The Role of Quantum Computing in Financial Modeling
Introduction
Quantum computing is a rapidly evolving field that leverages the principles of quantum mechanics to perform computations. Unlike classical computers, which use bits as their most basic unit of information, quantum computers use quantum bits or qubits. This fundamental difference allows quantum computers to solve certain types of problems much more efficiently than classical computers.
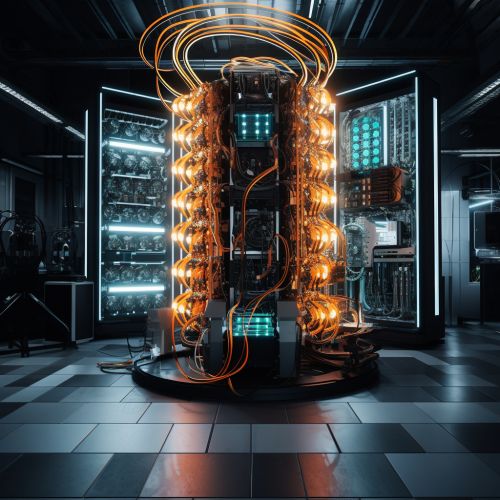
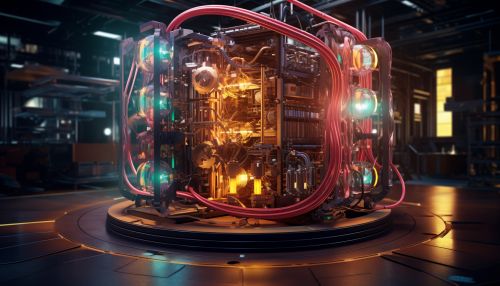
Quantum Computing and Financial Modeling
Financial modeling is a critical component of modern finance. It involves the creation of abstract representations (or models) of real-world financial situations. These models are typically built using mathematical formulas and are used for a variety of purposes, such as valuing financial instruments, assessing risk, and making investment decisions. Quantum computing has the potential to revolutionize financial modeling by providing solutions to problems that are currently intractable for classical computers.
Quantum Algorithms in Financial Modeling
One of the ways in which quantum computing can be applied to financial modeling is through the use of quantum algorithms. These are algorithms that are designed to run on quantum computers and take advantage of their unique properties. For example, the Quantum Fourier Transform (QFT) is a quantum algorithm that can be used to calculate the discrete Fourier transform of a sequence of qubits. This is a critical operation in many financial modeling tasks, such as pricing derivative securities.
Another quantum algorithm that has potential applications in financial modeling is the Quantum Amplitude Estimation (QAE). This algorithm can be used to estimate the expected value of a random variable, which is a common task in financial modeling. The QAE algorithm has been shown to provide a quadratic speedup over classical algorithms for this task, which could lead to significant improvements in the efficiency of financial models.
Quantum Machine Learning in Financial Modeling
Quantum machine learning is another area where quantum computing could have a significant impact on financial modeling. Machine learning involves the use of algorithms to identify patterns in data and make predictions or decisions without being explicitly programmed to do so. Quantum machine learning leverages the principles of quantum mechanics to improve the efficiency and accuracy of these algorithms.
For example, quantum support vector machines (QSVMs) are a type of quantum machine learning algorithm that can be used for classification and regression tasks. These tasks are common in financial modeling, where they are used to predict future prices or identify patterns in financial data. QSVMs have been shown to provide a speedup over classical support vector machines, which could lead to more accurate and efficient financial models.
Challenges and Future Directions
Despite the potential benefits of quantum computing in financial modeling, there are several challenges that must be overcome before these benefits can be fully realized. One of the main challenges is the current lack of large-scale, error-corrected quantum computers. Most of the quantum computers that exist today are small and prone to errors, which limits their practical usefulness.
Another challenge is the lack of quantum algorithms for many financial modeling tasks. While there are quantum algorithms for some tasks, such as the QFT and QAE, there are many other tasks for which no quantum algorithms currently exist. Developing these algorithms will be a critical step towards the widespread use of quantum computing in financial modeling.
Despite these challenges, the future of quantum computing in financial modeling looks promising. As quantum computers become more powerful and reliable, and as more quantum algorithms are developed, it is likely that the use of quantum computing in financial modeling will become increasingly common. This could lead to significant improvements in the accuracy and efficiency of financial models, which could have far-reaching implications for the world of finance.