Fire Dynamics Simulator
Introduction
The Fire Dynamics Simulator (FDS) is a computational fluid dynamics model developed by the NIST and the USFS. It is designed to simulate fire-driven fluid flow in a numerical manner. The simulator is widely used in the field of fire protection engineering and fire research due to its ability to accurately model the complex physical phenomena associated with fires.
Development and Features
The development of FDS began in the late 1990s with the aim of providing a tool that could simulate real-world fire scenarios with a high degree of accuracy. The simulator utilizes a large eddy simulation (LES) approach for the representation of the turbulent flow. This method is more accurate than the traditional Reynolds-averaged Navier-Stokes (RANS) approach, as it can capture the large-scale turbulent structures that are critical in fire dynamics.
FDS is capable of simulating a wide range of fire scenarios, from small-scale experimental fires to large-scale building fires. It can model the growth and spread of fire, the production and transport of smoke, and the heat transfer to the surrounding structures. The simulator also includes models for sprinkler activation, smoke detection, and occupant behavior, making it a comprehensive tool for fire safety analysis.
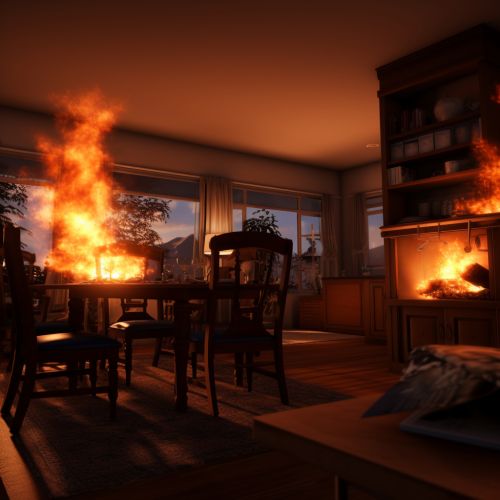
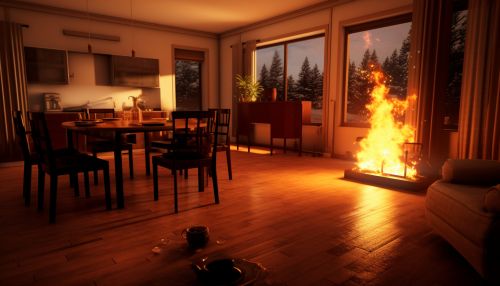
Mathematical Models
The core of FDS is based on the solution of the Navier-Stokes equations, which describe the motion of fluid. These equations are coupled with additional equations that model the heat transfer, the combustion process, and the production of smoke.
The Navier-Stokes equations are solved using a finite volume method, which divides the computational domain into a grid of cells. The fluid properties and the state of the fire are calculated at the center of each cell, and the equations are solved iteratively until a steady state is reached or the simulation time is completed.
The combustion process is modeled using a mixture fraction approach, which assumes that the fire is a mixing-controlled reaction between the fuel and the oxidizer. This approach is suitable for most fire scenarios, as it can accurately capture the rapid chemistry of the combustion process.
The production of smoke is modeled using a soot yield approach, which calculates the amount of soot produced based on the amount of fuel burned. The transport of smoke is then modeled using the same equations as the fluid flow, with additional terms to account for the buoyancy effect of the hot smoke.
Applications
FDS is used in a wide range of applications in the field of fire safety. It can be used to design and evaluate fire protection systems, such as sprinkler systems and smoke control systems. It can also be used to conduct fire investigations and to recreate fire scenarios for training purposes.
In the field of fire research, FDS is used to study the fundamental processes of fire dynamics and to develop new models for fire behavior. The simulator is also used in the development of fire safety standards and codes, as it can provide valuable data on the performance of buildings and materials under fire conditions.
Limitations and Future Development
While FDS is a powerful tool for fire safety analysis, it has certain limitations. The accuracy of the simulation results depends on the quality of the input data and the resolution of the computational grid. High-resolution simulations require significant computational resources and can take a long time to complete.
The development of FDS is ongoing, with new versions being released regularly. Future development will focus on improving the accuracy and efficiency of the models, and on expanding the range of fire scenarios that can be simulated. This includes the development of models for new types of fuels and combustion processes, and the integration of new technologies such as machine learning and high-performance computing.