Knowledge graph
Introduction
A knowledge graph is a specific kind of data structure that has emerged as a valuable tool for data organization and retrieval in the field of artificial intelligence and semantic web. It is a collection of interlinked descriptions of entities – objects, events, or concepts – which are organized in a graph structure. This structure allows for complex relationships between entities to be easily represented and understood, both by humans and machines.

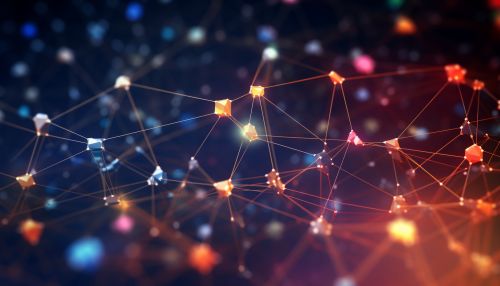
History and Development
The concept of a knowledge graph has its roots in the field of semantic networks and artificial intelligence, dating back to the 1960s and 1970s. However, the term "knowledge graph" itself was popularized by Google in 2012, when they introduced their own Google Knowledge Graph as a means to enhance their search engine's results with information gathered from a variety of sources.
Structure
A knowledge graph is composed of nodes and edges. The nodes represent entities, which can be anything from people, places, and things to ideas, events, or abstract concepts. The edges, on the other hand, represent the relationships or connections between these entities. Each edge is labeled with a type of relationship, and each node is labeled with a type of entity.
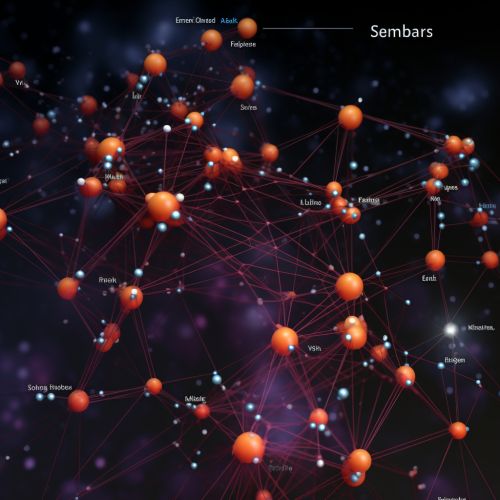
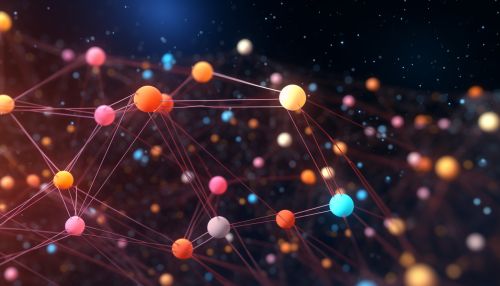
Creation and Maintenance
The creation of a knowledge graph involves several steps, including data gathering, data cleaning, entity recognition, relationship extraction, and graph construction. The maintenance of a knowledge graph involves regular updates to keep the information current and accurate, as well as the addition of new entities and relationships as they become relevant.
Applications
Knowledge graphs have a wide range of applications in various fields. In the field of information retrieval, they are used to enhance search results by providing additional context and related information. In natural language processing, they are used to improve understanding of the meaning of words in context. In data integration, they are used to link together disparate data sources and provide a unified view of the data.
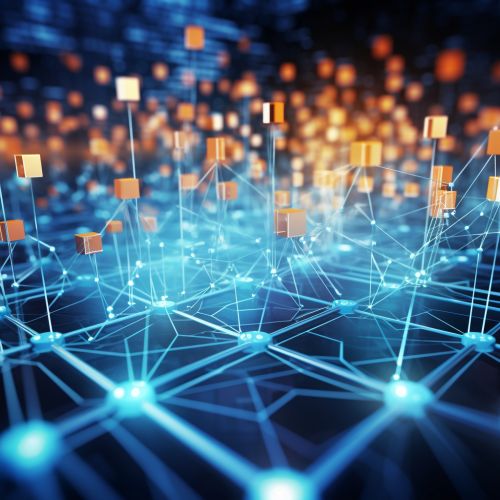
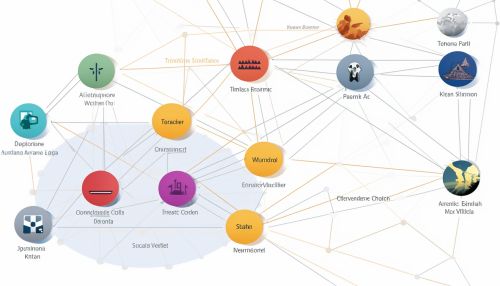
Challenges
Despite their many benefits, knowledge graphs also present several challenges. These include the difficulty of creating and maintaining a large and complex graph, the challenge of ensuring the accuracy and reliability of the information in the graph, and the issue of privacy and security when dealing with sensitive data.
Future Directions
The future of knowledge graphs looks promising, with ongoing research and development in areas such as graph learning, graph embeddings, and dynamic knowledge graphs. There is also increasing interest in the use of knowledge graphs for tasks such as question answering, recommendation systems, and semantic search.
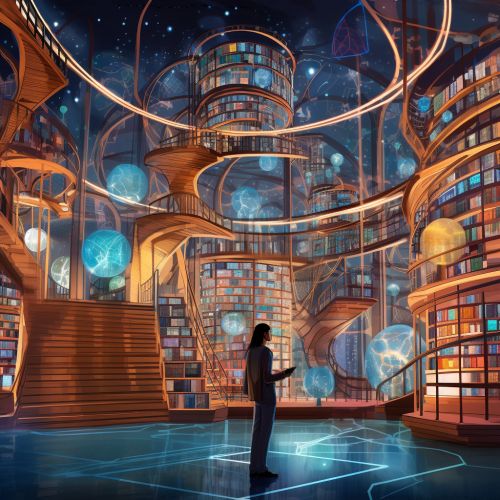
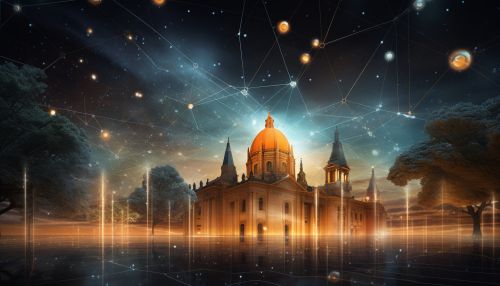