Random Selection
Introduction
Random selection, also known as random sampling, is a fundamental concept in statistics and probability theory. It refers to the process of selecting a subset of individuals or items from a larger population in such a way that each individual or item has an equal chance of being chosen. This method is widely used in various fields, including scientific research, survey methodology, and quality control, to ensure that the selected sample is representative of the entire population.
Theoretical Foundations
Random selection is grounded in the principles of probability theory. The key idea is that each element in the population has a non-zero probability of being included in the sample. This ensures that the sample is unbiased and can be used to make valid inferences about the population.
Probability Theory
Probability theory provides the mathematical framework for random selection. It involves the study of random events and the likelihood of their occurrence. Key concepts include probability distributions, expected value, and variance. These concepts help in understanding the behavior of random samples and in making predictions about the population.
Sampling Methods
There are several methods of random sampling, each with its own advantages and limitations. The most common methods include:
- Simple Random Sampling: Every member of the population has an equal chance of being selected. This can be achieved using random number generators or drawing lots.
- Stratified Sampling: The population is divided into subgroups (strata) based on certain characteristics, and random samples are drawn from each stratum. This ensures that the sample is representative of all subgroups.
- Systematic Sampling: Every nth member of the population is selected, starting from a randomly chosen point. This method is easier to implement but may introduce periodicity biases.
- Cluster Sampling: The population is divided into clusters, and a random sample of clusters is selected. All members of the chosen clusters are included in the sample. This method is useful when the population is large and geographically dispersed.
Applications of Random Selection
Random selection is used in various fields to achieve unbiased and representative samples.
Scientific Research
In scientific research, random selection is crucial for designing experiments and observational studies. It helps in eliminating selection bias and ensuring that the results are generalizable to the entire population. For example, in clinical trials, random selection is used to assign participants to treatment and control groups, minimizing the effects of confounding variables.
Survey Methodology
Surveys rely on random sampling to gather data from a subset of the population. This data is then used to make inferences about the entire population. Random selection ensures that the survey results are accurate and reliable. Techniques such as random digit dialing and random route sampling are commonly used in survey research.
Quality Control
In manufacturing and quality control, random sampling is used to inspect products and ensure that they meet quality standards. By randomly selecting items for inspection, manufacturers can identify defects and improve their production processes. This method is also used in auditing to detect errors and fraud.
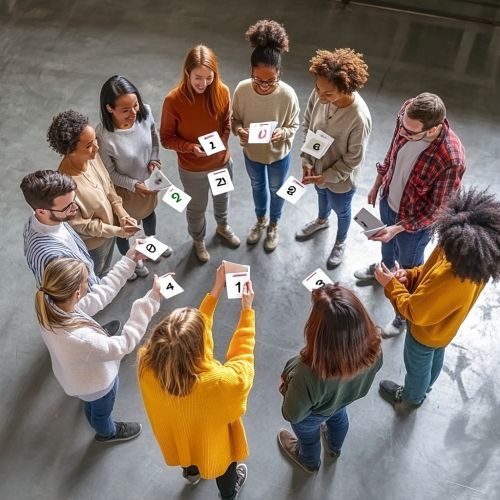
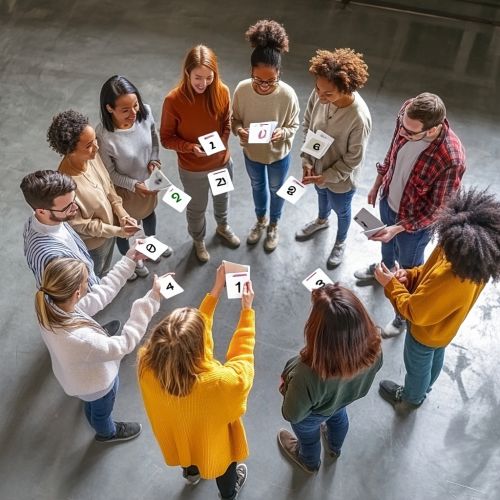
Statistical Inference
Statistical inference involves drawing conclusions about a population based on a random sample. This process relies on the principles of random selection to ensure that the sample is representative and the inferences are valid.
Estimation
Estimation is the process of using sample data to estimate population parameters, such as the mean or proportion. Point estimates provide a single value, while interval estimates provide a range of values within which the parameter is likely to lie. Confidence intervals are commonly used to express the uncertainty associated with an estimate.
Hypothesis Testing
Hypothesis testing is a method of making decisions about population parameters based on sample data. It involves formulating a null hypothesis and an alternative hypothesis, and using statistical tests to determine whether there is enough evidence to reject the null hypothesis. Common tests include the t-test, chi-square test, and ANOVA.
Challenges and Limitations
While random selection is a powerful tool, it is not without challenges and limitations.
Sampling Error
Sampling error is the difference between the sample statistic and the true population parameter. It arises due to the inherent variability in random sampling. Larger sample sizes can reduce sampling error, but it cannot be completely eliminated.
Nonresponse Bias
Nonresponse bias occurs when individuals selected for the sample do not respond. This can lead to biased results if the nonrespondents differ significantly from the respondents. Techniques such as follow-up surveys and weighting adjustments are used to mitigate nonresponse bias.
Practical Constraints
In practice, random selection may be constrained by factors such as cost, time, and accessibility. For example, it may be difficult to obtain a complete list of the population or to reach certain individuals. These constraints can limit the feasibility and accuracy of random sampling.
Ethical Considerations
Random selection also raises ethical considerations, particularly in research involving human subjects. It is important to ensure that the selection process is fair and that participants are not exposed to unnecessary risks.
Informed Consent
Informed consent is a fundamental ethical requirement in research. Participants must be fully informed about the study and its potential risks and benefits, and they must voluntarily agree to participate. Random selection should not compromise the process of obtaining informed consent.
Equity and Fairness
Random selection should be conducted in a manner that is equitable and fair. This means that all individuals in the population should have an equal chance of being selected, and that the selection process should not discriminate against any group.