Canonical Correlation Analysis
Introduction
Canonical Correlation Analysis (CCA) is a multivariate statistical procedure that originated from the work of Hotelling in the 1930s. It is a method used to identify and measure the associations among two sets of variables. CCA is used in various fields, including psychology, environmental science, economics, and genomics, among others. It is a powerful tool for analyzing multivariate data with multiple measurements multivariate statistics.
Mathematical Background
CCA is based on the principle of maximizing the correlation between linear combinations of multivariate observations. Given two random vectors X and Y, the canonical correlations are the correlations between linear combinations of X and Y that have been chosen to maximize this correlation. The canonical variables are these linear combinations that have the maximum correlation.
The mathematical formulation of CCA involves the calculation of eigenvalues and eigenvectors of certain matrices derived from the data. The eigenvalues represent the canonical correlations, and the eigenvectors are used to form the canonical variables.
Assumptions
CCA makes several assumptions about the data. It assumes that the variables are multivariate normal, that the observations are independent, and that the relationships between variables are linear. Violations of these assumptions can lead to misleading results.
Applications
CCA has a wide range of applications. It is used in psychology for the study of relationships between sets of psychological variables. In environmental science, it is used to study the relationships between different sets of environmental variables. In economics, it is used to study the relationships between sets of economic indicators. In genomics, it is used to study the relationships between sets of genetic markers.
Limitations
While CCA is a powerful tool, it has its limitations. The interpretation of the results can be challenging, especially when dealing with large sets of variables. It is also sensitive to outliers and can be influenced by multicollinearity. Lastly, the assumptions of CCA may not hold in all situations, leading to potential inaccuracies in the results.
Extensions and Variations
Over the years, several extensions and variations of CCA have been developed to overcome its limitations and extend its applicability. These include Partial Canonical Correlation Analysis, Regularized Canonical Correlation Analysis, and Kernel Canonical Correlation Analysis, among others.
Conclusion
CCA is a powerful and versatile statistical tool for analyzing multivariate data. Despite its limitations, its ability to uncover hidden relationships in data makes it a valuable tool in many fields of study.
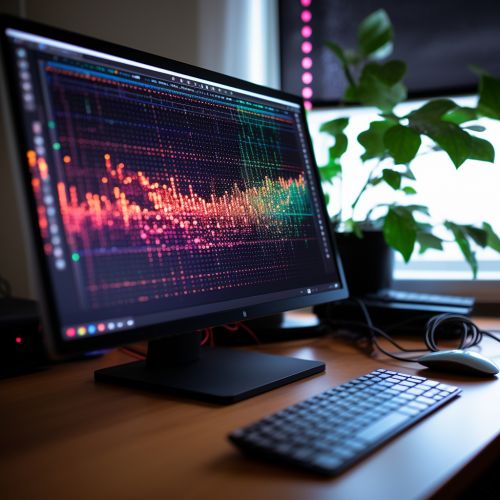
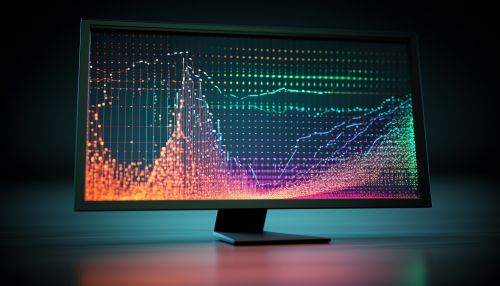