Structural Equation Modeling
Introduction
Structural Equation Modeling (SEM) is a multivariate statistical analysis technique used to analyze structural relationships. This technique is the combination of factor analysis and multiple regression analysis, and it is used to analyze the structural relationship between measured variables and latent constructs. This is why it is called structural equation modeling.
History
The history of structural equation modeling can be traced back to the work of Sewall Wright, Karl Gustav Jöreskog and other researchers. Wright is credited with the development of path analysis, which is considered a precursor to SEM. Jöreskog developed the general structural equation models – a major component of SEM.
Basics of SEM
SEM includes confirmatory rather than exploratory factor analysis. It also includes multiple regression analysis. SEM allows for the examination of a series of relationships between variables simultaneously. It is a powerful tool that allows for tests of complex theoretical models.
SEM Components
SEM consists of two main components: the structural model and the measurement model. The structural model shows potential causal dependencies between endogenous and exogenous variables. The measurement model shows the relations between latent variables and their indicators. Combining these two models gives the SEM.
SEM Assumptions
There are several assumptions made when using SEM. These include the assumption of linearity, the assumption of normality, and the assumption of homoscedasticity. Violations of these assumptions can lead to inaccurate results.
SEM Steps
The process of conducting SEM involves several steps. These include model specification, identification, estimation, testing, and modification. Each of these steps is crucial in obtaining accurate and meaningful results.
SEM Software
There are several software packages available for conducting SEM. These include AMOS, LISREL, Mplus, and R. Each of these software packages has its own strengths and weaknesses, and the choice of software should be based on the specific needs of the analysis.
SEM Applications
SEM has been widely used in many fields, including psychology, education, business, and social sciences. It is a powerful tool for testing hypotheses about causal relationships among observed and latent variables.
Limitations of SEM
Despite its strengths, SEM is not without its limitations. These include the requirement for large sample sizes, the difficulty of testing complex models, and the potential for misuse due to the complexity of the technique.
Conclusion
In conclusion, Structural Equation Modeling is a powerful statistical tool that allows for the testing of complex theoretical models. Despite its limitations, it is widely used in many fields and offers many advantages over traditional statistical techniques.
See Also
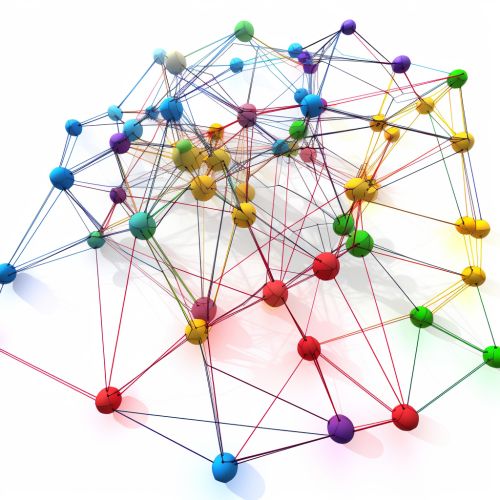
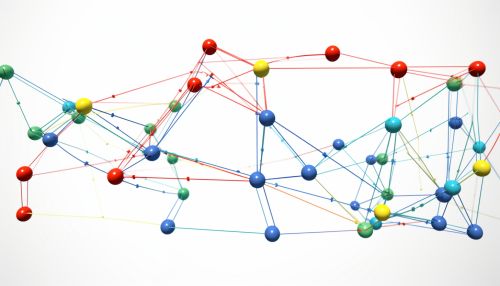