Data Quality
Introduction
Data quality refers to the condition of a set of values of qualitative or quantitative variables. It can be characterized by the following aspects: accuracy, completeness, update status, relevance, consistency across data sources, reliability, appropriate presentation, and accessibility. It is crucial in various fields such as data mining, machine learning, data integration, and any other data-related tasks.
Importance of Data Quality
Data quality is of high importance in any data-driven decision-making process. Poor data quality can lead to inaccurate decision-making, inefficiency, and financial loss. For instance, in the business world, companies rely on data to make strategic decisions. If the data is of poor quality, it can lead to misguided strategies that can have detrimental effects on the company's performance and profitability.
Data Quality Dimensions
Data quality dimensions are the various aspects or facets of data quality. They provide a framework for assessing, managing, and improving the quality of data. The most common dimensions of data quality include:
Accuracy
Accuracy refers to the degree to which data correctly describes the "real world" object or event being described. It is one of the most critical dimensions of data quality.
Completeness
Completeness refers to the extent to which data is not missing and is of sufficient breadth and depth for the task at hand.
Consistency
Consistency refers to the extent to which data is consistent, within the same data set or across multiple data sets.
Timeliness
Timeliness refers to the extent to which data is sufficiently up-to-date for the task at hand.
Data Quality Management
Data quality management involves the implementation of practices, processes, and technologies to maintain and improve data quality. It includes data profiling, data cleaning, data integration, and data enrichment.
Data Profiling
Data profiling is the process of examining the data available in an existing data source and collecting statistics and information about that data.
Data Cleaning
Data cleaning is the process of detecting and correcting (or removing) corrupt or inaccurate records from a record set, table, or database.
Data Integration
Data integration involves combining data residing in different sources and providing users with a unified view of these data.
Data Enrichment
Data enrichment is the process of enhancing, refining, and improving raw data.
Data Quality in Different Domains
Data quality requirements and standards can vary significantly across different domains. For instance, in the healthcare industry, data quality is of utmost importance as it can impact patient care and outcomes. In the financial industry, data quality is crucial for accurate financial reporting and regulatory compliance.
Data Quality Tools
Data quality tools are software applications used to analyze, improve, and control the quality of data. They provide functionalities such as data profiling, data cleaning, data validation, and data monitoring.
Challenges in Ensuring Data Quality
Ensuring data quality is not a straightforward task. It involves various challenges, such as dealing with large volumes of data, data integration from multiple sources, handling unstructured data, and maintaining data privacy and security.
Conclusion
In conclusion, data quality is a critical aspect in various fields, and ensuring high data quality is crucial for accurate decision-making and efficient operations. Despite the challenges, various tools and techniques are available to manage and improve data quality.
See Also

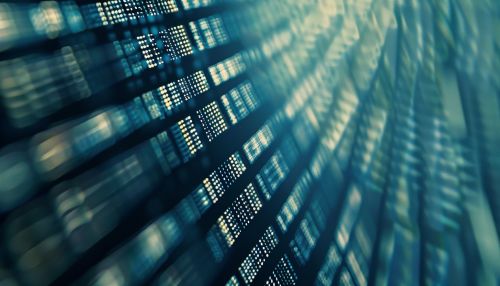