Data Quality Assurance
Introduction
Data Quality Assurance (DQA) is a critical aspect of data management that ensures the accuracy, consistency, and reliability of data throughout its lifecycle. It encompasses a range of processes and methodologies aimed at detecting and correcting errors, validating data, and maintaining data integrity. DQA is essential for organizations to make informed decisions, comply with regulations, and maintain trust with stakeholders.
Key Concepts in Data Quality Assurance
Data Quality Dimensions
Data quality is often assessed based on several dimensions, including:
- **Accuracy**: The degree to which data correctly describes the real-world object or event it represents.
- **Completeness**: The extent to which all required data is available.
- **Consistency**: The absence of contradictions within the data.
- **Timeliness**: The degree to which data is up-to-date and available within a useful time frame.
- **Validity**: The extent to which data conforms to defined formats and rules.
- **Uniqueness**: Ensuring that each record is unique and not duplicated.
Data Quality Frameworks
Several frameworks and models guide the implementation of DQA processes. Notable frameworks include:
- **Total Data Quality Management (TDQM)**: Focuses on continuous improvement and the integration of data quality into all aspects of data management.
- **Data Quality Assessment Framework (DQAF)**: Developed by the International Monetary Fund (IMF), this framework provides a structured approach to assessing data quality.
- **ISO 8000**: An international standard for data quality management, providing guidelines for data quality assessment and improvement.
Data Quality Assurance Processes
Data Profiling
Data profiling involves analyzing data to understand its structure, content, and quality. This process helps identify anomalies, inconsistencies, and areas for improvement. Key activities in data profiling include:
- **Column Analysis**: Examining the content of individual columns to identify patterns, missing values, and outliers.
- **Cross-Column Analysis**: Analyzing relationships between columns to detect inconsistencies and dependencies.
- **Rule-Based Analysis**: Applying predefined rules to validate data against expected patterns and standards.
Data Cleansing
Data cleansing, also known as data scrubbing, involves detecting and correcting errors and inconsistencies in data. This process includes:
- **Standardization**: Converting data into a consistent format.
- **Deduplication**: Identifying and removing duplicate records.
- **Error Correction**: Fixing inaccuracies and filling in missing values.
- **Validation**: Ensuring data conforms to predefined rules and standards.
Data Enrichment
Data enrichment enhances the value of data by adding additional information from external sources. This process can improve data accuracy, completeness, and relevance. Common enrichment techniques include:
- **Appending Missing Data**: Adding missing information from external databases.
- **Geocoding**: Adding geographical coordinates to address data.
- **Data Integration**: Combining data from multiple sources to create a comprehensive dataset.
Data Monitoring
Continuous monitoring of data quality is essential to maintain high standards. Data monitoring involves:
- **Automated Alerts**: Setting up alerts to notify stakeholders of data quality issues.
- **Regular Audits**: Conducting periodic reviews to assess data quality.
- **Performance Metrics**: Tracking key performance indicators (KPIs) to measure data quality over time.
Tools and Technologies for Data Quality Assurance
Several tools and technologies support DQA processes, including:
- **Data Quality Software**: Specialized software solutions that provide functionalities for data profiling, cleansing, and monitoring. Examples include Informatica Data Quality, Talend Data Quality, and IBM InfoSphere QualityStage.
- **Data Integration Platforms**: Tools that facilitate the integration and transformation of data from multiple sources. Examples include Apache Nifi, Microsoft SQL Server Integration Services (SSIS), and Oracle Data Integrator (ODI).
- **Master Data Management (MDM)**: Systems that ensure the consistency and accuracy of key business data across the organization. Examples include SAP Master Data Governance, Informatica MDM, and IBM InfoSphere MDM.
Challenges in Data Quality Assurance
Despite the availability of tools and frameworks, organizations face several challenges in implementing effective DQA processes:
- **Data Volume and Complexity**: The increasing volume and complexity of data make it difficult to maintain high-quality standards.
- **Data Silos**: Isolated data systems can lead to inconsistencies and hinder data integration efforts.
- **Resource Constraints**: Limited resources and budget constraints can impact the effectiveness of DQA initiatives.
- **Changing Data Requirements**: Evolving business needs and regulatory requirements necessitate continuous updates to DQA processes.
