Representativeness Heuristic
Introduction
The Representativeness Heuristic is a cognitive bias that influences the decision-making process in humans. It is a mental shortcut that helps individuals make judgments about the probability of an event under uncertainty.
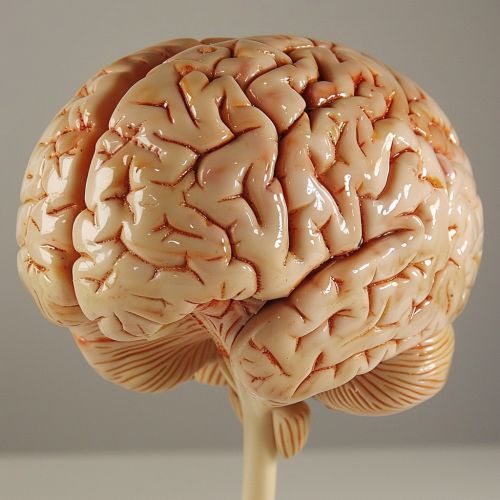
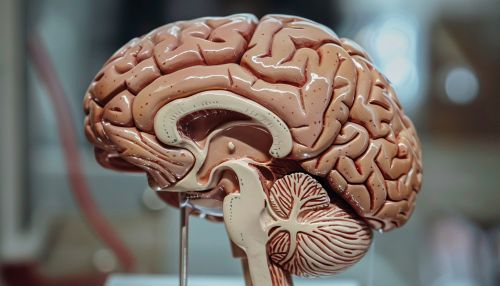
Concept
The Representativeness Heuristic was first introduced by psychologists Amos Tversky and Daniel Kahneman in 1972. It is a part of their Heuristics and Biases research program, which aims to understand the cognitive biases that influence human decision-making and judgment.
The heuristic is based on the principle of representativeness, where people judge the probability or frequency of a hypothesis by considering how much the hypothesis resembles available data. In other words, individuals tend to believe that outcomes that are more representative are also more likely.
Mechanism
The Representativeness Heuristic operates on the principle of similarity. When faced with uncertainty, individuals tend to classify objects or events based on how similar they are to the prototype in their mind. This prototype is often based on stereotypes or past experiences.
For instance, if a person is described as quiet and introverted, people might assume that this person is more likely to be a librarian than a salesperson, based on the stereotypical image of these professions. This is despite the fact that there are many more salespeople than librarians, making it statistically more likely for a randomly selected person to be a salesperson.
Applications
The Representativeness Heuristic has been applied in various fields, including psychology, economics, and artificial intelligence.
In psychology, the heuristic is used to explain certain cognitive biases, such as the Base Rate Fallacy and the Conjunction Fallacy. These biases occur when individuals ignore base rates or statistical information in favor of representativeness.
In economics, the heuristic is used to understand consumer behavior and market trends. For example, investors might judge the quality of an investment based on how similar it is to successful investments in the past, ignoring other relevant information.
In artificial intelligence, the heuristic is used in machine learning algorithms to classify data based on its similarity to existing data.
Criticisms
While the Representativeness Heuristic is a useful tool for understanding human decision-making, it has been criticized for its oversimplification of the cognitive process. Critics argue that the heuristic does not account for other factors that might influence decision-making, such as emotions or social influences.
Furthermore, the heuristic can lead to biases and errors in judgment. For instance, individuals might overestimate the likelihood of representative events and underestimate the likelihood of non-representative events. This can lead to irrational decisions and behaviors.
Conclusion
The Representativeness Heuristic is a fundamental concept in cognitive psychology. Despite its limitations, it provides valuable insights into the cognitive biases that shape human decision-making and judgment. Understanding these biases can help individuals make more rational decisions and improve their critical thinking skills.