Flood Forecasting
Introduction
Flood forecasting is a method used to predict flood events in advance. It is a crucial aspect of hydrology and plays a significant role in disaster management and mitigation. This process involves the use of various meteorological data, hydrological models, and computational techniques to estimate the likelihood and severity of flooding.
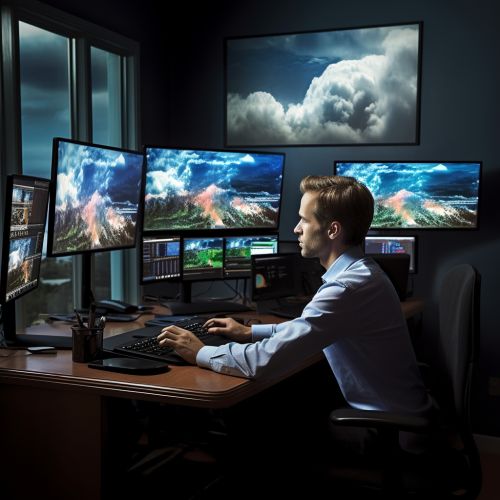
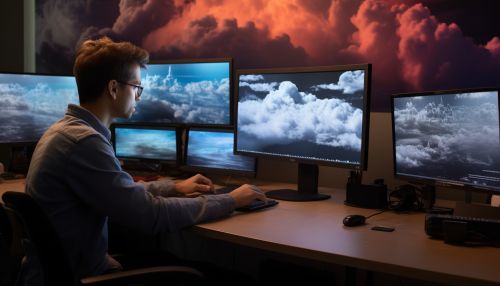
Understanding Floods
A flood is an overflow of water onto normally dry land. This natural disaster can occur due to several reasons, including heavy rainfall, snowmelt, dam failure, or coastal storms. The severity of a flood can vary from a mere inconvenience to a major disaster, causing significant damage to infrastructure, property, and loss of life.
Flood Forecasting Methods
There are several methods used in flood forecasting, each with its own strengths and weaknesses. These methods can be broadly classified into statistical methods, deterministic methods, and machine learning methods.
Statistical Methods
Statistical methods in flood forecasting use historical flood data to predict future flood events. These methods include frequency analysis, regression analysis, and time series analysis. While these methods are relatively simple to use, they often fail to account for changes in land use, climate change, and other factors that can influence flood patterns.
Deterministic Methods
Deterministic methods use physical models to simulate the hydrological cycle and predict flood events. These models include the Saint-Venant equations, which describe the motion of water in rivers and channels, and the Shallow water equations, which are used to model larger bodies of water such as lakes and oceans. These methods can provide accurate predictions, but they require a lot of computational resources and detailed data about the area being modeled.
Machine Learning Methods
Machine learning methods use algorithms to learn patterns in data and make predictions based on those patterns. These methods can handle large amounts of data and can account for complex interactions between different variables. However, they require a large amount of data to train the algorithms and can be difficult to interpret.
Flood Forecasting Systems
Flood forecasting systems are designed to provide timely and accurate forecasts of flood events. These systems typically include data collection, data processing, flood forecasting models, and dissemination of forecast information.
Data Collection
Data collection in flood forecasting involves gathering data from various sources, including weather stations, river gauges, and satellites. This data includes rainfall, temperature, wind speed and direction, river flow, and other relevant information.
Data Processing
Once the data is collected, it is processed to remove errors and inconsistencies. This process includes quality control, data interpolation, and data assimilation.
Flood Forecasting Models
The processed data is then input into a flood forecasting model, which uses mathematical equations to simulate the hydrological cycle and predict flood events.
Dissemination of Forecast Information
Once the forecast is made, it is disseminated to relevant authorities and the public. This can be done through various channels, including websites, mobile apps, and emergency alert systems.
Challenges in Flood Forecasting
Despite advances in technology, there are still several challenges in flood forecasting. These include data availability and quality, model uncertainty, and communication of forecast information.
Future of Flood Forecasting
With the advent of new technologies such as remote sensing, big data, and artificial intelligence, the future of flood forecasting looks promising. These technologies have the potential to improve the accuracy and timeliness of flood forecasts, thereby reducing the impact of floods.