The Physics of Quantum Mechanics in Quantum Machine Learning
Introduction
Quantum mechanics is a fundamental theory in physics that describes nature at the smallest scales of energy levels of atoms and subatomic particles. It is the foundation of all quantum physics including quantum field theory, quantum chemistry, quantum computing, and quantum information.
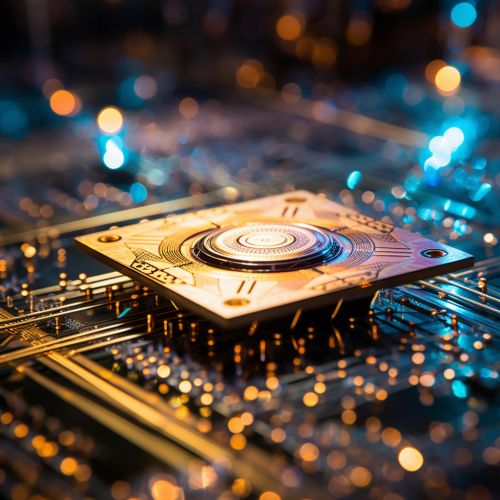
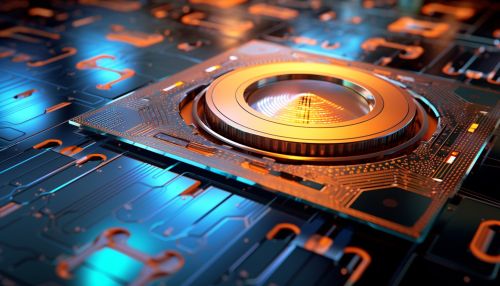
Quantum Mechanics and Machine Learning
Quantum mechanics and machine learning are two seemingly disparate fields that have found common ground in recent years. Quantum machine learning, an emerging field, involves integrating quantum algorithms into machine learning models. Quantum algorithms, such as the Quantum Fourier Transform and Quantum Phase Estimation, can provide exponential speedup over classical algorithms, making them potentially beneficial for machine learning tasks.
Quantum Algorithms in Machine Learning
Quantum algorithms can be used in various aspects of machine learning, including data preprocessing, model training, and prediction. For instance, the Quantum Support Vector Machine (QSVM) is a quantum version of the classical support vector machine, a popular machine learning model for classification and regression tasks. QSVM utilizes quantum states to represent data and quantum operations to manipulate these states, potentially offering computational advantages over classical SVMs.
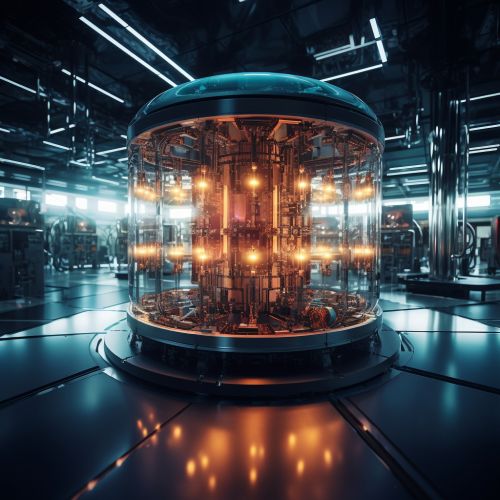
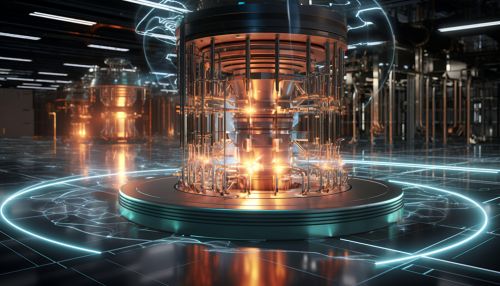
Quantum Data in Machine Learning
In addition to quantum algorithms, quantum data can also play a crucial role in quantum machine learning. Quantum data refers to data that is encoded in the state of quantum systems. This data can be processed by quantum computers in a way that is fundamentally different from classical computers. For example, the Quantum Amplitude Estimation algorithm can be used to estimate properties of quantum data, which can be useful in various machine learning tasks.
Challenges and Future Directions
Despite the promising potential of quantum machine learning, there are several challenges that need to be addressed. These include the lack of large-scale quantum computers, the difficulty in understanding and interpreting quantum algorithms, and the need for new theoretical frameworks to guide the development of quantum machine learning models. However, with ongoing research and technological advancements, the field of quantum machine learning is expected to continue to grow and evolve.
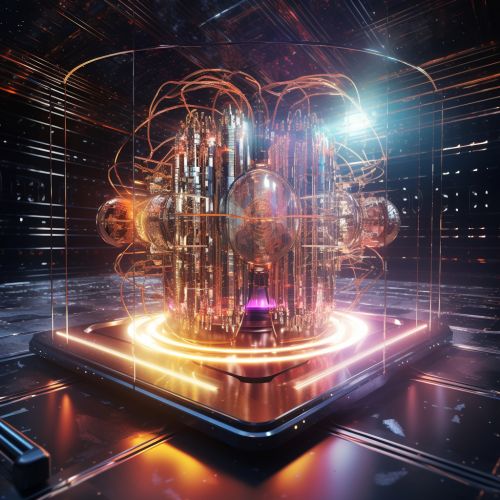
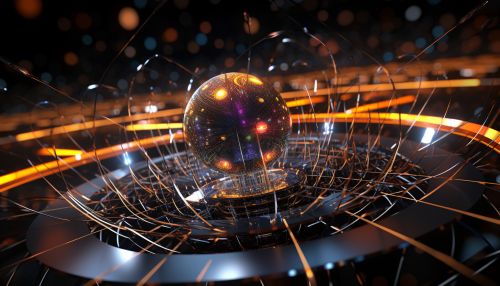