Data citation
Introduction
Data citation refers to the practice of providing a reference to datasets in scholarly publications, similar to how traditional citations reference books, articles, and other sources. This practice is essential for ensuring the reproducibility of scientific research, acknowledging the contributions of data creators, and facilitating the discovery and reuse of datasets.
Importance of Data Citation
Data citation is crucial for several reasons. Firstly, it ensures that datasets are properly credited, which can incentivize researchers to share their data. Secondly, it enhances the transparency and reproducibility of research by allowing others to access the original data used in a study. Thirdly, it facilitates data discovery and reuse, enabling other researchers to build upon existing datasets.
Principles of Data Citation
The principles of data citation are designed to ensure that datasets are cited in a consistent and informative manner. These principles include:
- **Credit and Attribution**: Properly crediting the creators of the dataset.
- **Unique Identification**: Using persistent identifiers such as DOIs (Digital Object Identifiers) to uniquely identify datasets.
- **Access**: Ensuring that the dataset is accessible to others.
- **Specificity**: Providing enough detail to identify the specific dataset used.
- **Interoperability**: Using standard formats and practices to enable data sharing and reuse.
Components of a Data Citation
A complete data citation typically includes the following components:
- **Author(s)**: The individuals or organizations responsible for creating the dataset.
- **Title**: The title of the dataset.
- **Year of Publication**: The year the dataset was made available.
- **Version**: The specific version of the dataset, if applicable.
- **Publisher**: The entity that distributes the dataset.
- **Identifier**: A persistent identifier, such as a DOI, that uniquely identifies the dataset.
Standards and Guidelines
Several organizations have developed standards and guidelines for data citation. These include:
- **DataCite**: An organization that provides DOIs for datasets and promotes best practices for data citation.
- **CODATA**: The Committee on Data of the International Science Council, which has developed principles for data citation.
- **Force11**: A community of scholars, librarians, and publishers that has developed the Joint Declaration of Data Citation Principles.
Challenges in Data Citation
Despite its importance, data citation faces several challenges:
- **Lack of Awareness**: Many researchers are not aware of the importance of data citation or how to properly cite datasets.
- **Technical Barriers**: Ensuring that datasets are accessible and properly identified can be technically challenging.
- **Cultural Barriers**: In some fields, there is a lack of a culture of data sharing and citation.
Best Practices for Data Citation
To overcome these challenges, researchers and institutions can adopt several best practices:
- **Education and Training**: Providing education and training on the importance of data citation and how to properly cite datasets.
- **Infrastructure**: Developing infrastructure to support data citation, such as repositories that provide persistent identifiers.
- **Policies and Incentives**: Implementing policies and incentives to encourage data sharing and citation.
Case Studies
Several case studies highlight the importance and impact of data citation:
- **GenBank**: A database of genetic sequences that requires users to cite the database when using its data.
- **ICPSR**: The Inter-university Consortium for Political and Social Research, which provides DOIs for datasets and tracks citations.
Future Directions
The field of data citation is rapidly evolving, and several future directions are emerging:
- **Integration with Research Workflows**: Integrating data citation into research workflows to make it easier for researchers to cite datasets.
- **Improved Metrics**: Developing better metrics to track the impact of datasets and data citation.
- **Interdisciplinary Collaboration**: Promoting interdisciplinary collaboration to develop common standards and practices for data citation.
See Also
References
- DataCite. (n.d.). DataCite Metadata Schema Documentation for the Publication and Citation of Research Data. DataCite.
- CODATA-ICSTI Task Group on Data Citation Standards and Practices. (2013). Out of Cite, Out of Mind: The Current State of Practice, Policy, and Technology for the Citation of Data. Data Science Journal.
- Force11. (2014). Joint Declaration of Data Citation Principles.
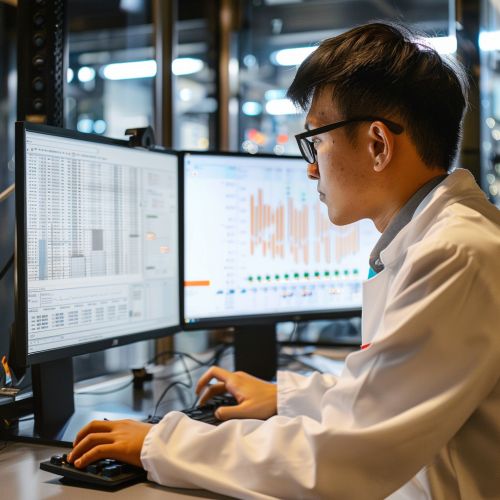
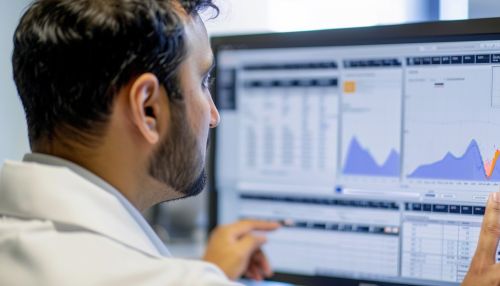