The Role of Quantum Computing in Artificial Intelligence
Introduction
Quantum computing is a rapidly evolving field that leverages the principles of quantum mechanics to perform computational tasks. It represents a significant departure from classical computing, which relies on bits to process information. Quantum computers use quantum bits, or qubits, which can exist in multiple states at once due to the phenomenon of superposition. This ability, along with other quantum phenomena such as entanglement and quantum tunneling, allows quantum computers to process vast amounts of data simultaneously, potentially solving complex problems much faster than classical computers.
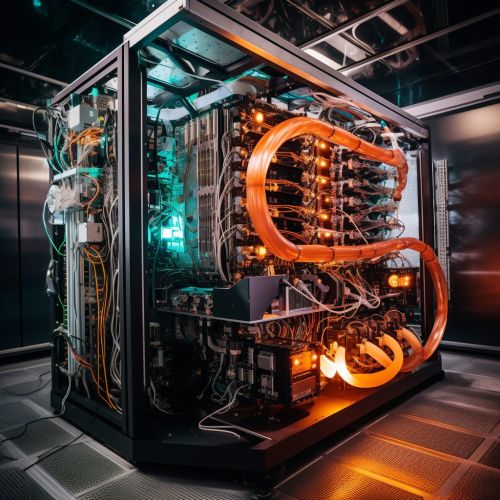
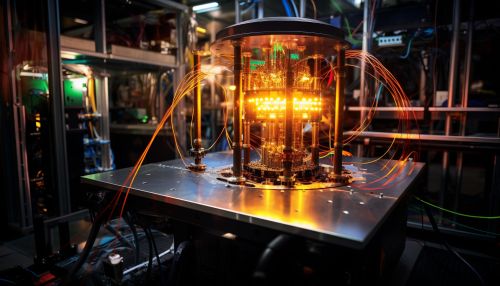
Quantum Computing and Artificial Intelligence
Artificial Intelligence (AI) is a field that seeks to create machines capable of intelligent behavior. It encompasses various subfields, including machine learning, where computers learn from data, and deep learning, a subset of machine learning that structures algorithms in layers to create an "artificial neural network" that can learn and make intelligent decisions on its own. Quantum computing's potential to process large amounts of data quickly and efficiently could significantly impact these areas of AI.
Quantum Machine Learning
Quantum machine learning is a subfield of quantum computing and machine learning. It uses quantum algorithms to improve the efficiency and speed of machine learning tasks. Quantum machine learning algorithms can potentially process vast amounts of data faster than classical algorithms, making them particularly suitable for handling big data. Quantum machine learning could also lead to advancements in deep learning, as quantum algorithms could potentially handle the complex computations involved in artificial neural networks more efficiently.
Quantum Optimization
Quantum optimization is another area where quantum computing could significantly impact AI. Optimization problems, which involve finding the best solution from a set of possible solutions, are common in AI. For example, in machine learning, optimization algorithms are used to adjust the parameters of a model to improve its performance. Quantum optimization algorithms could potentially solve these problems more efficiently than classical algorithms, leading to more effective AI models.
Quantum Neural Networks
Quantum neural networks are a type of artificial neural network that uses quantum theory's principles. They are still largely theoretical but could potentially offer significant advantages over classical neural networks. For example, they could potentially process information more efficiently, due to quantum superposition and entanglement, leading to more powerful AI models.
Potential Applications of Quantum Computing in AI
The potential applications of quantum computing in AI are vast and varied. They range from improving machine learning algorithms to enabling more complex AI models.
Improved Machine Learning Algorithms
As mentioned earlier, quantum machine learning algorithms could potentially process large amounts of data faster than classical algorithms. This could lead to significant improvements in machine learning tasks, such as classification, regression, and clustering.
More Complex AI Models
Quantum computing could also enable more complex AI models. For example, it could potentially make it feasible to train larger and deeper neural networks, leading to more powerful AI systems.
Quantum-Assisted AI
Quantum-assisted AI is a concept where quantum computing is used to assist AI, rather than replace it. For example, quantum computers could be used to process large amounts of data quickly, while classical computers handle tasks that they are better suited for. This could lead to more efficient and effective AI systems.
Challenges and Future Directions
Despite the potential benefits, there are several challenges to integrating quantum computing with AI. These include the current lack of large-scale quantum computers, the difficulty of developing quantum algorithms, and the need for more research in quantum machine learning and quantum neural networks.
However, the field is rapidly advancing, and these challenges are likely to be overcome in the future. As quantum computing technology continues to improve, it is expected to play an increasingly important role in AI.