Representativeness
Overview
Representativeness is a cognitive heuristic in which individuals make judgments about the probability of an event under uncertainty. It is one of several heuristics (simple rules governing judgment or decision-making) proposed by psychologists Amos Tversky and Daniel Kahneman in the early 1970s. Individuals using the representativeness heuristic make decisions based on how similar something is to a prototype they have in mind. This heuristic is frequently used in everyday decision making and, while it can be beneficial, it can also lead to neglect of relevant base rates and other cognitive biases.
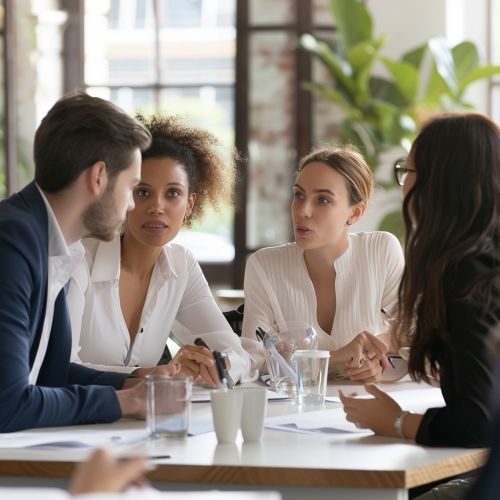
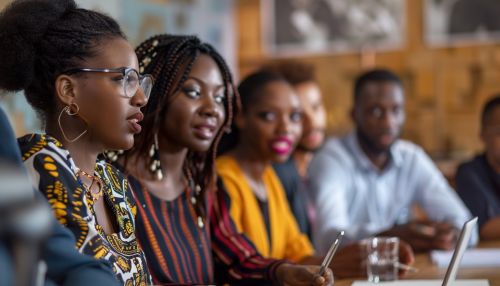
History and Development
The concept of representativeness was first introduced by Tversky and Kahneman in their seminal 1972 paper, "Judgment under Uncertainty: Heuristics and Biases". The authors proposed that individuals rely on a limited number of heuristic principles which reduce the complex tasks of assessing probabilities and predicting values to simpler judgmental operations. In a later paper, Kahneman and Tversky further elaborated on the concept, describing how the representativeness heuristic can lead to predictable biases in many situations.
Function and Mechanism
The representativeness heuristic operates under the belief that if something is similar in characteristics to a general category, then it belongs to that category. For example, if a person is described as quiet and withdrawn, they might be judged as more likely to be a librarian than a salesperson, because the description is more representative of the stereotype of librarians.
Applications
The representativeness heuristic has been applied in various fields, such as psychology, economics, and artificial intelligence. In psychology, it is often used to explain the occurrence of certain cognitive biases. In economics, the heuristic is used to model bounded rationality, where decision-makers are limited by the information they have, their cognitive limitations, and the finite amount of time they have to make a decision. In artificial intelligence, the heuristic is used in machine learning algorithms to classify data into predefined categories.
Criticisms and Controversies
Despite its wide acceptance and application, the representativeness heuristic has been criticized on several fronts. Some critics argue that the heuristic is too vague and lacks a clear definition. Others argue that it is not a heuristic at all, but rather a bias or a fallacy. There are also debates about the extent to which the heuristic is used in everyday decision making.