The Role of Machine Learning in Climate Forecasting
Introduction
Machine learning is a branch of artificial intelligence that involves the use of algorithms and statistical models to enable computers to perform tasks without explicit programming. In recent years, machine learning has found applications in a wide range of fields, including climate forecasting. This article explores the role of machine learning in climate forecasting, detailing the methods used, the benefits and challenges, and future prospects.
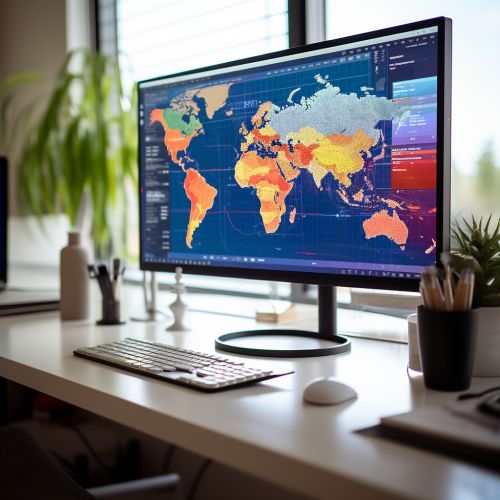
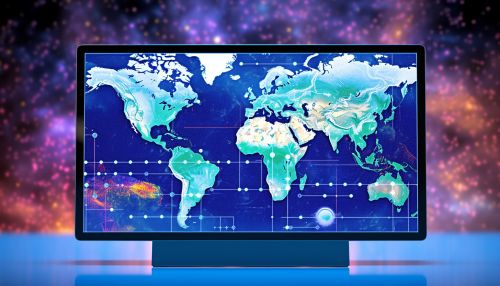
Machine Learning in Climate Science
Climate science involves the study of the climate system, which includes the atmosphere, hydrosphere, cryosphere, land surface, and biosphere. It is a complex field that requires the analysis of vast amounts of data to make accurate predictions. Machine learning, with its ability to analyze large datasets and identify patterns, has become an invaluable tool in this field.
Climate Models and Machine Learning
Traditional climate models are based on physical laws and mathematical equations. These models, while effective, are computationally intensive and require significant resources. Machine learning offers an alternative approach. Instead of relying on physical laws, machine learning algorithms learn from data. They can identify patterns and make predictions based on these patterns.
Machine learning algorithms used in climate science include supervised learning, unsupervised learning, and reinforcement learning. Supervised learning involves training an algorithm using labeled data. The algorithm learns to predict the output from the input data. In unsupervised learning, the algorithm learns from unlabeled data by identifying patterns and structures. Reinforcement learning involves an agent that learns to make decisions by interacting with its environment.
Benefits of Machine Learning in Climate Forecasting
Machine learning offers several benefits in climate forecasting. Firstly, it can handle large datasets, which are common in climate science. Secondly, machine learning algorithms can identify complex patterns that may be missed by traditional models. This can lead to more accurate predictions. Thirdly, machine learning can automate the process of climate forecasting, reducing the need for human intervention.
Challenges and Limitations
Despite its benefits, the use of machine learning in climate forecasting also presents several challenges. One of the main challenges is the quality and availability of data. Machine learning algorithms require large amounts of high-quality data to function effectively. However, obtaining such data can be difficult, especially for regions with limited monitoring infrastructure.
Another challenge is the interpretability of machine learning models. These models can be seen as "black boxes", making it difficult to understand how they make their predictions. This lack of transparency can be a problem, especially in a field like climate science where understanding the underlying processes is important.
Future Prospects
The use of machine learning in climate forecasting is still a relatively new field, and there is much potential for future development. One area of interest is the integration of machine learning with traditional climate models. This could potentially combine the strengths of both approaches, leading to more accurate and efficient forecasts.
Another area of interest is the use of machine learning in climate change mitigation and adaptation strategies. Machine learning algorithms could be used to predict the impacts of climate change and to identify the most effective strategies for mitigation and adaptation.
See Also
- Artificial Intelligence in Environmental Science
- Data Analysis in Climate Science
- Predictive Modeling in Climate Science