Best Practices for Data Quality Assurance
To overcome challenges and ensure effective DQA, organizations should adopt the following best practices:
- **Establish Data Governance**: Implement a robust data governance framework to define roles, responsibilities, and policies for data management.
- **Invest in Training**: Provide training and resources to employees to enhance their data quality skills and awareness.
- **Leverage Automation**: Utilize automated tools and technologies to streamline DQA processes and reduce manual effort.
- **Foster a Data-Driven Culture**: Encourage a culture of data-driven decision-making and emphasize the importance of data quality across the organization.
- **Regularly Review and Update Processes**: Continuously review and update DQA processes to adapt to changing business needs and regulatory requirements.
Case Studies
Financial Services
In the financial services industry, data quality is critical for regulatory compliance and risk management. A leading bank implemented a comprehensive DQA program, leveraging data profiling and cleansing tools to improve the accuracy and completeness of customer data. As a result, the bank achieved better compliance with regulatory requirements and enhanced its risk management capabilities.
Healthcare
In the healthcare sector, accurate and reliable data is essential for patient care and research. A major hospital implemented an MDM system to ensure the consistency of patient records across different departments. This initiative led to improved patient care, reduced medical errors, and enhanced data-driven research capabilities.
Retail
A global retail company faced challenges with inconsistent product data across its e-commerce platforms. By implementing a data quality software solution, the company was able to standardize product information, eliminate duplicates, and enrich data with additional attributes. This resulted in a better customer experience and increased sales.
Future Trends in Data Quality Assurance
The field of DQA is continuously evolving, with several emerging trends shaping its future:
- **Artificial Intelligence (AI) and Machine Learning (ML)**: AI and ML technologies are being increasingly used to automate data quality processes, identify patterns, and predict data quality issues.
- **Big Data and Real-Time Analytics**: The growing adoption of big data and real-time analytics necessitates advanced DQA techniques to handle large volumes of data and ensure timely insights.
- **Blockchain Technology**: Blockchain offers potential for enhancing data integrity and traceability, particularly in industries such as supply chain and finance.
- **Data Privacy and Security**: With increasing concerns about data privacy and security, DQA processes must incorporate measures to protect sensitive information and comply with regulations such as the General Data Protection Regulation (GDPR).
Conclusion
Data Quality Assurance is a vital component of effective data management, ensuring that data is accurate, consistent, and reliable. By adopting robust DQA processes and leveraging advanced tools and technologies, organizations can enhance their decision-making capabilities, comply with regulations, and maintain trust with stakeholders. As the field continues to evolve, staying abreast of emerging trends and best practices will be essential for maintaining high data quality standards.
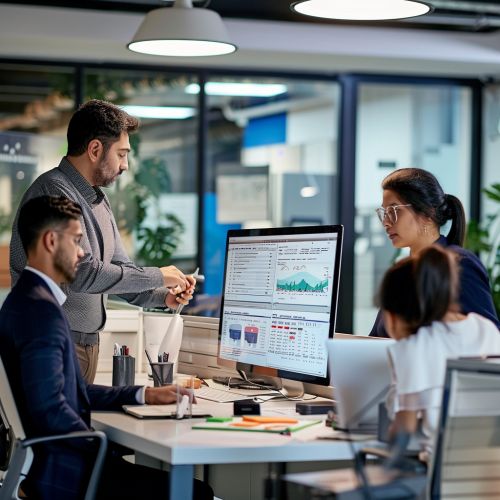
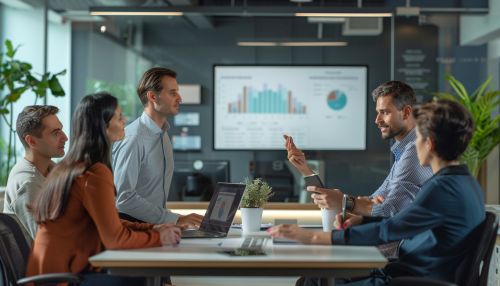
See Also
- Data Governance
- Master Data Management
- Data Profiling
- Data Cleansing
- Data Integration
- Data Privacy
- Big Data
- Artificial Intelligence