Correlation Matrix
Introduction
A Correlation Matrix is a table that displays the correlation coefficients between many variables. Each cell in the table shows the correlation between two variables. A correlation matrix is used to summarize data, as an input into a more advanced analysis, and as a diagnostic for advanced analyses.
Definition
In Statistics, a correlation matrix is a table showing correlation coefficients between sets of variables. Each random variable (Xi) in the table is correlated with each of the other values in the table (Xj). This allows the identification of collinear relationships in the data. Collinearity is a linear association between two explanatory variables. Two variables are perfectly collinear if there is an exact linear relationship between the two.
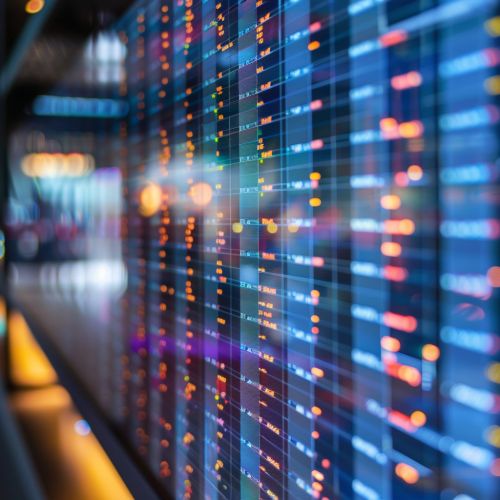
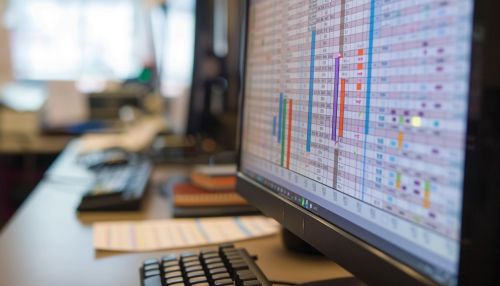
Correlation Coefficient
The Correlation Coefficient is a measure that determines the degree to which two variables' movements are associated. The range of values for the correlation coefficient bounded by 1 on an absolute value basis or between -1 to 1. If the correlation coefficient is greater than zero, it is known as a positive correlation. If the value is less than zero, it is known as a negative correlation.
Types of Correlation Matrix
There are three types of correlation matrix: Pearson product-moment correlation, Kendall rank correlation, and Spearman rank correlation.
Pearson Product-Moment Correlation
Pearson Product-Moment Correlation is a measure of the linear correlation between two variables X and Y. It has a value between +1 and −1. A value of +1 is total positive linear correlation, 0 is no linear correlation, and −1 is total negative linear correlation.
Kendall Rank Correlation
Kendall Rank Correlation is a non-parametric test that measures the strength of dependence between two variables. If we consider two samples, a and b, where each sample size is n, we know that the total number of pairings with a b is n(n-1)/2.
Spearman Rank Correlation
Spearman Rank Correlation is a non-parametric test that is used to measure the degree of association between two variables. The Spearman rank correlation test does not carry any assumptions about the distribution of the data and is the appropriate correlation analysis when the variables are measured on a scale that is at least ordinal.
Use of Correlation Matrix
Correlation matrices are used to measure how closely related are two datasets. While correlation does not imply causation, it can hint at a relationship between two variables. Correlation matrices are widely used in multiple fields such as:
- Finance: In finance, the correlation matrix is used to understand the correlation between different types of investments. This understanding can be used to diversify the investments and minimize the risk.
- Machine Learning: In machine learning, a correlation matrix is used to understand the correlation between different features of the dataset. This understanding can be used to select the most relevant features for training the model.
- Social Sciences: In social sciences, a correlation matrix is used to understand the correlation between different variables. This understanding can be used to identify the key variables that influence a particular social phenomenon.
Conclusion
The correlation matrix is a powerful tool in statistical analysis, allowing researchers to identify potential relationships between variables. However, it is important to remember that correlation does not imply causation, and further research is often necessary to understand the underlying mechanisms of these relationships.