Turbulence modeling
Introduction
Turbulence modeling is a critical aspect of fluid dynamics, focusing on the representation and prediction of turbulent flows. Turbulence is characterized by chaotic changes in pressure and flow velocity, which makes its modeling complex and computationally intensive. Accurate turbulence models are essential for various engineering applications, including aerodynamics, meteorology, oceanography, and industrial processes.
Fundamentals of Turbulence
Turbulence is a fluid flow regime characterized by chaotic property changes. This includes low momentum diffusion, high momentum convection, and rapid variation of pressure and velocity in space and time. The Reynolds number is a dimensionless quantity that helps predict flow patterns in different fluid flow situations. High Reynolds numbers typically indicate turbulent flow.
Characteristics of Turbulent Flow
Turbulent flow exhibits several distinct characteristics:
- Irregularity: Turbulent flows are highly irregular and chaotic.
- Diffusivity: Turbulence enhances the mixing and transport of momentum, heat, and mass.
- Rotationality: Turbulent flows exhibit vorticity, a measure of the local rotation of the fluid.
- Dissipation: Turbulence involves the conversion of kinetic energy into thermal energy through viscous forces.
Turbulence Modeling Approaches
Turbulence modeling aims to develop mathematical models that can predict the behavior of turbulent flows. Several approaches exist, each with its strengths and limitations.
Direct Numerical Simulation (DNS)
DNS involves solving the Navier-Stokes equations directly for all relevant scales of motion. This approach provides the most accurate representation of turbulence but is computationally expensive and impractical for most real-world applications due to the high computational cost.
Large Eddy Simulation (LES)
LES resolves the larger scales of turbulence while modeling the smaller scales. This approach reduces computational cost compared to DNS while still capturing essential features of turbulence. LES is widely used in engineering applications where detailed flow information is necessary.
RANS models involve averaging the Navier-Stokes equations over time, resulting in equations that describe the mean flow properties. The effects of turbulence are modeled using additional terms known as Reynolds stresses. RANS models are computationally efficient and widely used in industrial applications.
Hybrid Models
Hybrid models combine elements of DNS, LES, and RANS to balance accuracy and computational cost. Examples include Detached Eddy Simulation (DES) and Partially-Averaged Navier-Stokes (PANS) models.
Common Turbulence Models
Several turbulence models are commonly used in practice, each with specific applications and limitations.
k-ε Model
The k-ε model is one of the most widely used RANS models. It involves two transport equations: one for the turbulent kinetic energy (k) and one for the rate of dissipation (ε). This model is robust and provides reasonable accuracy for many engineering applications.
k-ω Model
The k-ω model is another popular RANS model, involving two transport equations for the turbulent kinetic energy (k) and the specific dissipation rate (ω). This model performs well in near-wall regions and is often used in aerodynamic applications.
Spalart-Allmaras Model
The Spalart-Allmaras model is a single-equation RANS model that solves a transport equation for a modified form of the turbulent viscosity. It is computationally efficient and suitable for aerodynamic flows.
Reynolds Stress Model (RSM)
The RSM involves solving transport equations for the Reynolds stresses themselves, providing a more detailed representation of turbulence. This model is more accurate but also more computationally intensive than simpler models like k-ε and k-ω.
Applications of Turbulence Modeling
Turbulence modeling is essential in various fields, including:
Aerodynamics
In aerodynamics, turbulence modeling is used to predict the behavior of airflow over aircraft surfaces, which is crucial for designing efficient and safe aircraft.
Meteorology
Meteorologists use turbulence models to understand and predict weather patterns, including the formation of clouds and the dispersion of pollutants.
Oceanography
Turbulence modeling helps oceanographers study ocean currents, wave dynamics, and the mixing of nutrients and pollutants in the ocean.
Industrial Processes
In industrial processes, turbulence modeling is used to optimize the design of equipment such as reactors, heat exchangers, and combustion systems.
Challenges in Turbulence Modeling
Despite significant advancements, turbulence modeling remains challenging due to the complexity of turbulent flows. Some of the key challenges include:
Scale Separation
Turbulent flows involve a wide range of scales, from large energy-containing eddies to small dissipative scales. Accurately capturing all these scales is computationally demanding.
Model Validation
Validating turbulence models requires high-quality experimental or DNS data, which can be difficult and expensive to obtain.
Uncertainty Quantification
Turbulence models involve various assumptions and approximations, leading to uncertainties in the predictions. Quantifying and reducing these uncertainties is an ongoing area of research.
Future Directions in Turbulence Modeling
Advancements in computational power and numerical methods continue to drive progress in turbulence modeling. Some promising future directions include:
Machine Learning
Machine learning techniques are being explored to develop data-driven turbulence models that can improve accuracy and reduce computational cost.
High-Performance Computing
The use of high-performance computing (HPC) enables more detailed simulations, bringing DNS and LES within reach for more applications.
Multiscale Modeling
Multiscale modeling approaches aim to bridge the gap between different scales of turbulence, providing a more comprehensive representation of turbulent flows.
Conclusion
Turbulence modeling is a vital aspect of fluid dynamics, with applications across various fields. While significant progress has been made, challenges remain in accurately and efficiently predicting turbulent flows. Continued research and advancements in computational methods hold promise for further improving turbulence models.
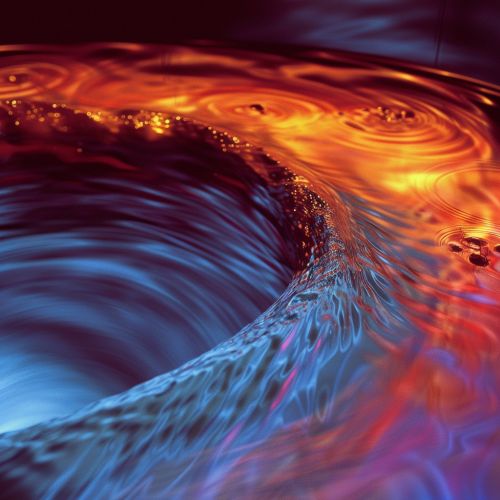
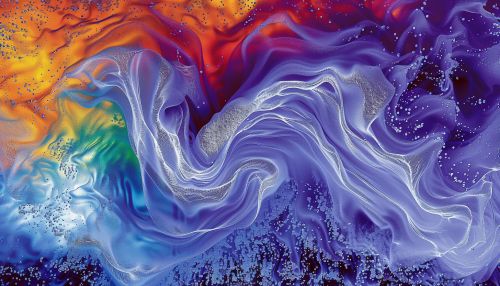