Genome-wide association study
Introduction
A Genome-Wide Association Study (GWAS) is an observational study of a genome-wide set of genetic variants in different individuals to see if any variant is associated with a trait. GWASs typically focus on associations between single-nucleotide polymorphisms (SNPs) and traits like major human diseases, but can equally be applied to any other organism.
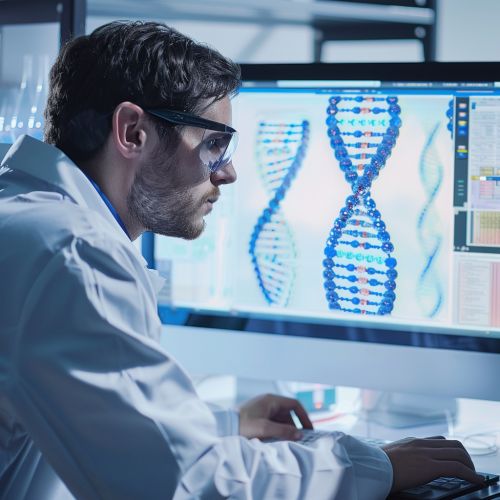
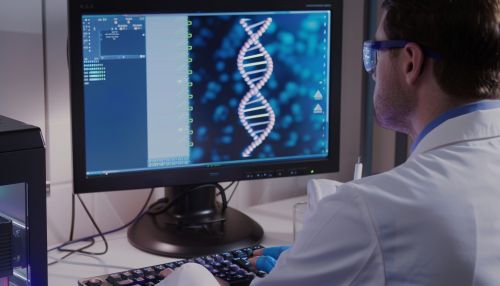
Overview
GWAS is a way to identify genes involved in human disease. This method searches the genome for small variations, called SNPs, that occur more frequently in people with a particular disease than in people without the disease. Each study can look at hundreds or thousands of SNPs at the same time. Researchers use data from this type of study to pinpoint genes that may contribute to a person's risk of developing a certain disease.
Methodology
The general methodology of a GWAS is relatively straightforward. First, choose a large enough sample size of individuals with the disease or trait of interest (cases) and a similar number of individuals without the disease or trait (controls). Then, genotype both cases and controls for many genetic markers spread throughout the genome. These markers are usually SNPs. Finally, compare the frequency of the alleles at each SNP in cases versus controls.
Data Analysis
Data analysis for GWAS involves five basic steps: quality control, population stratification, single marker analysis, gene-gene interaction analysis, and gene-environment interaction analysis. Each step is crucial in ensuring the validity and reliability of the study results.
Limitations and Criticisms
Despite the success of GWAS in identifying genetic variants associated with common diseases, the approach has received criticism. The major concerns include the lack of reproducibility, the reliance on linkage disequilibrium, the focus on common variants, and the lack of functional information.
Future Directions
The future of GWAS will likely involve larger sample sizes, more comprehensive coverage of genetic variation, and more detailed phenotyping. The incorporation of functional data and the use of next-generation sequencing are also expected to play a significant role in the future of GWAS.