The Role of Computer Vision in Automated Quality Control
Introduction
Computer vision is a field of artificial intelligence (AI) that trains computers to interpret and understand the visual world. By using digital images from cameras and videos and deep learning models, machines can accurately identify and classify objects — and then react to what they “see.”
In the context of automated quality control, computer vision plays a pivotal role. It allows for the automated inspection of products, components, and manufacturing processes, replacing manual inspection in many scenarios. This article will delve into the role of computer vision in automated quality control, discussing its applications, benefits, and challenges.
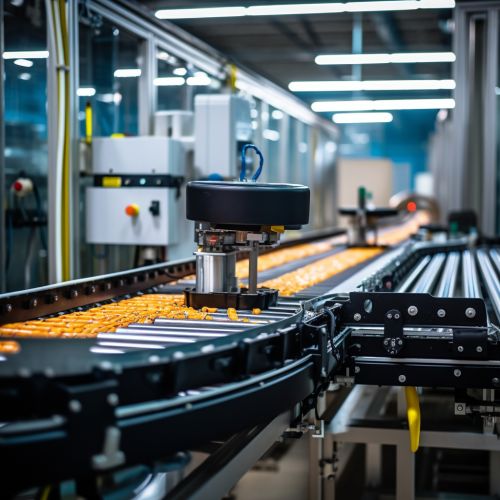

Applications of Computer Vision in Automated Quality Control
Computer vision has a wide range of applications in automated quality control, from manufacturing to food production and beyond.
Manufacturing
In manufacturing, computer vision is used for automated optical inspection (AOI). AOI is a key process used in the manufacturing and testing of electronics assemblies, particularly printed circuit board assemblies (PCBAs). Computer vision systems can inspect PCBAs for defects such as shorts, opens, insufficient solder, incorrect components, and missing components, replacing manual inspection to improve speed and accuracy.
Food Production
In food production, computer vision can be used to inspect the quality of products on a production line. For example, computer vision systems can inspect fruits and vegetables for defects, sort them based on their size or color, and even determine whether they are ripe or not. This allows for faster and more accurate quality control, reducing waste and improving productivity.
Pharmaceutical Industry
In the pharmaceutical industry, computer vision is used for pill identification and verification. Computer vision systems can identify pills based on their color, shape, and imprint, ensuring that the correct medication is dispensed. This reduces the risk of medication errors, improving patient safety.
Benefits of Using Computer Vision in Automated Quality Control
There are several benefits to using computer vision in automated quality control.
Increased Accuracy
One of the main benefits of using computer vision for automated quality control is increased accuracy. Computer vision systems can identify and classify objects with a high degree of accuracy, reducing the risk of errors. This is particularly important in industries where errors can have serious consequences, such as the pharmaceutical industry.
Improved Efficiency
Computer vision can also improve efficiency. By automating the quality control process, companies can inspect more products in less time, increasing productivity. This is particularly beneficial in industries with high production volumes, such as manufacturing and food production.
Reduced Costs
By increasing accuracy and efficiency, computer vision can help to reduce costs. Fewer errors mean less waste and fewer recalls, saving companies money. Additionally, by automating the quality control process, companies can reduce labor costs.
Challenges of Using Computer Vision in Automated Quality Control
While there are many benefits to using computer vision in automated quality control, there are also some challenges.
Technical Challenges
One of the main challenges is the technical complexity of computer vision systems. Developing and implementing a computer vision system requires a high level of expertise in AI and machine learning, as well as a deep understanding of the specific quality control requirements of the industry.
Data Privacy Concerns
Another challenge is data privacy. Computer vision systems often require access to large amounts of data, which can raise privacy concerns. Companies must ensure that they comply with all relevant data protection regulations when using computer vision for automated quality control.
Dependence on Quality Data
Finally, the effectiveness of computer vision systems depends on the quality of the data they are trained on. If the training data is not representative of the real-world scenarios the system will encounter, the system may not perform as expected.
Conclusion
Computer vision plays a crucial role in automated quality control, with applications in a wide range of industries. By increasing accuracy and efficiency, and reducing costs, computer vision can significantly improve the quality control process. However, there are also challenges to consider, including technical complexity, data privacy concerns, and dependence on quality data. Despite these challenges, the potential benefits of computer vision in automated quality control make it a promising area for further research and development.