Hebbian Theory
Introduction
Hebbian theory is a neuroscientific theory proposing that an increase in synaptic efficacy arises from a presynaptic cell's repeated and persistent stimulation of a postsynaptic cell. It is an attempt to explain synaptic plasticity, the adaptation of brain neurons during the learning process. The theory is named after Canadian neuropsychologist Donald O. Hebb, who introduced it in his 1949 book The Organization of Behavior. Hebb's postulate is often summarized as "Cells that fire together, wire together". This means the connections between neurons in the brain can change, grow stronger or weaker over time, depending on whether they are activated at the same time.
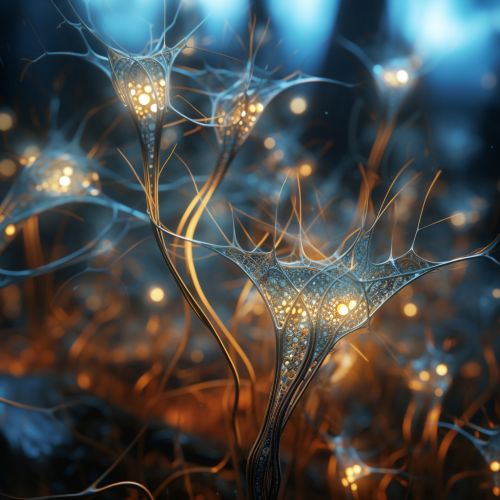
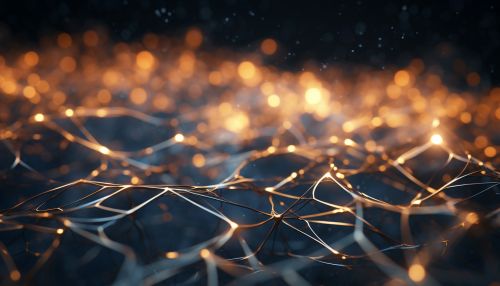
Biological Basis
The Hebbian theory is grounded in the biological and physical realities of electrical activity in the brain and the actual structures of neurons. Neurons are connected by synapses, and it is these synapses that allow electrical or chemical signals to pass from one neuron to the next. Hebbian theory proposes that when two neurons are activated simultaneously, the synapse connecting them strengthens. This is often referred to as synaptic plasticity or long-term potentiation (LTP). Conversely, if two neurons are rarely activated simultaneously, the synapse between them weakens, a process known as long-term depression (LTD).
Hebb's Postulate
Hebb's postulate, also known as Hebb's rule, states that the repeated activation of two neurons or systems of neurons in sequence leads to strengthened connections between them. This is often summarized as "neurons that fire together, wire together" and "neurons that fire out of sync, lose their link". This postulate is considered foundational in the field of neural networks and has been used to explain a variety of phenomena, including associative learning.
Applications of Hebbian Theory
Hebbian theory has been applied in numerous fields, including psychology, neuroscience, computer science, and artificial intelligence. In psychology and neuroscience, it has been used to explain how memory and learning occur. The theory suggests that learning involves strengthening the connections between neurons that work together to produce a particular outcome, thereby making it easier for these neurons to trigger one another and produce the same outcome in the future.
In computer science and artificial intelligence, Hebbian learning rules have been used in the design of artificial neural networks. These networks, which are designed to mimic the neural networks in the brain, use Hebbian learning to adjust the weights of the connections between artificial neurons. This allows the network to learn and adapt to new information, much like the brain does.
Criticisms and Limitations
While Hebbian theory has been influential in our understanding of learning and memory, it is not without its criticisms and limitations. One of the main criticisms is that it does not account for the role of inhibitory synapses, which prevent or limit the firing of a postsynaptic neuron. Another criticism is that it does not explain how a specific memory is retrieved once it has been stored.
In terms of limitations, Hebbian theory is a relatively simple model of learning and does not account for the complexity of most learning processes. For example, it does not explain how we can learn new concepts or skills without repeated practice. Furthermore, while Hebbian learning can explain how connections between neurons can change over time, it does not explain how these changes translate into changes in behavior or cognition.