The Role of Computer Vision in Precision Agriculture
Introduction
Computer vision is a subfield of artificial intelligence (AI) that trains computers to interpret and understand the visual world. By using digital images from cameras and videos and deep learning models, machines can accurately identify and classify objects — and then react to what they “see.” In the context of precision agriculture, computer vision is used to increase efficiency and productivity while minimizing costs and manual labor.
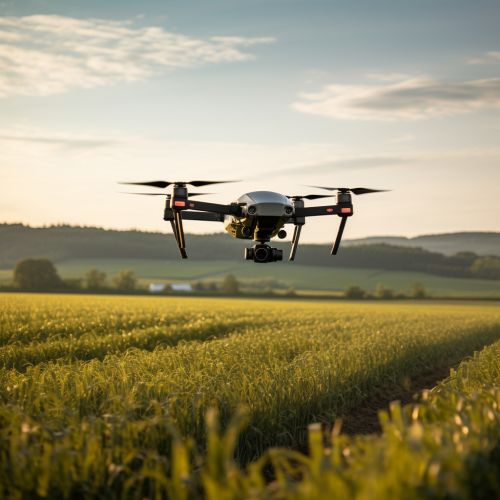
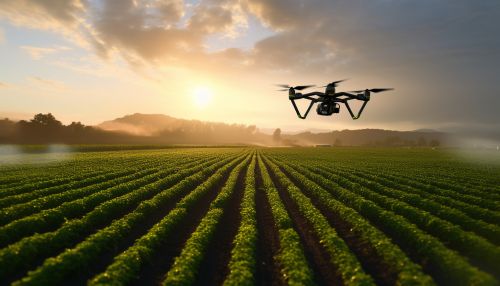
Role of Computer Vision in Precision Agriculture
Precision agriculture is a farming management concept based on observing, measuring, and responding to inter and intra-field variability in crops. The role of computer vision in precision agriculture is to provide accurate and timely information about the field conditions.
Crop Monitoring
One of the primary uses of computer vision in precision agriculture is for crop monitoring. Using images captured by drones, satellites, or other aerial imaging methods, computer vision algorithms can analyze the health and condition of crops. This can include identifying areas of stress, disease, or pest infestation, which allows farmers to take targeted action to address these issues.
Yield Estimation
Computer vision can also be used for yield estimation. By analyzing images of crops at various stages of growth, computer vision algorithms can predict the likely yield of a crop. This can help farmers plan for harvest and market their crops more effectively.
Weed Detection
Weed detection is another important application of computer vision in precision agriculture. By analyzing images of a field, computer vision algorithms can identify weeds and distinguish them from crops. This allows for targeted weed control, which can reduce the amount of herbicides used and increase crop yields.
Soil Analysis
Computer vision can also be used for soil analysis. By analyzing images of the soil, computer vision algorithms can determine the soil type, moisture content, and nutrient levels. This information can be used to optimize irrigation and fertilization, leading to more efficient use of resources and higher crop yields.
Challenges and Future Directions
While computer vision has the potential to greatly improve the efficiency and productivity of precision agriculture, there are also challenges to its implementation. These include the high cost of equipment and software, the need for technical expertise to use and maintain the systems, and the variability of outdoor lighting conditions, which can affect the accuracy of image analysis.
Despite these challenges, the future of computer vision in precision agriculture looks promising. Advances in AI and machine learning are leading to more accurate and efficient algorithms, and the cost of equipment is decreasing. Furthermore, the growing awareness of the environmental impact of agriculture is driving the adoption of more sustainable practices, such as precision agriculture.