Disease Modeling
Introduction
Disease modeling is a scientific field that uses mathematical and computational techniques to understand and predict how diseases spread and progress. This discipline is crucial in epidemiology, public health, and clinical medicine. Disease models can help inform strategies for disease prevention and control, and they can also guide research into new treatments and interventions.
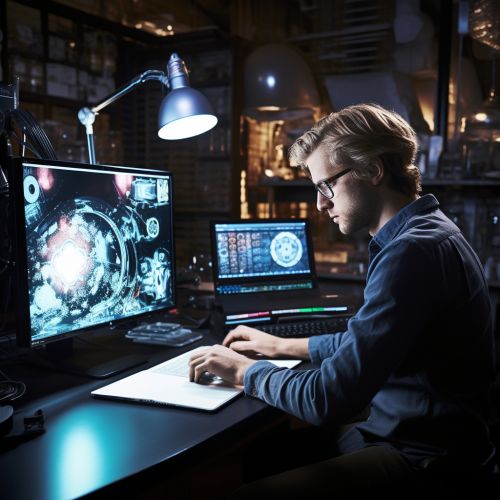
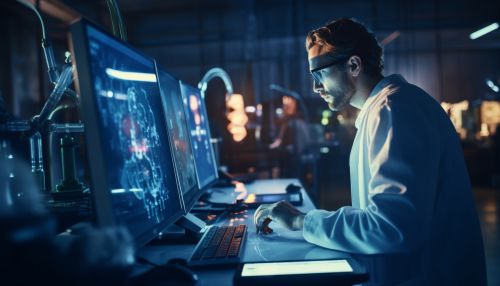
History of Disease Modeling
The history of disease modeling can be traced back to the 18th century, when mathematicians like Daniel Bernoulli started using mathematical equations to describe the spread of diseases like smallpox. In the 20th century, with the advent of computers, disease modeling became a more sophisticated and powerful tool. Today, disease modeling is a multidisciplinary field that involves mathematicians, biologists, epidemiologists, and computer scientists.
Types of Disease Models
There are several types of disease models, each with its own strengths and weaknesses. These include deterministic models, stochastic models, agent-based models, and network models.
Deterministic Models
Deterministic models are the simplest type of disease model. They use a set of equations to predict the future state of a disease based on its current state. The most famous deterministic model is the SIR model, which divides the population into three groups: susceptible, infected, and recovered.
Stochastic Models
Stochastic models are similar to deterministic models, but they incorporate randomness to account for the inherent uncertainty in disease dynamics. These models are often used when the number of infected individuals is small, or when the disease dynamics are highly unpredictable.
Agent-Based Models
Agent-based models are a type of computational model that simulates the actions and interactions of individual agents (e.g., people, cells) in a network. These models are particularly useful for studying complex diseases that involve multiple interacting factors.
Network Models
Network models are a type of disease model that represents the population as a network of individuals connected by social or physical links. These models are especially useful for studying diseases that spread through direct contact, such as sexually transmitted diseases or airborne infections.
Applications of Disease Modeling
Disease modeling has a wide range of applications, from predicting the spread of infectious diseases to understanding the progression of chronic diseases.
Infectious Disease Modeling
Infectious disease modeling is perhaps the most well-known application of disease modeling. These models can predict the spread of diseases like influenza, HIV, and COVID-19, and they can inform strategies for vaccination, quarantine, and other interventions.
Chronic Disease Modeling
Chronic disease modeling is another important application of disease modeling. These models can help researchers understand the progression of diseases like cancer, diabetes, and heart disease, and they can guide the development of new treatments and prevention strategies.
Public Health Planning
Disease models are also used in public health planning. For example, they can help policymakers determine the most effective strategies for disease prevention and control, such as vaccination campaigns or screening programs.
Drug Development
In the field of drug development, disease models can predict the effect of new drugs or interventions on disease progression. This can help researchers design more effective clinical trials and speed up the process of drug discovery.
Future Directions in Disease Modeling
The field of disease modeling is rapidly evolving, with new techniques and technologies constantly emerging. Some of the most promising future directions in disease modeling include the use of big data, machine learning, and personalized medicine.
Big Data in Disease Modeling
The advent of big data has opened up new possibilities for disease modeling. With the ability to collect and analyze large amounts of data, researchers can create more accurate and detailed models of disease spread and progression.
Machine Learning in Disease Modeling
Machine learning is another promising tool in disease modeling. By training algorithms on large datasets, researchers can create models that can predict disease outcomes with high accuracy.
Personalized Medicine and Disease Modeling
The field of personalized medicine, which aims to tailor treatments to individual patients based on their genetic and environmental factors, is also benefiting from advances in disease modeling. By creating individualized disease models, researchers can predict how a particular patient will respond to a treatment, leading to more effective and personalized care.
See Also
- Epidemiology
- Public Health
- Computational Biology
- Mathematical Biology
- Infectious Diseases
- Chronic Diseases
- Big Data
- Machine Learning
- Personalized Medicine