urban planning began in the late 20th century, with the advent of computers and the internet. Early applications focused on basic data analysis and geographic information systems (GIS). Over time, the field has expanded to include advanced analytics, machine learning, and big data.
Methodologies
Data science in urban planning employs various methodologies to analyze and interpret data. These include:
Geographic Information Systems (GIS)
GIS is a foundational tool in urban planning, allowing planners to visualize, analyze, and interpret spatial data. GIS integrates various data types, including demographic, environmental, and infrastructural data, to create comprehensive maps and models.
Machine Learning
Machine learning algorithms are used to predict urban trends and patterns. These algorithms analyze historical data to forecast future developments, such as population growth, traffic patterns, and housing demands.
Big Data Analytics
Big data analytics involves the processing and analysis of large datasets to uncover hidden patterns and correlations. In urban planning, big data can be sourced from various channels, including social media, sensors, and public records.
Applications
Data science applications in urban planning are diverse and impactful. Some key applications include:
Traffic Management
Data science helps in optimizing traffic flow and reducing congestion. By analyzing real-time traffic data, planners can design better road networks and implement intelligent traffic systems.
Environmental Monitoring
Urban planners use data science to monitor environmental factors such as air quality, noise levels, and green space distribution. This information is crucial for creating sustainable and livable urban environments.
Housing and Real Estate
Data science aids in predicting housing market trends and identifying areas for development. By analyzing demographic and economic data, planners can make informed decisions about zoning and land use.
Public Safety
Crime data analysis helps in identifying high-risk areas and deploying resources effectively. Predictive policing models can forecast crime hotspots, enabling proactive measures to enhance public safety.
Tools and Technologies
Several tools and technologies are integral to data science in urban planning:
Software
- **ArcGIS**: A powerful GIS software used for mapping and spatial analysis. - **QGIS**: An open-source GIS application that supports viewing, editing, and analysis of geospatial data. - **R and Python**: Programming languages widely used for statistical analysis and machine learning.
Data Sources
- **Census Data**: Provides comprehensive demographic information. - **Sensor Networks**: Collect real-time data on various environmental and infrastructural parameters. - **Social Media**: Offers insights into public sentiment and behavior patterns.
Challenges
Despite its benefits, the integration of data science in urban planning faces several challenges:
Data Privacy
The collection and analysis of large datasets raise concerns about privacy and data security. Ensuring that data is anonymized and used ethically is paramount.
Data Quality
The accuracy and reliability of data are critical. Inaccurate or incomplete data can lead to flawed analyses and poor decision-making.
Interdisciplinary Collaboration
Effective urban planning requires collaboration between data scientists, urban planners, and other stakeholders. Bridging the gap between these disciplines can be challenging but is essential for successful outcomes.
Future Directions
The future of data science in urban planning is promising, with advancements in technology and data analytics driving innovation. Emerging trends include:
Smart Cities
The concept of smart cities involves the use of data and technology to enhance urban living. Data science plays a crucial role in developing smart city solutions, from intelligent transportation systems to energy-efficient buildings.
Internet of Things (IoT)
IoT devices generate vast amounts of data that can be used to monitor and manage urban infrastructure. Integrating IoT with data science enables real-time decision-making and predictive maintenance.
Artificial Intelligence (AI)
AI technologies, such as deep learning and natural language processing, are being increasingly applied in urban planning. These technologies can automate complex analyses and provide deeper insights into urban dynamics.
Conclusion
Data science is transforming urban planning by providing powerful tools and methodologies to analyze and interpret complex data. As technology continues to evolve, the role of data science in shaping the future of urban environments will only grow more significant.
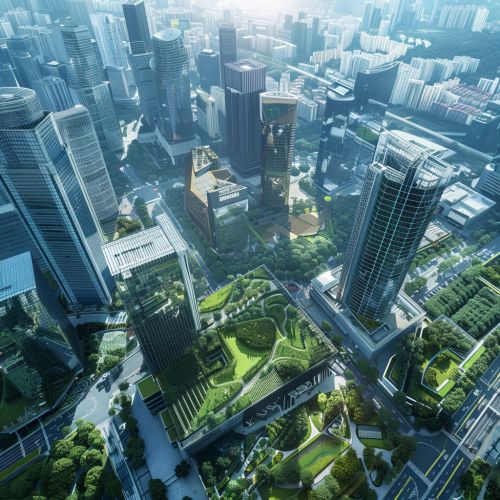
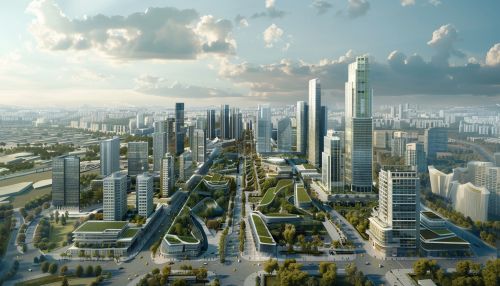