The Role of Data Mining in Healthcare Analytics
Introduction
Data mining, a process that involves the discovery of patterns and knowledge from large amounts of data, plays a crucial role in healthcare analytics. It is a multidisciplinary field that combines artificial intelligence, machine learning, statistics, and database systems. The goal of data mining in healthcare analytics is to extract valuable information from vast amounts of medical data, which can then be used to improve decision making, patient care, and outcomes.
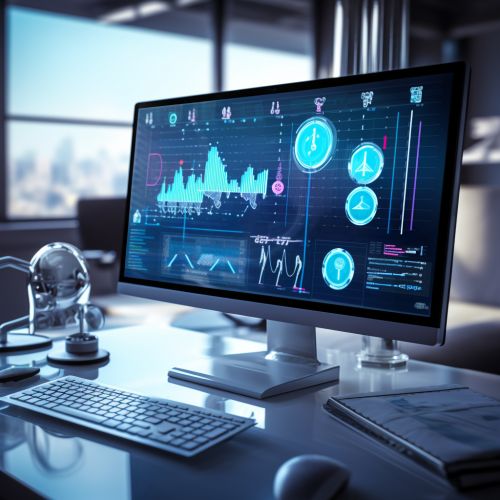
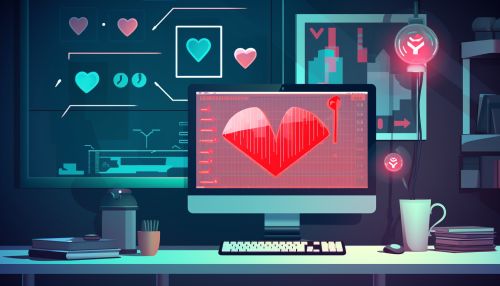
Data Mining Techniques in Healthcare
Various data mining techniques are employed in healthcare analytics. These include:
- Classification: This technique is used to classify data into different categories. In healthcare, it can be used to predict disease risk or to classify patients based on their symptoms or conditions.
- Clustering: Clustering is used to group similar data together. It can be used to identify groups of patients with similar health conditions or symptoms.
- Association Rules: This technique is used to discover associations between different data items. In healthcare, it can be used to identify associations between different diseases or between a disease and its potential risk factors.
- Regression: Regression is used to predict a continuous outcome variable from one or more predictor variables. In healthcare, it can be used to predict patient outcomes based on various factors such as age, gender, and medical history.
- Sequential Pattern Mining: This technique is used to discover patterns in sequential data. In healthcare, it can be used to identify patterns in patient treatment sequences.
Applications of Data Mining in Healthcare
Data mining has numerous applications in healthcare, some of which include:
- Disease Prediction and Diagnosis: Data mining techniques can be used to predict the likelihood of a patient developing a certain disease based on their medical history and other factors. They can also be used to diagnose diseases by analyzing patient symptoms and medical test results.
- Patient Segmentation: Data mining can be used to segment patients into different groups based on their health conditions, symptoms, or risk factors. This can help healthcare providers to provide personalized care and treatment to each patient group.
- Drug Discovery: Data mining can be used to discover new drugs by analyzing the chemical structures of existing drugs and their effects on different diseases.
- Healthcare Management: Data mining can be used to improve healthcare management by analyzing patient flow, resource utilization, and other operational data.
Challenges in Data Mining for Healthcare
Despite its potential benefits, data mining in healthcare faces several challenges. These include:
- Data Quality and Preprocessing: Healthcare data is often incomplete, noisy, and inconsistent. Data preprocessing, which involves cleaning and transforming raw data into a suitable format for data mining, is therefore a crucial step in the data mining process.
- Privacy and Security: Protecting patient privacy and data security is a major concern in healthcare data mining. Healthcare organizations must ensure that they comply with all relevant laws and regulations, such as the Health Insurance Portability and Accountability Act (HIPAA) in the United States.
- Interpretability: The results of data mining must be interpretable to healthcare professionals. This requires the development of data mining models that are not only accurate, but also understandable and explainable.
Future Directions
The future of data mining in healthcare looks promising, with several emerging trends:
- Big Data Analytics: The advent of big data in healthcare, which includes electronic health records (EHRs), genomic data, and wearable device data, presents new opportunities for data mining. Big data analytics can enable the analysis of large, complex, and diverse datasets, leading to more accurate and comprehensive insights.
- Artificial Intelligence and Machine Learning: AI and machine learning techniques, such as deep learning, are increasingly being used in healthcare data mining. These techniques can learn complex patterns from data and make accurate predictions, making them particularly useful for tasks such as disease prediction and diagnosis.
- Real-Time Analytics: Real-time analytics, which involves analyzing data as it is generated, can enable healthcare providers to make timely decisions and interventions. This can be particularly useful in critical care settings, where timely decision making can save lives.