Environmental Modelling
Introduction
Environmental modelling is a scientific practice that uses mathematical and computational techniques to understand and simulate the interactions between natural and human systems. It is a crucial tool in environmental science, helping to predict future scenarios, understand past changes, and inform policy decisions. Environmental modelling encompasses a wide range of disciplines, including climate, hydrology, ecology, and geology, among others.
History
The concept of environmental modelling dates back to the early 20th century, with the development of mathematical models to predict weather patterns. However, it was not until the advent of modern computing in the mid-20th century that environmental modelling truly came into its own. The increased computational power allowed scientists to develop more complex and accurate models, leading to significant advances in our understanding of the environment.
Types of Environmental Models
There are several types of environmental models, each suited to different types of problems and data. These include deterministic models, stochastic models, dynamic models, static models, and spatial models.
Deterministic Models
Deterministic models are those in which the output is fully determined by the input parameters. These models are often used in situations where the underlying processes are well understood and there is little uncertainty in the input data.
Stochastic Models
Stochastic models, on the other hand, incorporate elements of randomness. These models are used when there is significant uncertainty in the input data or the underlying processes are not fully understood.
Dynamic Models
Dynamic models are those that change over time. These models are often used to simulate processes that evolve over time, such as the growth of a population or the spread of a disease.
Static Models
Static models do not change over time. These models are often used to represent a snapshot of a system at a particular point in time.
Spatial Models
Spatial models represent the spatial distribution of a variable or process. These models are often used in fields such as geography and ecology to represent the distribution of species or resources.
Applications of Environmental Modelling
Environmental modelling is used in a wide range of applications, from predicting the impacts of climate change to managing natural resources. Some of the key applications include:
Climate Change Modelling
Climate change modelling is a key application of environmental modelling. These models simulate the Earth's climate system, including the atmosphere, oceans, land surface, and ice, to predict future climate change and its impacts.
Hydrological Modelling
Hydrological modelling is used to simulate the movement and distribution of water in the environment. These models are used in water resource management, flood forecasting, and understanding the impacts of land use changes on water resources.
Ecological Modelling
Ecological modelling is used to simulate the interactions between species and their environment. These models are used in conservation planning, understanding the impacts of habitat loss, and predicting the spread of invasive species.
Geological Modelling
Geological modelling is used to simulate the Earth's crust and the processes that shape it. These models are used in mineral exploration, understanding natural hazards, and predicting the impacts of human activities on the geosphere.
Challenges in Environmental Modelling
Despite its many applications, environmental modelling faces several challenges. These include the complexity of natural systems, the uncertainty in input data, and the need for high computational power. Furthermore, environmental models are often based on simplifications and assumptions that may not fully capture the complexity of the real world.
Future Directions
With advances in computational power and data collection, environmental modelling is set to become even more powerful and accurate. Future directions include the development of integrated models that can simulate multiple environmental processes simultaneously, the use of machine learning techniques to improve model accuracy, and the incorporation of social and economic factors into environmental models.
See Also
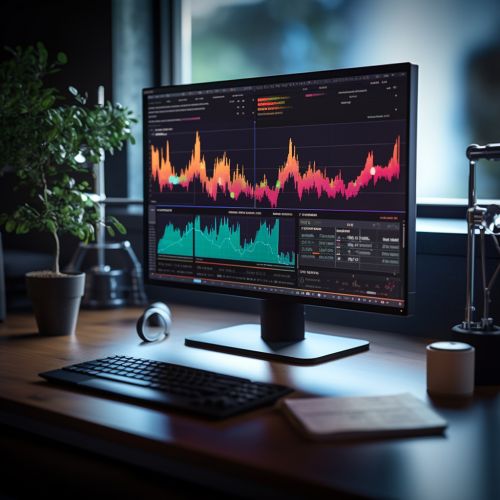
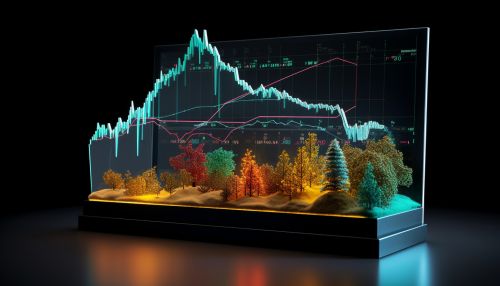