Healthcare Data Analytics
Introduction
Healthcare data analytics refers to the use of big data and data science techniques to extract insights from large and complex sets of healthcare data. This field has emerged as a critical component of modern healthcare systems, enabling healthcare providers to improve patient care, reduce costs, and streamline operations.
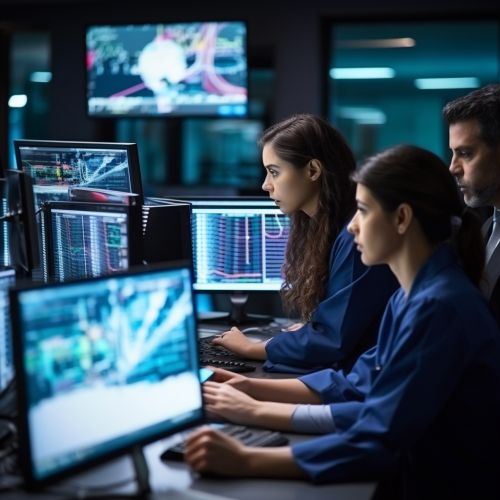
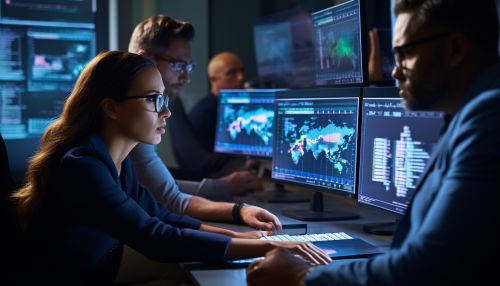
History and Evolution
The history of healthcare data analytics can be traced back to the late 20th century when healthcare providers began to leverage electronic health records (EHRs) to store and manage patient data. Over time, the volume of healthcare data grew exponentially, necessitating the development of advanced analytics techniques to process and analyze this data.
Types of Healthcare Data
Healthcare data can be broadly classified into three categories: structured data, unstructured data, and semi-structured data.
Structured Data
Structured data refers to information that is organized in a predefined manner and is easily searchable. Examples of structured data in healthcare include patient demographics, lab results, and medication lists.
Unstructured Data
Unstructured data, on the other hand, is information that does not have a predefined structure or format. This includes clinical notes, radiology images, and genomic data.
Semi-Structured Data
Semi-structured data falls somewhere in between structured and unstructured data. It has some level of organization but does not conform to the rigid structure of structured data. Examples include email communications and electronic health record (EHR) data.
Techniques Used in Healthcare Data Analytics
Several techniques are commonly used in healthcare data analytics, including predictive analytics, prescriptive analytics, descriptive analytics, and machine learning.
Predictive Analytics
Predictive analytics involves using statistical techniques to predict future outcomes based on historical data. In healthcare, predictive analytics can be used to forecast patient outcomes, predict disease outbreaks, and identify at-risk populations.
Prescriptive Analytics
Prescriptive analytics goes a step further than predictive analytics by not only predicting future outcomes but also suggesting actions to achieve desired outcomes. For instance, prescriptive analytics can recommend treatment plans for individual patients based on their unique health profiles.
Descriptive Analytics
Descriptive analytics involves analyzing historical data to understand past trends and patterns. This can help healthcare providers identify areas of improvement and develop strategies to enhance patient care.
Machine Learning
Machine learning, a subset of artificial intelligence, involves training algorithms to learn from data and make predictions or decisions without being explicitly programmed. In healthcare, machine learning can be used to predict disease progression, personalize treatment plans, and detect anomalies in medical images.
Applications of Healthcare Data Analytics
Healthcare data analytics has a wide range of applications, from improving patient care to optimizing operational efficiency.
Patient Care
One of the primary applications of healthcare data analytics is in improving patient care. By analyzing patient data, healthcare providers can identify risk factors, predict outcomes, and personalize treatment plans.
Operational Efficiency
Healthcare data analytics can also help healthcare organizations improve operational efficiency. For instance, by analyzing patient flow data, hospitals can optimize staffing levels and reduce wait times.
Research and Development
In the field of research and development, healthcare data analytics can accelerate drug discovery, enhance clinical trial design, and facilitate genomic research.
Challenges and Future Directions
Despite its potential, healthcare data analytics also faces several challenges, including data privacy concerns, data quality issues, and the need for specialized skills. Looking ahead, the field is expected to continue evolving with advancements in technologies such as artificial intelligence, machine learning, and blockchain.