Geostatistics in Environmental Management: Difference between revisions
(Created page with "== Introduction == Geostatistics is a branch of statistics focusing on spatial or spatiotemporal datasets. It plays a crucial role in environmental management by providing tools and methods to analyze and interpret data that are spatially distributed. This field is particularly important for understanding and managing environmental phenomena such as pollution, resource distribution, and ecological patterns. Geostatistics enables the prediction and mapping of environment...") |
No edit summary |
||
Line 39: | Line 39: | ||
Geostatistics is also used in ecological modeling to study the distribution and abundance of species, habitat suitability, and biodiversity patterns. By analyzing spatial data, ecologists can identify critical habitats, assess the impacts of environmental changes, and develop conservation strategies. Geostatistical techniques are used to model species distributions and predict the effects of climate change on ecosystems. | Geostatistics is also used in ecological modeling to study the distribution and abundance of species, habitat suitability, and biodiversity patterns. By analyzing spatial data, ecologists can identify critical habitats, assess the impacts of environmental changes, and develop conservation strategies. Geostatistical techniques are used to model species distributions and predict the effects of climate change on ecosystems. | ||
[[Image:Detail-98597.jpg|thumb|center|Aerial view of a diverse forest landscape with varying tree densities and colors under a clear blue sky.|class=only_on_mobile]] | |||
[[Image:Detail-98598.jpg|thumb|center|Aerial view of a diverse forest landscape with varying tree densities and colors under a clear blue sky.|class=only_on_desktop]] | |||
== Advanced Geostatistical Techniques == | == Advanced Geostatistical Techniques == |
Latest revision as of 09:45, 19 October 2024
Introduction
Geostatistics is a branch of statistics focusing on spatial or spatiotemporal datasets. It plays a crucial role in environmental management by providing tools and methods to analyze and interpret data that are spatially distributed. This field is particularly important for understanding and managing environmental phenomena such as pollution, resource distribution, and ecological patterns. Geostatistics enables the prediction and mapping of environmental variables, which is essential for effective decision-making and policy formulation.
Fundamental Concepts of Geostatistics
Geostatistics is grounded in several key concepts, including spatial autocorrelation, variograms, kriging, and spatial interpolation.
Spatial Autocorrelation
Spatial autocorrelation refers to the degree to which a set of spatial data points are correlated with each other based on their locations. It is a critical concept in geostatistics because it helps in understanding how environmental variables are related across space. Positive spatial autocorrelation indicates that similar values occur near each other, while negative autocorrelation suggests that dissimilar values are spatially clustered.
Variograms
A variogram is a fundamental tool in geostatistics used to quantify spatial autocorrelation. It is a graph that represents the degree of spatial dependence of a spatial random field or stochastic process. The variogram function describes how the similarity between data points decreases as the distance between them increases. This function is essential for modeling and predicting spatial patterns in environmental data.
Kriging
Kriging is a geostatistical interpolation technique that provides the best linear unbiased prediction of the intermediate values. It is widely used in environmental management for creating continuous surface maps from discrete data points. Kriging takes into account both the distance and the degree of variation between known data points to estimate values at unknown locations.
Spatial Interpolation
Spatial interpolation involves estimating the values of environmental variables at unsampled locations based on the values at sampled locations. Techniques such as inverse distance weighting, spline interpolation, and kriging are commonly used in geostatistics for this purpose. These methods are crucial for creating detailed maps of environmental variables, which are essential for monitoring and managing natural resources.
Applications in Environmental Management
Geostatistics is applied in various aspects of environmental management, including pollution monitoring, resource assessment, and ecological modeling.
Pollution Monitoring
Geostatistics is essential for monitoring pollution levels in air, water, and soil. By analyzing spatial data, geostatisticians can identify pollution hotspots, track the spread of contaminants, and assess the effectiveness of remediation efforts. Techniques such as kriging are used to create detailed maps of pollutant concentrations, which are vital for regulatory compliance and public health protection.
Resource Assessment
In resource management, geostatistics is used to assess the distribution and quantity of natural resources such as minerals, water, and biomass. By analyzing spatial data, resource managers can make informed decisions about resource extraction, conservation, and sustainable use. Geostatistical models help in estimating the reserves of resources and predicting their spatial variability.
Ecological Modeling
Geostatistics is also used in ecological modeling to study the distribution and abundance of species, habitat suitability, and biodiversity patterns. By analyzing spatial data, ecologists can identify critical habitats, assess the impacts of environmental changes, and develop conservation strategies. Geostatistical techniques are used to model species distributions and predict the effects of climate change on ecosystems.
