Numerical weather prediction
Introduction
Numerical weather prediction (NWP) refers to the use of mathematical models in the prediction of the atmosphere's behavior. It is a principal application of computational fluid dynamics and partial differential equations that are used to solve the equations that describe atmospheric dynamics. The goal of NWP is to predict future weather patterns based on current atmospheric conditions.
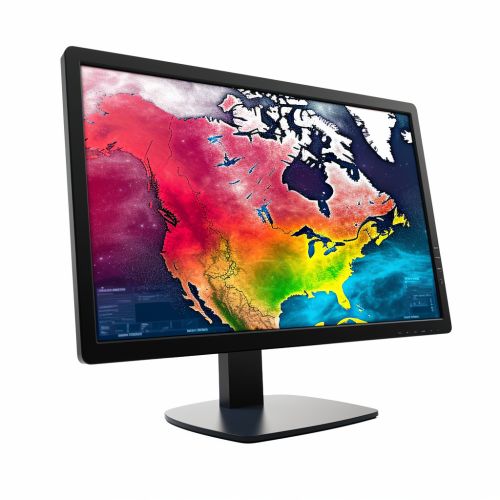
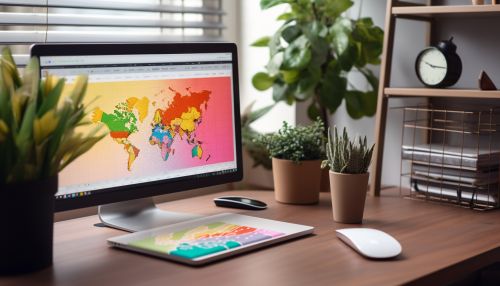
History
The concept of numerical weather prediction was first proposed by Lewis Fry Richardson, a British mathematician, in the early 20th century. Richardson's idea was to divide the world into a grid and solve the equations of motion for each grid point. However, the computational power required to perform such calculations was not available at the time.
The first successful numerical weather prediction was carried out by a group led by Jule Charney at the Institute for Advanced Study in Princeton, New Jersey, in 1950. The group used the ENIAC computer, the most powerful computer of the time, to perform the calculations.
Mathematical Models
The mathematical models used in NWP are based on the Navier-Stokes equations, which describe the motion of fluid substances such as air. These equations are then combined with the ideal gas law and the first law of thermodynamics to form a complete mathematical model of the atmosphere.
These models are then discretized using techniques such as finite difference methods, finite volume methods, or spectral methods. The resulting system of equations is then solved using numerical methods.
Data Assimilation
Data assimilation is a critical component of numerical weather prediction. It involves the integration of observational data into the NWP models to improve their accuracy. This data comes from a variety of sources, including weather stations, weather balloons, weather satellites, and radar.
Data assimilation techniques include the Kalman filter, the optimal interpolation, and the 4D-Var method. These techniques are used to combine the observational data with the model's forecast to produce a more accurate initial condition for the model.
Forecasting
Once the initial conditions have been determined through data assimilation, the NWP model can be used to forecast future weather conditions. This is done by integrating the model's equations forward in time.
The accuracy of NWP forecasts depends on several factors, including the accuracy of the initial conditions, the resolution of the model, and the physical parameterizations used in the model. Despite these challenges, NWP models have proven to be highly effective tools for weather forecasting.
Limitations and Challenges
Despite the significant advancements in numerical weather prediction, there are still several challenges and limitations. These include the chaotic nature of the atmosphere, the difficulty in accurately representing physical processes such as cloud formation and precipitation, and the computational cost of running high-resolution models.
Furthermore, there are also challenges associated with data assimilation, including the handling of errors in observational data and the integration of data from different sources.
Future Directions
The future of numerical weather prediction lies in the development of more accurate models, the improvement of data assimilation techniques, and the utilization of increasing computational power. There is also a growing interest in the use of machine learning techniques in NWP, particularly in the area of data assimilation.