Sampling (statistics)
Introduction
Sampling is a fundamental concept in the field of statistics that involves selecting a subset of individuals from within a statistical population to estimate characteristics of the whole population. It is a tool used by statisticians to infer information about a population based on results from a subset, or sample, of that population.
Types of Sampling
There are various types of sampling methods used in statistics, each with its own strengths and weaknesses. These include:
- Simple Random Sampling
- Stratified Sampling
- Cluster Sampling
- Systematic Sampling
- Quota Sampling
- Non-probability Sampling
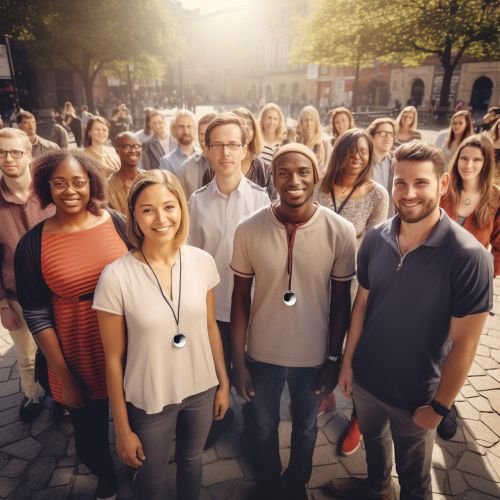
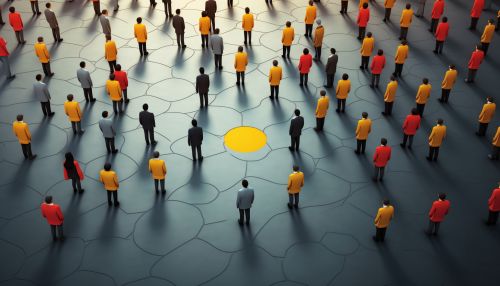
Simple Random Sampling
Simple random sampling is the most basic form of sampling. Every member of the population has an equal chance of being selected in the sample. This method is best used when the entire population is available for sampling.
Stratified Sampling
Stratified sampling involves dividing the population into homogeneous subgroups, or strata, and then taking a simple random sample from each stratum. This method is beneficial when the population is heterogeneous, or diverse.
Cluster Sampling
Cluster sampling involves dividing the population into clusters, or groups, and then selecting entire clusters at random for sampling. This method is useful when it is difficult or costly to sample from the entire population.
Systematic Sampling
Systematic sampling involves selecting every nth member of the population for the sample. This method is often used when a stream of representative individuals is available.
Quota Sampling
Quota sampling is a non-probability sampling method that involves selecting individuals based on specific traits, ensuring that the sample represents those traits in the population. This method is often used in market research.
Non-probability Sampling
Non-probability sampling methods are those that do not involve random selection. These methods include convenience sampling, judgment sampling, quota sampling, and snowball sampling. These methods are often used when the population is not well-defined, or when random selection is not feasible.
Importance of Sampling
Sampling is an essential tool in statistics because it allows for the estimation of population parameters with a known level of accuracy. It also allows for the testing of hypotheses about the population. Furthermore, sampling is often more practical and cost-effective than attempting to survey an entire population.
Sampling Error
Sampling error refers to the difference between the estimate derived from a sample and the true population value. This error can be reduced by increasing the sample size and using appropriate sampling methods.
Conclusion
In conclusion, sampling is a critical component of statistical analysis. It allows for the estimation of population parameters and hypothesis testing, and it is often more practical and cost-effective than surveying an entire population. However, sampling also introduces error, which must be carefully managed to ensure accurate results.