The Role of Deep Learning in Genomic Data Analysis
Introduction
Deep learning, a subfield of artificial intelligence, has revolutionized many areas of research and industry, including genomics. Genomic data analysis, the process of interpreting and understanding the vast amounts of data generated by genomic sequencing technologies, has greatly benefited from the application of deep learning techniques. This article will delve into the role of deep learning in genomic data analysis, discussing its applications, challenges, and future directions.

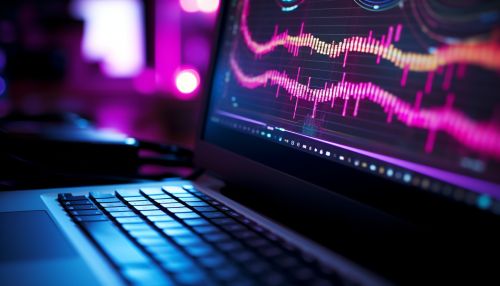
Deep Learning: A Brief Overview
Deep learning is a machine learning technique that teaches computers to do what comes naturally to humans: learn by example. Deep learning is a key technology behind driverless cars, enabling them to recognize a stop sign or to distinguish a pedestrian from a lamppost. It is the key to voice control in consumer devices like phones, tablets, TVs, and hands-free speakers.
Deep learning models are built using neural networks that consist of several layers. The 'deep' in deep learning refers to the number of layers through which the data is transformed. Deep learning models can have tens or even hundreds of layers that are interconnected in a complex manner.
Genomic Data Analysis: The Basics
Genomics is the study of the complete set of genes within an organism (the genome), including the interactions of those genes with each other and with the organism's environment. Genomic data analysis involves the use of computational and statistical techniques to decipher the biological information contained in genomic data.
Genomic data analysis is a complex task due to the sheer size and complexity of genomic data. The human genome, for instance, contains approximately 3 billion base pairs, and each individual's genome is unique. This makes the analysis and interpretation of genomic data a challenging task.
Deep Learning in Genomic Data Analysis
Deep learning has been increasingly applied in genomic data analysis due to its ability to model complex patterns and its capacity to handle large amounts of data. The following sections will discuss some of the key applications of deep learning in genomic data analysis.
Sequence Analysis
One of the primary applications of deep learning in genomics is in sequence analysis. Deep learning models can be trained to recognize patterns in genomic sequences that are indicative of certain genetic traits or diseases. For example, deep learning models have been used to predict the effects of genetic variants on disease risk, to identify regions of the genome that are involved in gene regulation, and to predict the 3D structure of DNA and proteins from sequence data.
Gene Expression Analysis
Deep learning can also be used to analyze gene expression data. Gene expression is the process by which the information contained in a gene is used to create a functional product, usually a protein. Deep learning models can be trained to predict gene expression levels based on genomic and epigenomic data, which can help in understanding the regulatory mechanisms that control gene expression.
Disease Prediction
Deep learning models can be used to predict disease risk based on genomic data. For example, deep learning models have been used to predict the risk of developing diseases such as cancer, Alzheimer's disease, and cardiovascular disease based on an individual's genomic data. These models can potentially be used in personalized medicine to identify individuals at high risk of developing certain diseases.
Challenges and Future Directions
Despite the promise of deep learning in genomic data analysis, there are several challenges that need to be addressed. One of the main challenges is the interpretability of deep learning models. Unlike traditional statistical models, deep learning models are often described as "black boxes" because it is difficult to understand how they make their predictions.
Another challenge is the need for large amounts of annotated data for training deep learning models. While genomic data is abundant, annotated genomic data (i.e., genomic data that has been labeled with relevant biological information) is relatively scarce.
Looking ahead, the field of deep learning in genomic data analysis is likely to continue to grow and evolve. As more annotated genomic data becomes available and as new deep learning techniques are developed, it is likely that the use of deep learning in genomic data analysis will become increasingly sophisticated and widespread.
See Also
- Artificial Intelligence in Healthcare
- Bioinformatics
- Computational Biology
- Machine Learning in Bioinformatics