Artificial Intelligence in Radiology
Introduction
Artificial Intelligence (AI) has become an integral part of modern radiology, revolutionizing the way medical images are analyzed and interpreted. This article delves into the various applications, methodologies, and impacts of AI in radiology, providing a comprehensive overview of this rapidly evolving field.
Historical Background
The integration of AI into radiology began in the late 20th century with the advent of computer-aided diagnosis (CAD) systems. These early systems were primarily rule-based and relied on predefined algorithms to detect abnormalities in medical images. Over the years, advancements in machine learning and deep learning have significantly enhanced the capabilities of AI in radiology, leading to more accurate and efficient diagnostic tools.
AI Technologies in Radiology
AI technologies used in radiology can be broadly categorized into machine learning, deep learning, and natural language processing (NLP).
Machine Learning
Machine learning algorithms analyze large datasets to identify patterns and make predictions. In radiology, these algorithms are used to detect anomalies in medical images, such as tumors or fractures. Techniques like support vector machines and random forests are commonly employed in these applications.
Deep Learning
Deep learning, a subset of machine learning, involves the use of artificial neural networks to model complex patterns in data. Convolutional neural networks (CNNs) are particularly effective in image analysis tasks. CNNs have been used to develop AI systems that can classify medical images with high accuracy, often surpassing human performance in specific tasks.
Natural Language Processing
NLP is used to analyze and interpret unstructured text data, such as radiology reports. AI systems equipped with NLP capabilities can extract relevant information from these reports, aiding in the diagnosis and treatment planning process.
Applications of AI in Radiology
AI has a wide range of applications in radiology, including image acquisition, image analysis, and workflow optimization.
Image Acquisition
AI can enhance image acquisition processes by optimizing imaging parameters and reducing scan times. For example, AI algorithms can adjust the settings of magnetic resonance imaging (MRI) machines in real-time to obtain clearer images with lower doses of radiation.
Image Analysis
AI systems are extensively used for image analysis, including detection, segmentation, and classification of abnormalities. These systems can identify subtle changes in medical images that may be missed by human radiologists. For instance, AI algorithms can detect early signs of breast cancer in mammograms with high sensitivity and specificity.
Workflow Optimization
AI can streamline radiology workflows by automating routine tasks, such as image sorting and report generation. This allows radiologists to focus on more complex cases, improving overall efficiency and reducing burnout.
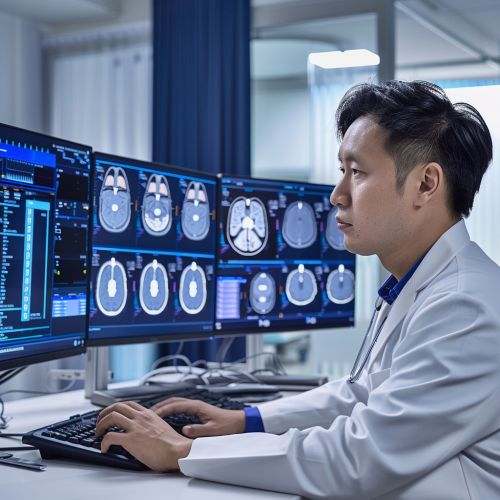
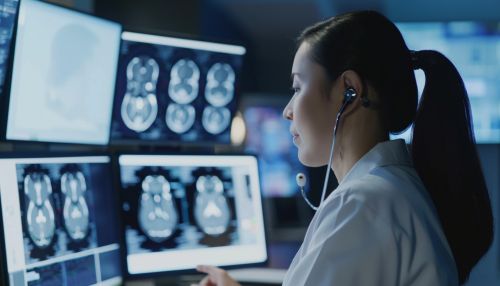
Ethical and Legal Considerations
The use of AI in radiology raises several ethical and legal issues, including data privacy, algorithmic bias, and accountability.
Data Privacy
AI systems require access to large amounts of medical data, raising concerns about patient privacy. Ensuring that data is anonymized and securely stored is crucial to maintaining patient confidentiality.
Algorithmic Bias
AI algorithms can exhibit bias if they are trained on unrepresentative datasets. This can lead to disparities in diagnostic accuracy across different patient populations. Efforts are being made to develop more inclusive datasets and to implement bias mitigation strategies.
Accountability
Determining accountability in cases where AI systems make diagnostic errors is a complex issue. Clear guidelines and regulations are needed to define the roles and responsibilities of AI developers, healthcare providers, and regulatory bodies.
Future Directions
The future of AI in radiology holds immense potential, with ongoing research focused on improving the accuracy, efficiency, and interpretability of AI systems.
Explainable AI
One of the key challenges in AI is the "black box" nature of deep learning models. Explainable AI aims to make these models more transparent, allowing radiologists to understand the reasoning behind AI-generated diagnoses.
Integration with Other Technologies
AI is expected to be increasingly integrated with other emerging technologies, such as augmented reality and telemedicine. This integration could further enhance diagnostic capabilities and improve access to radiological services in remote areas.
Personalized Medicine
AI has the potential to play a significant role in personalized medicine by analyzing individual patient data to tailor diagnostic and treatment plans. This approach could lead to more effective and targeted therapies.
Conclusion
AI is transforming the field of radiology by enhancing diagnostic accuracy, improving workflow efficiency, and enabling personalized medicine. While there are challenges to be addressed, the continued development and integration of AI technologies hold great promise for the future of radiology.