The Role of Quantum Computing in Materials Science
Introduction
Quantum computing is a rapidly evolving field that harnesses the principles of quantum mechanics to process information. The fundamental unit of computation in a quantum computer, known as a qubit, can exist in multiple states simultaneously, a property known as superposition. This, along with the property of entanglement, allows quantum computers to process vast amounts of data at once, making them potentially far more powerful than classical computers for certain tasks. One of the areas where quantum computing is expected to make a significant impact is in materials science.
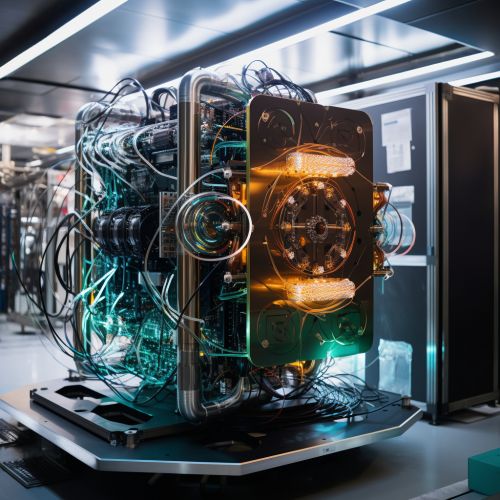
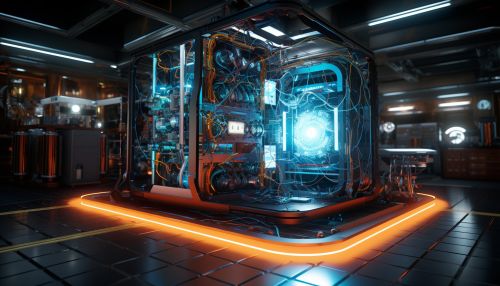
Quantum Computing and Materials Science
Materials science is an interdisciplinary field that studies the properties of matter and its applications to various areas of science and engineering. It involves the design and discovery of new materials, particularly solids. The traditional approach to materials discovery involves trial and error, or serendipity, but this is a slow and laborious process. Quantum computing, with its ability to process and analyze large amounts of data quickly and accurately, has the potential to revolutionize this process.
Quantum Simulations
One of the key applications of quantum computing in materials science is in the area of quantum simulations. These simulations can provide a detailed understanding of the quantum mechanical phenomena that govern the properties of materials. This is particularly important for materials that exhibit exotic quantum mechanical effects, such as high-temperature superconductors and topological insulators. Quantum simulations can also be used to predict the properties of new materials before they are synthesized in the lab, potentially saving a significant amount of time and resources.
Quantum Machine Learning
Another promising application of quantum computing in materials science is in the area of quantum machine learning. Machine learning involves the use of algorithms that can learn from and make predictions or decisions based on data. Quantum machine learning algorithms can process large amounts of data more efficiently than classical algorithms, making them particularly useful for tasks such as materials discovery and design. For example, they can be used to identify patterns in the properties of materials that would be difficult to detect using classical methods.
Challenges and Future Directions
Despite the significant potential of quantum computing in materials science, there are several challenges that need to be overcome. One of the key challenges is the development of reliable and scalable quantum computers. While significant progress has been made in this area, current quantum computers are still relatively small and prone to errors. Another challenge is the development of efficient quantum algorithms for materials science applications.
Looking ahead, the field of quantum computing in materials science is expected to continue to grow and evolve. As quantum computers become more powerful and reliable, they are likely to play an increasingly important role in materials discovery and design. At the same time, the development of new quantum algorithms and machine learning techniques will continue to expand the range of applications of quantum computing in materials science.