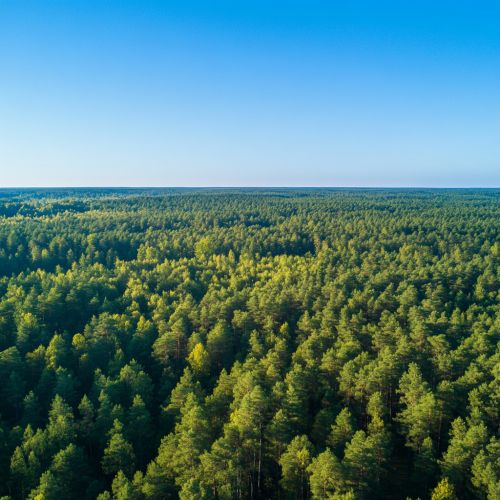
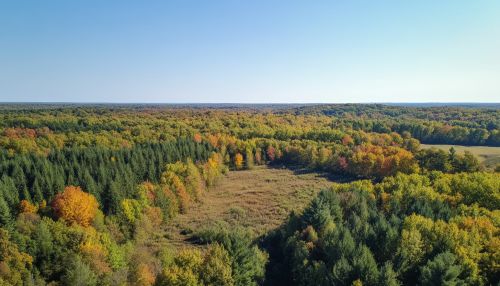
Advanced Geostatistical Techniques
In addition to basic methods, advanced geostatistical techniques are employed to address complex environmental management challenges.
Co-Kriging
Co-kriging is an extension of kriging that involves multiple correlated variables. It is used when there is more than one variable of interest, and these variables are spatially correlated. Co-kriging improves prediction accuracy by incorporating additional information from correlated variables, making it useful for complex environmental systems where multiple factors interact.
Indicator Kriging
Indicator kriging is a non-parametric geostatistical method used for categorical data. It is particularly useful for mapping the probability of occurrence of certain environmental conditions, such as the presence of a contaminant above a regulatory threshold. This technique helps in risk assessment and decision-making by providing probabilistic maps of environmental hazards.
Geostatistical Simulation
Geostatistical simulation involves generating multiple realizations of spatial data to assess uncertainty and variability. It is used in environmental management to evaluate the range of possible outcomes and the associated risks. Simulation techniques such as sequential Gaussian simulation and sequential indicator simulation are employed to model spatial uncertainty and support robust decision-making.
Challenges and Limitations
Despite its advantages, geostatistics faces several challenges and limitations in environmental management.
Data Quality and Availability
The accuracy of geostatistical analyses depends heavily on the quality and availability of spatial data. In many cases, environmental data may be sparse, incomplete, or of varying quality, which can affect the reliability of geostatistical models. Ensuring data quality and addressing data gaps are critical for effective geostatistical analysis.
Computational Complexity
Geostatistical methods can be computationally intensive, especially for large datasets and complex models. The computational demands of geostatistics may require specialized software and hardware, which can be a barrier for some organizations. Advances in computing technology and software development are helping to address these challenges.
Model Assumptions
Geostatistical models rely on certain assumptions, such as stationarity and isotropy, which may not always hold true in environmental systems. Violations of these assumptions can lead to biased or inaccurate results. It is important for practitioners to carefully evaluate model assumptions and consider alternative approaches when necessary.
Future Directions
The field of geostatistics is continually evolving, with new methods and applications emerging to address the growing complexity of environmental management.
Integration with Remote Sensing
The integration of geostatistics with remote sensing technologies is a promising area of development. Remote sensing provides high-resolution spatial data that can enhance geostatistical analyses and improve environmental monitoring. Combining geostatistics with remote sensing can lead to more accurate and timely assessments of environmental conditions.
Machine Learning and Geostatistics
The incorporation of machine learning techniques into geostatistics is another emerging trend. Machine learning algorithms can enhance geostatistical models by identifying complex patterns and relationships in spatial data. This integration has the potential to improve prediction accuracy and provide new insights into environmental processes.
Big Data and Geostatistics
The advent of big data presents both opportunities and challenges for geostatistics. The availability of large and diverse datasets can enhance geostatistical analyses, but also requires advanced data management and processing techniques. Developing methods to efficiently handle big data in geostatistics is an important area of research.
Conclusion
Geostatistics is a vital tool in environmental management, providing the means to analyze and interpret spatial data for informed decision-making. Despite challenges such as data quality and computational complexity, advances in technology and methodology continue to enhance the capabilities and applications of geostatistics. As the field evolves, it will play an increasingly important role in addressing environmental challenges and promoting sustainable management practices.