The Role of Bioinformatics in Microbiome Analysis
Introduction
Bioinformatics is a multidisciplinary field that combines biology, computer science, information engineering, mathematics, and statistics to analyze and interpret biological data. One of the most significant applications of bioinformatics is in the analysis of microbiomes, complex communities of microorganisms that inhabit various environments, including the human body.
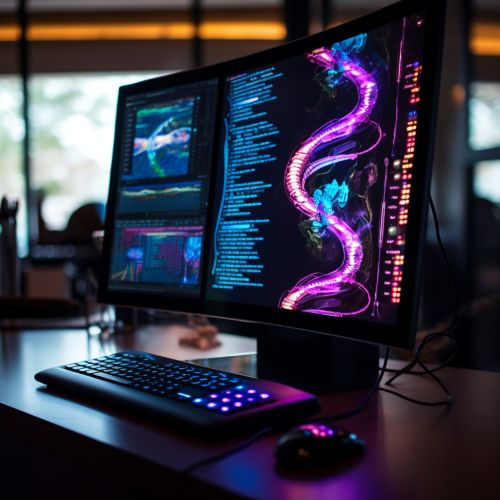
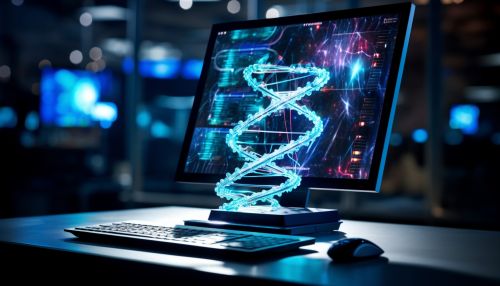
Bioinformatics and Microbiome Analysis
Bioinformatics plays a crucial role in microbiome analysis, as it provides the necessary tools and methodologies to process, analyze, and interpret the vast amounts of data generated by microbiome studies. This includes the use of genomic, metagenomic, and proteomic data to understand the composition, function, and dynamics of microbiomes.
Microbiome Data Generation
Microbiome data is primarily generated through two methods: 16S rRNA gene sequencing and metagenomic sequencing. 16S rRNA gene sequencing is used to identify and classify bacteria present in a sample based on the 16S rRNA gene, while metagenomic sequencing provides a more comprehensive view of the microbiome by sequencing all the genetic material present in a sample.
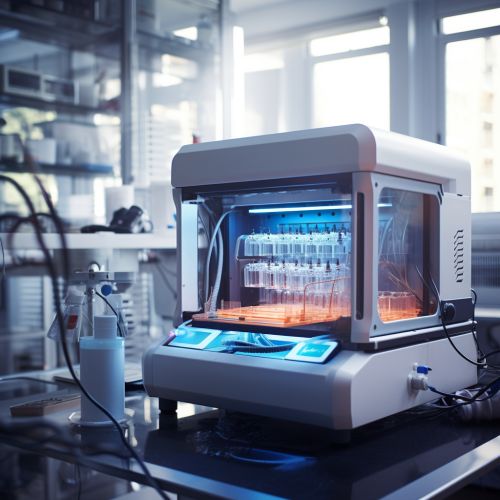
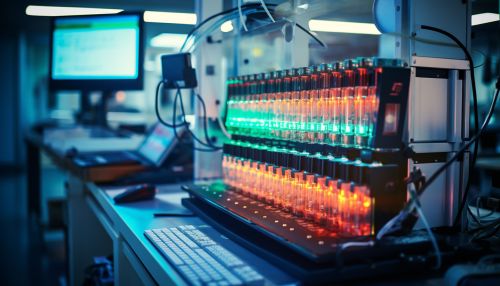
Bioinformatics Tools for Microbiome Analysis
There are numerous bioinformatics tools available for microbiome analysis. These tools can be broadly categorized into those used for sequence data processing, taxonomic assignment, functional annotation, and statistical analysis. Some of the most widely used tools include QIIME, mothur, MetaPhlAn, and HUMAnN.
Challenges in Microbiome Analysis
Despite the advances in bioinformatics, there are still several challenges in microbiome analysis. These include the difficulty in distinguishing between true biological variation and technical noise, the lack of standardized protocols and tools, and the need for more comprehensive databases and computational resources.
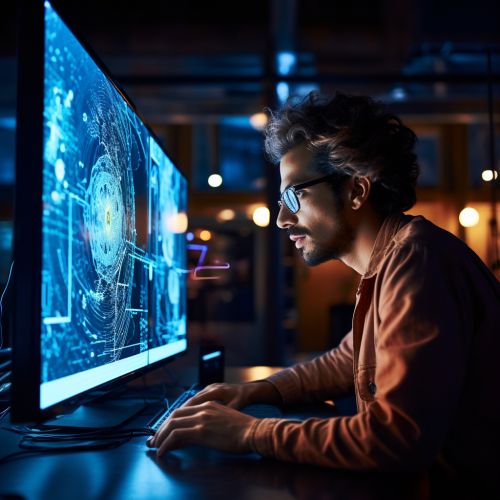
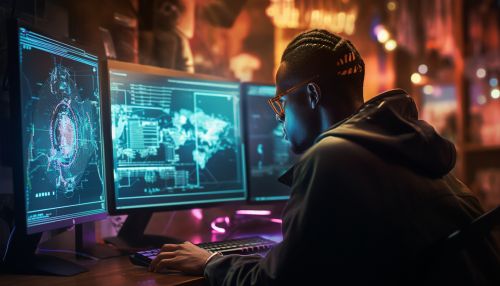
Future Directions
The field of bioinformatics is continually evolving, and new tools and methodologies are being developed to address the challenges in microbiome analysis. These include the use of machine learning and artificial intelligence to analyze microbiome data, the development of more comprehensive and accurate databases, and the integration of multi-omics data for a more holistic understanding of microbiomes.
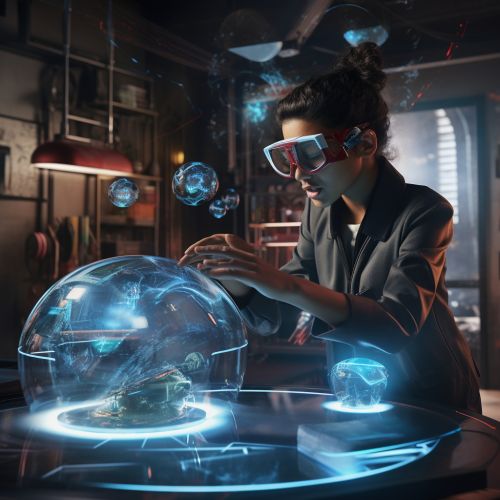
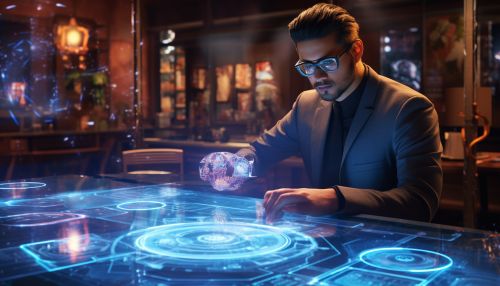