Stochastic Processes in Population Dynamics
Introduction
A stochastic process is a mathematical object usually defined as a family of random variables. Historically, the random variables were associated with or indexed by a set of numbers, usually viewed as points in time, giving the interpretation of a stochastic process representing numerical values of some system randomly changing over time, such as the growth rate of a population.
Population dynamics refers to the ways in which populations change in size and structure over time. In the field of population dynamics, stochastic processes are used to model the random elements that can affect the growth or decline of a population. This includes factors such as random births and deaths, but also broader environmental factors that can have a random effect on the population size.
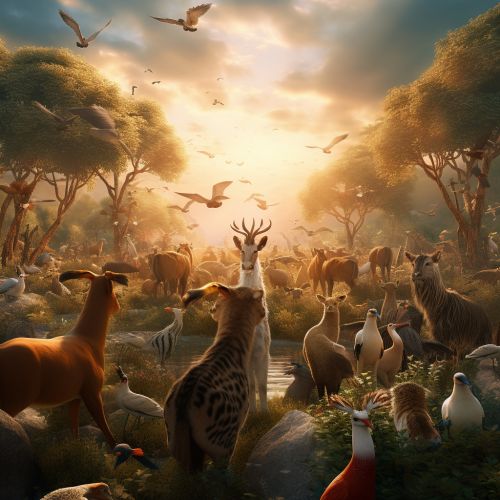
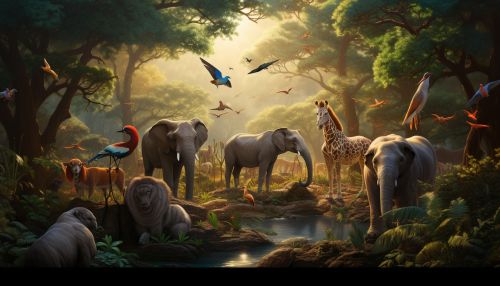
Stochastic Processes in Population Dynamics
Stochastic processes are used in population dynamics to account for the inherent randomness in the birth and death process. This randomness can arise from a variety of sources, including environmental variability, demographic stochasticity, and genetic drift.
Environmental Variability
Environmental variability, also known as environmental noise, refers to the random fluctuations in environmental conditions. These fluctuations can have a significant impact on population dynamics, affecting birth rates, death rates, and migration rates. For example, a sudden change in weather conditions can lead to an unexpected increase in death rates, causing a rapid decline in the population size.
Demographic Stochasticity
Demographic stochasticity refers to the random variation in birth and death rates due to the discrete nature of individuals in a population. Even if the average birth and death rates are known, the actual number of births and deaths that occur in a given time period can vary due to chance. This can lead to fluctuations in population size, especially in small populations.
Genetic Drift
Genetic drift is a stochastic process that refers to the random changes in allele frequencies in a population due to chance. This can lead to changes in the genetic composition of the population over time, which can in turn affect the population dynamics. For example, if a particular allele that confers resistance to a disease becomes more common due to genetic drift, this could lead to a decrease in death rates and an increase in population size.
Mathematical Models of Stochastic Processes in Population Dynamics
There are several mathematical models that are commonly used to represent stochastic processes in population dynamics. These include the Gillespie algorithm, the master equation, and the Fokker-Planck equation.
Gillespie Algorithm
The Gillespie algorithm is a computational method used to simulate the time evolution of a stochastic process. It is particularly useful in population dynamics, as it allows for the simulation of populations with small numbers of individuals, where demographic stochasticity can have a significant impact.
Master Equation
The master equation is a mathematical model that describes the probabilities of different states in a stochastic process. In the context of population dynamics, it can be used to model the probabilities of different population sizes over time.
Fokker-Planck Equation
The Fokker-Planck equation is a partial differential equation that describes the time evolution of the probability density function of a stochastic process. It is often used in population dynamics to model the effects of environmental variability and genetic drift on population size.
Applications of Stochastic Processes in Population Dynamics
Stochastic processes have a wide range of applications in population dynamics. They are used in the study of epidemiology, ecology, conservation biology, and evolutionary biology, among other fields.
Epidemiology
In epidemiology, stochastic processes are used to model the spread of diseases in a population. These models can take into account the random nature of disease transmission, recovery, and death, providing a more realistic representation of disease dynamics than deterministic models.
Ecology
In ecology, stochastic processes are used to model the dynamics of populations and communities. These models can incorporate the effects of environmental variability, demographic stochasticity, and genetic drift, providing a more comprehensive understanding of ecological dynamics.
Conservation Biology
In conservation biology, stochastic processes are used to assess the risk of extinction for endangered species. These models can take into account the effects of environmental variability, demographic stochasticity, and genetic drift, providing a more accurate assessment of extinction risk than deterministic models.
Evolutionary Biology
In evolutionary biology, stochastic processes are used to model the dynamics of genetic variation in a population. These models can incorporate the effects of mutation, selection, and genetic drift, providing a more comprehensive understanding of evolutionary dynamics.