Data Lifecycle Management
Introduction
Data Lifecycle Management (DLM) is a comprehensive approach to managing the flow of data throughout its lifecycle, from creation and initial storage to the time it becomes obsolete and is deleted. This process encompasses a variety of stages, including data creation, storage, usage, sharing, archiving, and deletion. DLM aims to ensure data integrity, security, and availability while optimizing storage resources and complying with regulatory requirements.
Stages of Data Lifecycle Management
Data Creation
Data creation is the initial phase where data is generated or acquired. This can occur through various means such as data entry, data capture from sensors, or data import from external sources. The quality and accuracy of data at this stage are crucial, as they impact all subsequent stages. Proper metadata tagging and classification are essential to facilitate effective data management.
Data Storage
Once data is created, it needs to be stored in a manner that ensures its integrity and accessibility. Storage solutions can range from on-premises databases and file systems to cloud storage services. The choice of storage depends on factors such as data volume, access frequency, and security requirements. Techniques like data deduplication and compression can optimize storage efficiency.
Data Usage
Data usage involves accessing and manipulating data to derive value. This stage includes data analysis, reporting, and decision-making processes. Ensuring data quality and consistency is vital for accurate analysis. Data governance policies play a significant role in managing access controls and ensuring that data usage complies with organizational and regulatory standards.
Data Sharing
Data sharing refers to the distribution of data within or outside the organization. This can be achieved through APIs, data integration platforms, or data exchange protocols. Secure data sharing practices, such as encryption and access controls, are essential to protect sensitive information. Data sharing agreements and policies help define the terms and conditions for data exchange.
Data Archiving
Data archiving involves moving inactive or infrequently accessed data to long-term storage solutions. Archived data is retained for historical reference, compliance, or future analysis. Archiving strategies should ensure data retrievability and integrity while minimizing storage costs. Technologies like hierarchical storage management (HSM) and cloud archiving services are commonly used.
Data Deletion
Data deletion is the final stage of the data lifecycle, where data that is no longer needed is securely removed. This process must comply with data retention policies and regulatory requirements. Secure deletion methods, such as data wiping and shredding, ensure that deleted data cannot be recovered. Proper documentation of the deletion process is essential for audit purposes.
Data Governance and Compliance
Data governance encompasses the policies, procedures, and standards that ensure effective data management throughout its lifecycle. It involves defining roles and responsibilities, establishing data quality standards, and implementing data stewardship practices. Compliance with regulatory frameworks, such as GDPR, HIPAA, and CCPA, is a critical aspect of data governance. Organizations must ensure that data handling practices align with legal and ethical standards to avoid penalties and reputational damage.
Data Security
Data security is a fundamental component of DLM, aimed at protecting data from unauthorized access, breaches, and other threats. Security measures include encryption, access controls, authentication mechanisms, and regular security audits. Implementing a robust data security strategy helps safeguard sensitive information and maintain data integrity. Organizations should also have incident response plans in place to address potential security breaches promptly.
Data Quality Management
Data quality management focuses on maintaining the accuracy, completeness, and reliability of data throughout its lifecycle. This involves data profiling, data cleansing, and data validation processes. High-quality data is essential for informed decision-making and effective business operations. Data quality metrics and continuous monitoring help identify and address data quality issues proactively.
Technologies and Tools for DLM
Various technologies and tools support DLM processes, including:
- **Data Management Platforms (DMPs):** These platforms provide comprehensive solutions for data integration, storage, and governance.
- **Data Warehousing Solutions:** Data warehouses enable the consolidation and analysis of large volumes of data from multiple sources.
- **Data Integration Tools:** These tools facilitate the seamless exchange and transformation of data between different systems.
- **Data Archiving Solutions:** Technologies like cloud archiving and HSM help manage long-term data storage efficiently.
- **Data Security Tools:** Encryption software, access management systems, and security information and event management (SIEM) solutions enhance data protection.
Best Practices for Implementing DLM
Implementing an effective DLM strategy involves several best practices:
- **Define Clear Policies:** Establish comprehensive data management policies that cover all stages of the data lifecycle.
- **Ensure Data Quality:** Implement robust data quality management practices to maintain data accuracy and reliability.
- **Adopt Scalable Storage Solutions:** Choose storage solutions that can scale with data growth and provide flexibility.
- **Implement Strong Security Measures:** Protect data with encryption, access controls, and regular security assessments.
- **Regularly Review and Update Policies:** Continuously review and update data management policies to adapt to changing requirements and technologies.
Challenges in Data Lifecycle Management
Organizations may face several challenges in implementing DLM, including:
- **Data Volume and Variety:** Managing large volumes of diverse data types can be complex and resource-intensive.
- **Data Silos:** Data stored in isolated systems can hinder effective data integration and sharing.
- **Compliance Requirements:** Navigating complex regulatory landscapes requires thorough understanding and adherence to various laws and standards.
- **Security Threats:** Evolving cybersecurity threats necessitate continuous vigilance and advanced security measures.
- **Resource Constraints:** Limited resources and budget constraints can impact the implementation of comprehensive DLM strategies.
Future Trends in Data Lifecycle Management
The field of DLM is continuously evolving, with several emerging trends shaping its future:
- **Artificial Intelligence (AI) and Machine Learning (ML):** AI and ML technologies are being leveraged to automate data management processes, enhance data quality, and improve predictive analytics.
- **Edge Computing:** The rise of edge computing is driving the need for efficient data management at the edge of networks, closer to data sources.
- **Data Privacy Enhancements:** Growing concerns about data privacy are leading to the development of advanced privacy-preserving techniques, such as differential privacy and homomorphic encryption.
- **Blockchain for Data Integrity:** Blockchain technology is being explored for its potential to ensure data integrity and traceability in DLM processes.
- **Hybrid Cloud Solutions:** The adoption of hybrid cloud environments is increasing, necessitating integrated data management strategies across on-premises and cloud infrastructures.
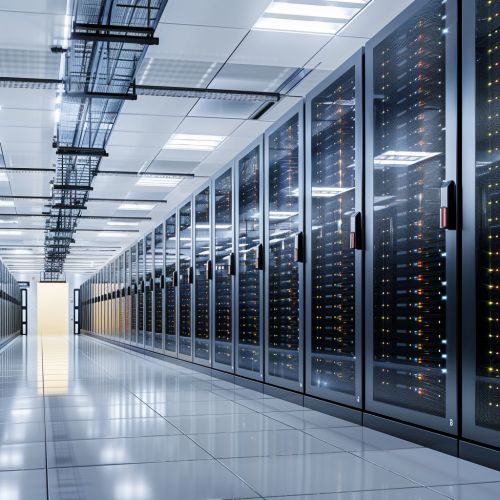
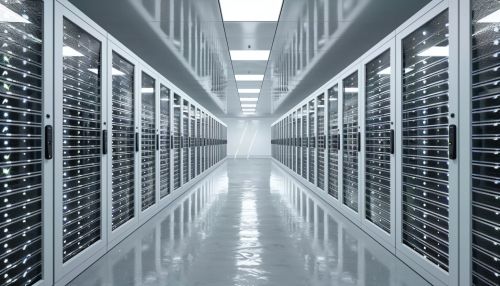