The Role of Computer Vision in Wildlife Monitoring and Conservation
Introduction
Computer vision is a field of artificial intelligence (AI) that enables computers to interpret and understand the visual world. In the context of wildlife monitoring and conservation, computer vision technologies are increasingly being used to automate the process of identifying and tracking animals in their natural habitats. This article explores the role of computer vision in wildlife monitoring and conservation, discussing its applications, benefits, challenges, and future potential.
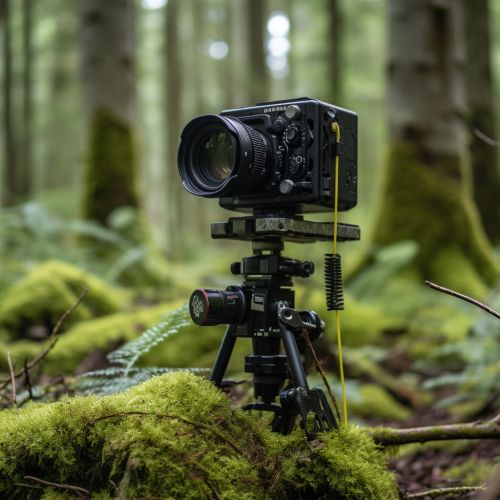
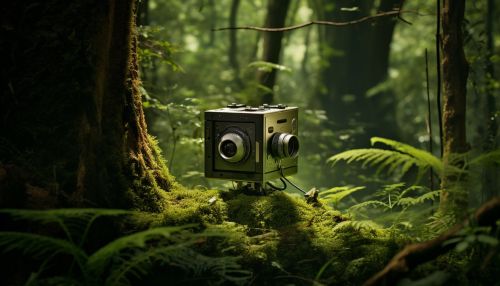
The Concept of Computer Vision
Computer Vision is a branch of artificial intelligence that trains computers to interpret and understand the visual world. By using digital images from cameras and videos and deep learning models, machines can accurately identify and classify objects, and then react to what they "see."
Applications of Computer Vision in Wildlife Monitoring and Conservation
Computer vision has a wide range of applications in wildlife monitoring and conservation. These include species identification, population estimation, behavior analysis, and habitat assessment.
Species Identification
One of the primary applications of computer vision in wildlife conservation is species identification. Traditionally, this task has been performed manually by trained experts, which is time-consuming and prone to errors. Computer vision algorithms, particularly those based on deep learning, can automate this process, providing more accurate and efficient identification of different species.
Population Estimation
Computer vision can also be used for population estimation. By analyzing images or video footage captured by wildlife cameras, computer vision algorithms can count the number of individuals in a species and track their movements over time. This information is crucial for monitoring population trends and implementing effective conservation strategies.
Behavior Analysis
Computer vision can be used to analyze animal behavior. By tracking the movements of animals and analyzing their interactions, computer vision algorithms can provide insights into animal behavior that can be used to inform conservation efforts.
Habitat Assessment
Computer vision can also be used for habitat assessment. By analyzing images of an animal's habitat, computer vision algorithms can identify changes in the environment that may impact wildlife, such as deforestation or urban development.
Benefits of Using Computer Vision in Wildlife Monitoring and Conservation
The use of computer vision in wildlife monitoring and conservation offers several benefits. These include increased efficiency, improved accuracy, and the ability to monitor wildlife in remote or inaccessible areas.
Increased Efficiency
One of the main benefits of using computer vision in wildlife monitoring and conservation is increased efficiency. Manual identification and counting of animals is a time-consuming process that requires significant human resources. Computer vision algorithms can automate these tasks, freeing up conservationists to focus on other aspects of their work.
Improved Accuracy
Computer vision algorithms can also provide more accurate identification and counting of animals than manual methods. This is particularly important in the context of wildlife conservation, where accurate data is crucial for monitoring population trends and implementing effective conservation strategies.
Remote Monitoring
Computer vision technologies also enable remote monitoring of wildlife. This is particularly useful in areas that are difficult to access or where human presence may disturb the animals.
Challenges and Limitations of Using Computer Vision in Wildlife Monitoring and Conservation
Despite its potential, the use of computer vision in wildlife monitoring and conservation also presents several challenges. These include issues related to data quality, algorithmic bias, and the need for specialized expertise.
Data Quality
The effectiveness of computer vision algorithms depends on the quality of the data they are trained on. In the context of wildlife monitoring, this data typically comes from wildlife cameras, which can vary in terms of their image quality and the conditions under which they are able to capture images.
Algorithmic Bias
Like all machine learning algorithms, computer vision algorithms are susceptible to bias. This can occur when the data used to train the algorithms is not representative of the diversity of species and habitats that the algorithms will be used to analyze.
Need for Specialized Expertise
The use of computer vision in wildlife monitoring and conservation requires specialized expertise in both ecology and computer science. This can be a barrier to the adoption of these technologies, particularly in resource-limited settings.
Future Potential of Computer Vision in Wildlife Monitoring and Conservation
Despite these challenges, the future potential of computer vision in wildlife monitoring and conservation is significant. Advances in AI and machine learning are continually improving the accuracy and efficiency of computer vision algorithms. At the same time, the increasing availability of high-quality wildlife cameras and the growing interest in citizen science are expanding the amount of data available for training these algorithms.