Microarray Analysis
Introduction
Microarray analysis is a high-throughput method used in molecular biology and in medicine to simultaneously assess the expression levels of thousands of genes across multiple samples. This technology has revolutionized genomic research by allowing comprehensive analysis of gene expression in a single experiment. The technique is based on two scientific principles: the hybridization between two DNA strands forming a stable double-stranded DNA structure and the light absorption properties of DNA.
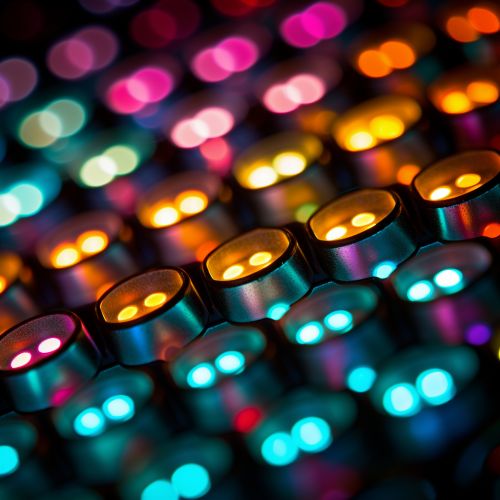
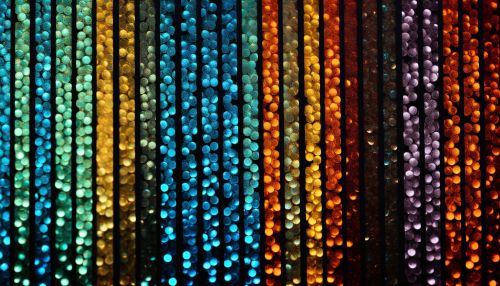
Principle of Microarray Analysis
The fundamental principle of microarray analysis is hybridization. In this process, two complementary strands of DNA bind to form a stable double-stranded DNA molecule. The degree of hybridization between the DNA on the array and the labeled cDNA or cRNA target is measured and interpreted as a relative measure of gene expression.
Microarrays are typically constructed by robotic application of DNA samples onto a solid support, such as a glass slide. Each spot on the array represents a different gene or sequence. The DNA on the array is known as the probe, and the labeled cDNA or cRNA target is known as the target.
Types of Microarrays
There are several types of microarrays, including DNA microarrays (also known as gene chips), oligonucleotide microarrays, and cDNA microarrays. The choice of which type of microarray to use depends on the specific requirements of the experiment, such as the number of genes to be analyzed, the availability of sequence information, and the desired resolution of the analysis.
DNA Microarrays
DNA microarrays, also known as gene chips, are the most common type of microarray. They are typically used for gene expression profiling, comparative genomic hybridization, and genotyping. DNA microarrays consist of DNA probes that are attached to a solid surface, such as a glass slide. Each probe corresponds to a specific gene or a part of a gene.
Oligonucleotide Microarrays
Oligonucleotide microarrays consist of short DNA sequences that are synthesized directly onto the array surface. These arrays are typically used for SNP detection, resequencing, and gene expression profiling. The main advantage of oligonucleotide microarrays is their high specificity and sensitivity, which allows for the detection of single nucleotide polymorphisms (SNPs).
cDNA Microarrays
cDNA microarrays are constructed by spotting cDNA onto a solid surface. These arrays are typically used for gene expression profiling. The main advantage of cDNA microarrays is their ability to detect transcripts that are not represented in the genome, such as alternatively spliced transcripts.
Microarray Analysis Process
The process of microarray analysis involves several steps, including sample preparation, hybridization, scanning, and data analysis.
Sample Preparation
The first step in microarray analysis is sample preparation. This involves extracting RNA from the sample, converting the RNA to cDNA or cRNA, and labeling the cDNA or cRNA with a fluorescent dye. The labeled cDNA or cRNA is then purified to remove unincorporated dye and other impurities.
Hybridization
The next step is hybridization. The labeled cDNA or cRNA is applied to the microarray and incubated under conditions that promote hybridization between the probe and target. The unbound target is then washed away, leaving only the bound target on the array.
Scanning
After hybridization, the array is scanned using a microarray scanner. The scanner uses a laser to excite the fluorescent dye, and a detector measures the intensity of the emitted light. The intensity of the light is proportional to the amount of bound target, which reflects the level of gene expression.
Data Analysis
The final step in microarray analysis is data analysis. This involves normalizing the data to correct for variations in labeling efficiency and other experimental factors, and then analyzing the normalized data to identify differentially expressed genes. Various statistical methods can be used for this analysis, including t-tests, analysis of variance (ANOVA), and clustering algorithms.
Applications of Microarray Analysis
Microarray analysis has a wide range of applications in various fields of biological and medical research.
Gene Expression Profiling
One of the main applications of microarray analysis is gene expression profiling. This involves comparing the expression levels of thousands of genes across different samples to identify genes that are differentially expressed. This can provide insights into the molecular mechanisms underlying various biological processes and diseases.
Comparative Genomic Hybridization
Another application of microarray analysis is comparative genomic hybridization (CGH). This involves comparing the genomic content of different samples to identify genomic gains and losses. This can provide insights into the genetic changes associated with diseases such as cancer.
Genotyping
Microarray analysis can also be used for genotyping. This involves determining the genetic makeup of an individual by analyzing their DNA sequence. This can be used for a variety of purposes, including disease diagnosis, drug response prediction, and ancestry determination.
Limitations and Challenges
Despite its many advantages, microarray analysis also has several limitations and challenges. These include the high cost of microarray experiments, the complexity of data analysis, and the difficulty in validating the results. In addition, microarray analysis can only detect known genes, and it cannot detect post-transcriptional modifications or protein-protein interactions.
Future Directions
The field of microarray analysis is constantly evolving, with new technologies and methodologies being developed to overcome the limitations and challenges of current techniques. These include the development of next-generation sequencing technologies, which can provide higher resolution and more comprehensive analysis than microarrays, and the integration of microarray data with other types of data, such as proteomic and metabolomic data, to provide a more comprehensive understanding of biological systems.